Best MLOps Course Training in Nigeria
- 40 Hours Interactive Online Sessions
- 80 Hours Assignments
- Complementary Kubernetes for Beginners
- Complimentary DevOps for Beginners
- Complementary Python Programming

2064 Learners
Academic Partners & International Accreditations
Tab Four Description
Machine Learning - this is the buzzword that has everyone talking! Over the past few years, there has been a steady transition of Machine Learning from being strictly an academic discipline to a very exciting technological domain. The use cases are innumerable from analyzing videos from an autonomous vehicle (AV)s to providing highly personalized medical care, Machine Learning has become ubiquitous in every industry. However, most companies still have not been able to standardize the Machine Learning systems to become fully automated in a way that produces the models and results automatically. This has led to the birth of a new kind of discipline - Machine Learning Operations or MLOps for short. This field is still emerging but as companies look to leverage Machine Learning and Deep Learning to improve their business processes, MLOps Engineers will become one of the most sought after roles. It is estimated that 85% of most Machine Learning projects fail because among other things there is no standardized way of deploying these models to ‘production’. With this course, we aim to bridge the gap between and train MLOps Engineers that can deploy any model to production efficiently and quickly.
MLOps Course

Total Duration
40 Hours

Prerequisites
- Computer Skills
- Basic Mathematical Concepts
- Analytical Mindset
MLOps Course Overview in Nigeria
The MLOps Engineering course is a first of its kind program which tackles the subject of deploying the Machine Learning models in production and at scale. This program is born out of a frustration that we experienced while working on consulting engagements and trying to deploy Machine Learning projects into production. The challenges that any ML project faces is to ‘operationalize’ and ‘productionalize’ the code. There is no platform or guidelines that usually exist in other software engineering projects which makes it very difficult to deploy ML models quickly and efficiently. As part of this course, you will learn to deploy models into production environments using cutting edges open-source frameworks like Tensorflow Extended, Apache Beam, Apache Airflow, Kubernetes, and Kubeflow.
MLOps Course Learning Outcomes in Nigeria
This course has been meticulously and laboriously designed to be one of the pioneering works in the field of MLOps. While there is both a lot of demand and supply of Data Scientists, the market is experiencing a crushing shortage of MLOps engineers who can then convert the models into products and services that can be automatically deployed. This course is one of the first to offer MLOps training and will help the learners land coveted jobs as ML Engineers. ML projects have a lot of hidden technical debt as referenced in this wonderful paper. Unfortunately, the ML code will only be a very insignificant part of the entire codebase required to put an ML project into operation as shown in the below picture. So, this course addresses how an ML project can be quickly deployed into production with highly reusable pipelines.
Block Your Time
Who Should Sign Up?
- Data Scientists
- Data and Analytics Manager
- Business Analysts
- Data Engineers
- DevOps Engineers
- IT/Software Engineers
- Machine Learning Architects
- Model Risk Managers/Auditors
Modules for MLOps Course
The following modules will take the student through the course in a step by step fashion building upon the foundations and progressing to advanced topics. Initially, the first module introduces the students to the general ML workflow and the different phases in an ML lifecycle. The subsequent chapters will introduce the participant to Tensorflow Extended (TFX) followed by a deep dive into its various components and how they facilitate the different phases of the ML lifecycle. The learner will then gain an understanding of how TFX components are used for data ingestion, validation, preprocessing, model training and tuning, evaluation, and finally deployment. Later chapters will also introduce the learner to the orchestration software Kubeflow, Apache Airflow, and Apache Beam. Using a combination of all these tools, the learner will be able to deploy models in some popular cloud platforms like AWS, GCP, and Azure.
One of the key benefits of investing in machine learning pipelines is that all the steps of the data science life cycle can be automated. As and when new data is available (for training), ideally an automated workflow should be triggered which performs data validation, preprocessing, model training, analysis, and deployment. A lot of data science teams spend ridiculous amounts of time, money and resources doing all these tasks manually. By investing in an ML workflow, these issues could be resolved. Some of the benefits include (but are not limited to):
- Create new models, don’t get stuck maintaining Existing Models
- Preventing and Debugging Errors
- Audit Trail
- Standardization
The Tensorflow Extended (TFX) library contains all the components that are needed to build robust ML pipelines. Once the ML pipeline tasks are defined using TFX, they can then be sequentially executed with an orchestration framework such as Airflow or Kubeflow Pipelines.
During this module, you will learn to install TFX and its fundamental concepts along with some literature which will make the future modules easier to understand. Additionally, you will learn Apache Beam which is an open-source tool that helps in defining and executing some data manipulation tasks. There are two basic purposes of Apache Beam in the TFX pipelines:
- It forms the base of several TFX components for data preparation/preprocessing and validation
- Is one of the orchestration frameworks for TFX components so a good understanding of Apache Beam is necessary if you wish to write custom components
In the previous modules, we set up TFX the ML MetadataStore. In this module, we discuss how to ingest data into a pipeline for consumption in various TFX components (like ExampleGen). There are several TFX components that allow us to ingest data from files or services. In this module we discuss the fundamental concepts, explore how to split the datasets into train and eval files and practically understand how to join multiple data sources into one all-encompassing dataset. We will also understand what a TFRecord stands for and how to interact with external services such as Google Cloud BigQuery. You will also learn how TFRecord can work with CSV, Apache Avro, Apache Parquet etc. This module will also introduce some strategies to ingest different forms of data structured, text, and images. In particular, you will learn
- Ingesting local data files
- Ingesting remote data files
- Ingesting directly from databases (Google BigQuery, Presto)
- Splitting the data into train and eval files
- Spanning the datasets
- Versioning
- Working with unstructured data (image, text etc)
Data validation and preprocessing is essential for any machine learning algorithm to perform well. The old adage ‘garbage-in, garbage out’ perfectly encapsulates this fundamental characteristic of any ML model. As such, this module will focus on validation and preprocessing of data to ensure the creation of high performing ML models.
Data Validation: This module will introduce you to a Python package called Tensorflow Data Validation (TFDV) which will help in ensuring that
- The data in the pipeline is in line with what the feature engineering step expects
- Assists in comparing multiple datasets
- Identifies if the data changes over time
- Identify the schema of the underlying data
- Identify data skew and data shift
Real-world data is extremely noisy and not in the same format that can be used to train our machine learning models. Consider a feature which has values as Yes and No tags which need to be converted to a numerical representation of these values (e.g., 1and 0) to allow for consumption by an ML model. This module focuses on how to convert features into numerical representations so that your machine learning model can be trained.
We introduce Tensorflow Transform (TFT) which is the TFX component specifically built for data preprocessing allowing us to set up preprocessing steps as TensorFlow graphs. Although this step of the model has a considerable learning curve it is important to know about it for the following reasons:
- Efficiently preprocessing the data within the context of the entirety of the dataset
- The ability to scale the data preprocessing steps efficiently
- Develop immunity to potentially encountering training-serving skew
As part of the previous modules, we completed data preprocessing and transforming the data to fit our model formats. The next logical step in the pipeline is to begin the model training, perform analysis on the trained models and evaluate and select the final model. This module already assumes that you have the knowledge of training and evaluating models so we don’t dwell fully in the different model architectures. We will learn about the TFX Trainer component which helps us in training a model which can easily be put into production. Additionally, you will also be introduced to Tensorboard which can be used to monitor training metrics, visualize word embeddings in NLP problems or view activations for layers in a deep learning model.
During the model training phase, we typically monitor its performance on an evaluation set and use Hyperparameter optimization to improve performance. As we are building an ML pipeline, we need to remember that the purpose is to answer a complex business question modelling a complex real-world system. Oftentimes our data deals with people, so a decision that is made by the ML model could have far-reaching effects for real people and sometimes even put them in danger. Hence it is critical that we monitor your metrics through time—before deployment, after deployment, and while in production. Sometimes it may be easy to think that since the model is static it does not need to be monitored constantly, but in reality, the incoming data into the pipeline will more likely than not change with time, leading to performance degradation.
TFX has produced the Tensorflow Model Analysis (TFMA) module which is a fantastic and super-easy way to obtain exhaustive evaluation metrics such as accuracy, precision, recall, AUC metrics and f1-score, RMSE, MAPE, MAE among others. Using TFMA, the metrics can be visually depicted in the form of a time series spanning all the different model versions and as an add-on, it gives the ability to view metrics on different splits of the dataset. Another important feature is that by using this module it is easy to scale to large evaluation sets via Apache Beam. Additionally in this module, you will learn
- How to analyse a single model using TFMA
- How to analyse multiple models using TFMA
- Checking for fairness among models
- Apply decision thresholds with fairness indicators
- Tackling model explainability
- Using the TFX components Resolver, Evaluator and Pusher to analyze models automatically
This module is in many ways the crux of the MLOps domain because the original question was - ‘I have a great ML model prototype, how do I deploy it to production?’. With this module, we answer that question with - here is how: using Tensorflow Serving which allows ML engineers and data engineers to deploy any TensorFlow graph allowing them to generate predictions from the graph through its standardized endpoints. TF Serving takes care of the model and version control allowing for models to be served based on policies and the ability to load models from various sources. All of this is accomplished by focussing on high-performance throughput to achieve low-latency predictions. Some of the topics discussed in this module are:
- How to export models for TF (TensorFlow) Serving
- Signatures of Models
- How to inspect exported models
- Set up of TF Serving
- How to configure a TF Server
- gRPC vs REST API architecture
- How to make predictions from a model server using
- gRPC
- REST
- Conduct A/B testing using TF Serving
- Seeking model metadata from the model server using
- gRPC
- REST
- How to configure batch inference requests
Pipeline orchestration tool is crucial to ensure that we are abstracted from having to write some glue code to automate an ML pipeline. Pipeline orchestrators usually lie under the components introduced in the previous modules.
- Decide upon the orchestration tool - Apache Beam vs Apache Airflow vs Kubeflow
- Overview of Kubleflow pipelines on AI Platform
- How to push your TFX Pipeline into production
- Pipeline conversion for Apache Beam and Apache Airflow
- How to set up and orchestrate TFX pipelines using
- Apache Beam
- Apache Airflow
- Kubeflow
Tools Covered
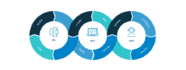
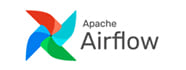
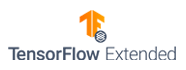
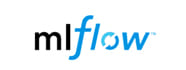
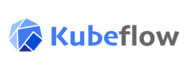
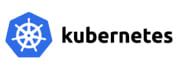
MLOps Course Trends
Data Science technologies paramount in the effort to collect, prepare, predict, and respond to the proactive and accelerated growth of data. The trends that will dominate the data and analytics market to prepare for a reset will include smarter and faster integration of AI technologies from piloting to operationalizing phase. It is predicted that 77% of enterprises will engage in more responsible AI that will contribute to an epic increase in streaming data and analytics infrastructures. The other trend to look out is Augmented data management, uses AI and ML technologies to optimize and improve operations, configuration, security, and performance. It also converts metadata to powering dynamic systems and facilitates automation in redundant data management tasks.
By the year 2022, 85% of data and analytics innovation will exploit cloud capabilities to improve the workload’s performance and for cost optimization. Next comes in a technology that provides transparency for complex networks of participants and provides the full lineage of assets and transactions and that is the Blockchain Technology. The other new trend is the graph technologies and algorithms that will be used to comb through thousands of data documents to uncover hidden patterns and relationships. The application of Graph Analytics ranges from discovering new possible treatments for diseases that often have negative outcomes for patients, traffic route optimization n, fraud detection, and social network analysis to genome research. Nothing else adds a bigger opportunity to the employability of professionals for the Data Science industry that needs 6 million workers every year. Get ready to enroll for the practical Data Science training in the Nigeria if you want to power your dreams ahead.
How We Prepare You
-
Additional Assignments of over 80+ hours
-
Live Free Webinars
-
Resume and LinkedIn Review Sessions
-
Lifetime LMS Access
-
24/7 Support
-
100% Practical Oriented Course
-
Complimentary Courses
-
Unlimited Mock Interview and Quiz Session
-
Hands-on Experience in Capstone Projects
-
Life Time Free Access to Industry Webinars
Call us Today!
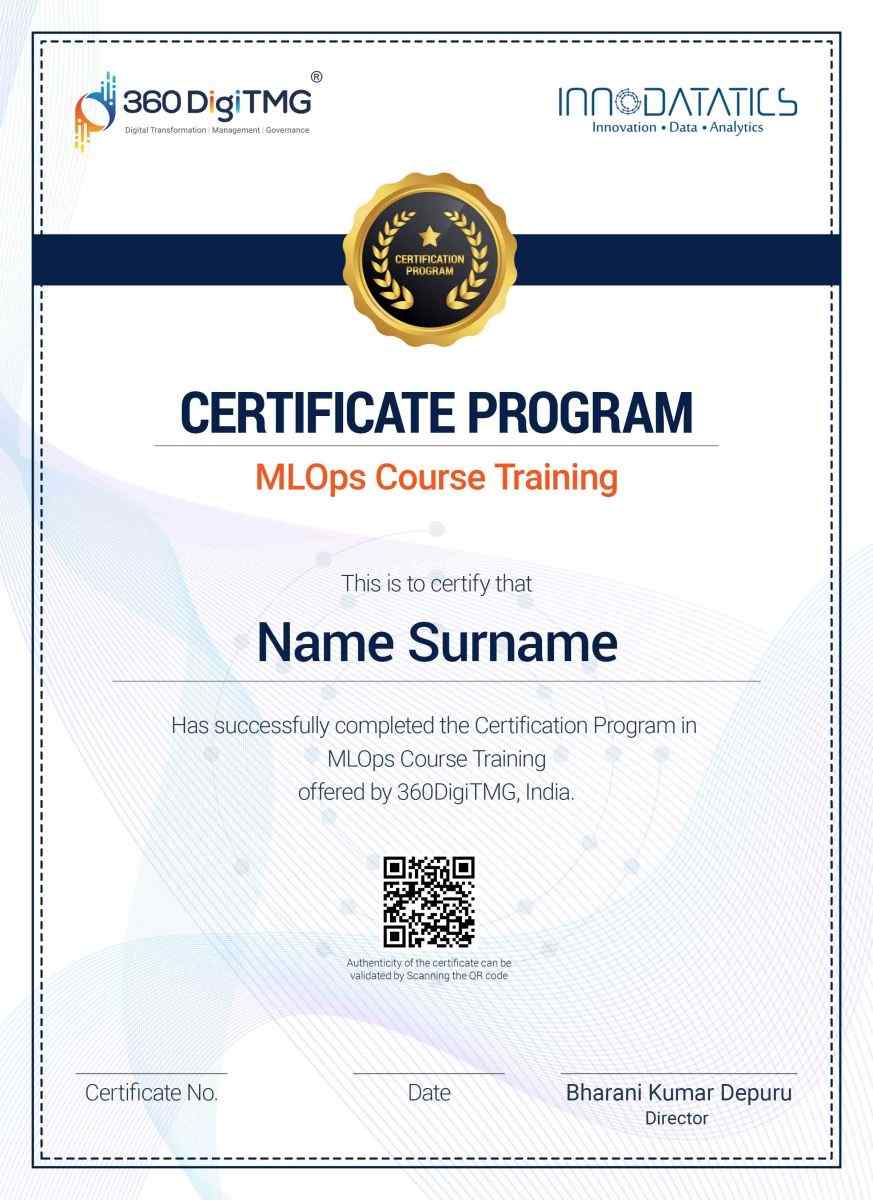
Certificate
Earn a certificate and demonstrate your commitment to the profession. Use it to distinguish yourself in the job market, get recognised at the workplace and boost your confidence. The MLOps Engineer Course Certificate is your passport to an accelerated career path.
Recommended Programmes
Foundation Program In Data Science

2064 Learners
Certification Program In Big Data

3021 Learners
Certificate Course In AI & Deep Learning

2915 Learners
Alumni Speak
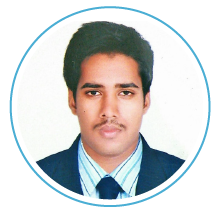
"The training was organised properly, and our instructor was extremely conceptually sound. I enjoyed the interview preparation, and 360DigiTMG is to credit for my successful placement.”
Pavan Satya
Senior Software Engineer

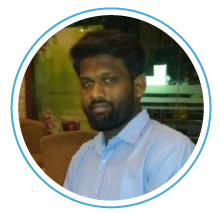
"Although data sciences is a complex field, the course made it seem quite straightforward to me. This course's readings and tests were fantastic. This teacher was really beneficial. This university offers a wealth of information."
Chetan Reddy
Data Scientist

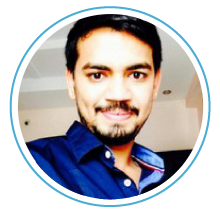
"The course's material and infrastructure are reliable. The majority of the time, they keep an eye on us. They actually assisted me in getting a job. I appreciated their help with placement. Excellent institution.”
Santosh Kumar
Business Intelligence Analyst

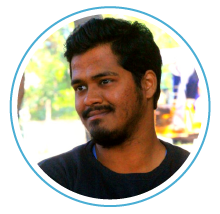
"Numerous advantages of the course. Thank you especially to my mentors. It feels wonderful to finally get to work.”
Kadar Nagole
Data Scientist

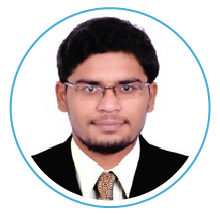
"Excellent team and a good atmosphere. They truly did lead the way for me right away. My mentors are wonderful. The training materials are top-notch.”
Gowtham R
Data Engineer

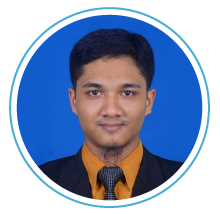
"The instructors improved the sessions' interactivity and communicated well. The course has been fantastic.”
Wan Muhamad Taufik
Associate Data Scientist

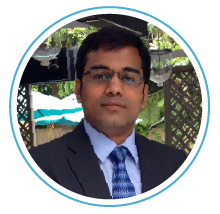
"The instructors went above and beyond to allay our fears. They assigned us an enormous amount of work, including one very difficult live project. great location for studying.”
Venu Panjarla
AVP Technology

Our Alumni Work At
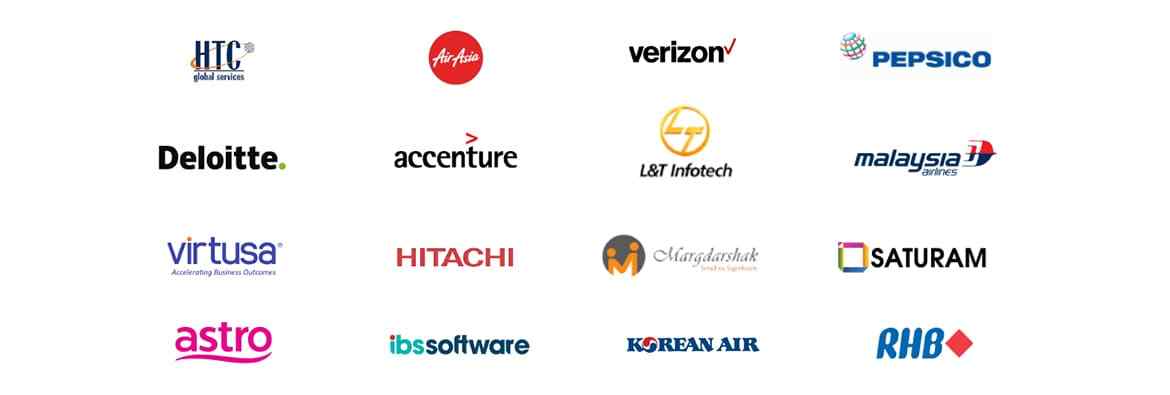
And more...
FAQs for MLOps Course
Basic Degree is required. Basic knowledge of Maths and Statistics is needed to learn the tools.
More than 20 assignments are provided for the students to make them proficient. A dedicated team of mentors will guide the students throughout their learning process.
MLOps is needed to enhance the machine learning-driven applications in business. This enables Data Scientists to concentrate on their work and empowers MLOps engineers to take responsibility and handle machine learning in production.
Machine Learning operations are considered to be the most valuable practices any company can have. It helps in improving quality and delivering better performance.
We provide a career coach to help you to build your portfolio and prepare you for facing interviews. 100% job placement is guaranteed.
Yes, you can attend a free demo class and can interact with the trainer to clarify your queries.
Yes, students will be given more than 3 real-time projects under the guidance of industry experts.
Students after completing the course will be prepared for interviews. Guidance will be given by conducting mock interviews and questionnaires. This session will help students to boost their confidence and improve their communication skills.
We provide online training with flexible timings.
You can clarify your doubts with trainers, and mentors are provided for the students to whom you can approach at any time.
You will be given LMS access, which helps you to revise the course and if you miss any class you can see the recorded version of the class. You can attend webinars for free that will be conducted on trending topics for a lifetime and many more.
The average salary for a Machine Learning Engineer in Nigeria is up to $111,165 in early career and at midlevel, the salary would amount to $135,506 per annum.
For an experienced Machine Learning Engineer in Nigeria, the average salary is $147,575. The salary changes with experience and skills.
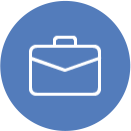
Jobs in the Field of MLOps Course in Nigeria.
The popular job profiles for MLOps include Data Scientist, Machine Learning Engineer, Business Analyst, Principal Design Manager, Machine Learning Scale specialist, and henceforth.
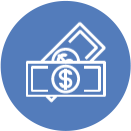
Salaries in Nigeria for the MLOps Course
The average salary for a Machine Learning Engineer in the Nigeria is up to $111,165 in early career and at mid level, the salary would amount to $135,506 per annum. For an experienced Machine Learning Engineer in the Nigeria, the average salary is $147,575. The salary changes with experience and skills.
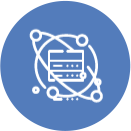
MLOps Course Projects in Nigeria
ML is emerging and making better decisions in business-critical use cases, from sales to Business intelligence, Marketing, R&D, Executive, Production and Management.
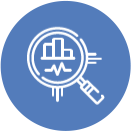
Role of Open Source Tools in MLOps Course
The most important and major tools of MLOps are DVC( Data Version Control), Pachyderm, and Kubeflow. Students are required to be proficient in these tools.
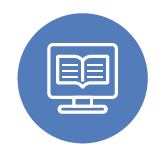
Modes of Training for MLOps Course
Training is provided through interactive live online sessions. Individual attention and personal mentorship are also provided for the students throughout their learning journey.
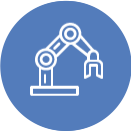
Industry Applications of MLOps Course
MLOps is driving rapidly in many sectors that include IT, Finance, Telecommunications, Manufacturing, Retail, Education, Health care, and so on.
Companies That Trust Us
360DigiTMG offers customised corporate training programmes that suit the industry-specific needs of each company. Engage with us to design continuous learning programmes and skill development roadmaps for your employees. Together, let’s create a future-ready workforce that will enhance the competitiveness of your business.
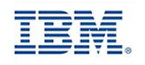
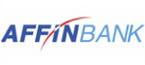
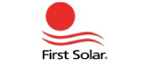
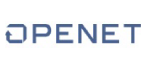
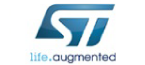
Student Voices