Artificial Intelligence Course Training in Gurgaon
- 80 Hours of Intensive Classroom & Online Sessions
- 100+ Hours of Practical Assignments
- 2 Capstone Live Projects
- 100% Job Placement Assurance

2915 Learners
Academic Partners & International Accreditations
Calendar-On-Campus Classes
Course Fee
Artificial Intelligence Training in Gurgaon
The Artificial Intelligence training in Gurgaon introduces you to the concept of AI which is the process of teaching machines to mimic the way humans learn. Automate your key business processes with AI through the certification program on AI and Deep Learning in Gurgaon. Build Artificial Intelligence systems using Deep Learning and Machine Learning algorithms with the assistance of this Artificial Intelligence Course. Develop AI and Deep Learning solutions using Python libraries. Implement Deep Learning solutions with CNN's and enable Natural Language Processing (NLP) with RNN's. Apply GPUs and TPUs in Deep Learning algorithms. Master the concepts of Artificial Intelligence at 360DigiTMG - the best Artificial Intelligence Course in Gurgaon.
This Artificial Intelligence Course has been conceived and structured to groom consummate AI professionals. In the initial modules, training is imparted on building AI systems using Deep Learning algorithms. The student learns to run all variants of Neural Network Machine Learning Algorithms. This course enables the students to implement Deep Learning solutions with Convolution Neural Networks and perform Text Analytics and Natural Language Processing (NLP) using Recurrent Neural Networks. The usage of Python libraries, GPUs, and TPUs in solving Deep Learning problems are highlighted in the best Artificial Intelligence Course in Gurgaon.
What is Artificial Intelligence?
AI is making intelligent computer programs that make machines intelligent so they can act, plan, think, move, and manipulate objects like humans. Due to massive increases in data collection and new algorithms, AI has made rapid advancement in the last decade. It is going to create newer and better jobs and liberate people from repetitive mental and physical tasks. Companies are using image recognition, machine learning, and deep learning in the fields of advertising, security, and automobiles to better serve customers. Digital assistants like Alexa or Siri are giving smarter answers to questions and performing various tasks and services with just a voice command.
What is Deep Learning?
Deep Learning is often referred to as a subfield of machine learning, where computers are taught to learn by example just like humans, sometimes exceeding human-level performance. In deep learning, we train a computer model by feeding in large sets of labeled data and providing a neural network architecture with many layers. In the course of this program, you will also learn how deep learning has become so popular because of its supremacy in terms of accuracy when trained with massive amounts of data.
AI Training Outcomes in Gurgaon
This AI Training in Gurgaon introduces you to the concept of Artificial Intelligence and Deep learning and helps you in understanding Neural Network Architectures, Supervised and Unsupervised Learning, Decision Tree Learning, and Structuring of Algorithms for new AI machines along with learning to minimize errors through advanced optimization techniques. Artificial intelligence is always compared to human intelligence when it comes to solving complex human-centered problems. It has been examining and studying how to incorporate intelligent human behaviors in a computer.It has been researching on topics related to reasoning, problem solving, machine learning, automatic planning and so on.This course provides a challenging avenue for exploring the basic principles, techniques, strengths, and limitations of the various applications of Artificial Intelligence. Students will also gain an understanding of the current scope, limitations, and societal implications of artificial intelligence globally. They will investigate the various AI structures and techniques used for problem-solving, inference, perception, knowledge representation, and learning. This training will also equip them to design AI functions and components for computer games and analyze the algorithms and techniques used for searching, reasoning, machine learning, and language processing. Also, gain expertise in applying the various scientific methods to models of machine learning.
You will also
AI Course Modules in Gurgaon
This course will be the first stepping stone towards Artificial Intelligence and Deep Learning. In this module, you will be introduced to the analytics programming languages. R is a statistical programming language and Python is a general-purpose programming language. These are the most popular tools currently being employed to churn data for deriving meaningful insights. You will have a high-level understanding of the human brain, the importance of multiple layers in the Neural Network, extraction of features layers wise, composition of the data in Deep Learning using an image, speech, and text. Students will be exposed to GPUs and TPUs that will be used on cloud platforms such as Google Colab to run Google AI algorithms alongside running Neural Network algorithms on-premise GPU machines.
The Perceptron Algorithm is defined based on a biological brain model. You will talk about the parameters used in the perceptron algorithm which is the foundation of developing much complex neural network models for AI applications. Understand the application of perceptron algorithms to classify binary data in a linearly separable scenario.
- Neurons of a Biological Brain
- Artificial Neuron
- Perceptron
- Perceptron Algorithm
- Use case to classify a linearly separable data
- Multilayer Perceptron to handle non-linear data
Neural Network is a black box technique used for deep learning models. Learn the logic of training and weights calculations using various parameters and their tuning. Understand the activation function and integration functions used in developing a Artificial Neural Network.
- Integration functions
- Activation functions
- Weights
- Bias
- Learning Rate (eta) - Shrinking Learning Rate, Decay Parameters
- Error functions - Entropy, Binary Cross Entropy, Categorical Cross Entropy, KL Divergence, etc.
- Artificial Neural Networks
- ANN Structure
- Error Surface
- Gradient Descent Algorithm
- Backward Propagation
- Network Topology
- Principles of Gradient Descent (Manual Calculation)
- Learning Rate (eta)
- Batch Gradient Descent
- Stochastic Gradient Descent
- Minibatch Stochastic Gradient Descent
- Optimization Methods: Adagrad, Adadelta, RMSprop, Adam
- Convolution Neural Network (CNN)
- ImageNet Challenge – Winning Architectures
- Parameter Explosion with MLPs
- Convolution Networks
- Recurrent Neural Network
- Language Models
- Traditional Language Model
- Disadvantages of MLP
- Back Propagation Through Time
- Long Short-Term Memory (LSTM)
- Gated Recurrent Network (GRU)
Learn about single-layered Perceptrons, Rosenblatt’s perceptron for weights and bias updation. You will understand the importance of learning rate and error. Walk through a toy example to understand the perceptron algorithm. Learn about the quadratic and spherical summation functions. Weights updating methods - Windrow-Hoff Learning Rule & Rosenblatt’s Perceptron.
- Introduction to Perceptron
- Introduction to Multi-Layered Perceptron (MLP)
- Activation functions – Identity Function, Step Function, Ramp Function, Sigmoid Function, Tanh Function, ReLU, ELU, Leaky ReLU & Maxout
- Back Propagation Visual Demonstration
- Network Topology – Key characteristics and Number of layers
- Weights Calculation in Back Propagation
Understand the difference between perception and MLP or ANN. Learn about error surface, challenges related to gradient descent and the practical issues related to deep learning. You will learn the implementation of MLP on MNIST dataset - multi class problem, IMDB dataset - binary classification problem, Reuters dataset - single labelled multi class classification problem and Boston Housing dataset - Regression Problem using Python and Keras.
- Error Surface – Learning Rate & Random Weight Initialization
- Local Minima issues in Gradient Descent Learning
- Is DL a Holy Grail? Pros and Cons
- Practical Implementation of MLP/ANN in Python using Real Life Use Cases
- Segregation of Dataset - Train, Test & Validation
- Data Representation in Graphs using Matplotlib
- Deep Learning Challenges – Gradient Primer, Activation Function, Error Function, Vanishing Gradient, Error Surface challenges, Learning Rate challenges, Decay Parameter, Gradient Descent Algorithmic Approaches, Momentum, Nestrov Momentum, Adam, Adagrad, Adadelta & RMSprop
- Deep Learning Practical Issues – Avoid Overfitting, DropOut, DropConnect, Noise, Data Augmentation, Parameter Choices, Weights Initialization (Xavier, etc.)
Convolution Neural Network are the class of Deep Learning networks which are mostly applied on images. You will learn about ImageNet challenge, overview on ImageNet winning architectures, applications of CNN, problems of MLP with huge dataset.
You will understand convolution of filter on images, basic structure on convent, details about Convolution layer, Pooling layer, Fully Connected layer, Case study of AlexNet and few of the practical issues of CNN.
- ImageNet Challenge – Winning Architectures, Difficult Vision Problems & Hierarchical Approach
- Parameter Explosion with MLPs
- Convolution Networks - 1D ConvNet, 2D ConvNet, Transposed Convolution
- Convolution Layers with Filters and Visualizing Convolution Layers
- Pooling Layer, Padding, Stride
- Transfer Learning - VGG16, VGG19, Resnet, GoogleNet, LeNet, etc.
- Practical Issues – Weight decay, Drop Connect, Data Manipulation Techniques & Batch Normalization
You will learn image processing techniques, noise reduction using moving average methods, different types of filters - smoothing the image by averaging, Gaussian filter and the disadvantages of correlation filters. You will learn about different types of filters, boundary effects, template matching, rate of change in the intensity detection, different types of noise, image sampling and interpolation techniques.
You will also learn about colors and intensity, affine transformation, projective transformation, embossing, erosion & dilation, vignette, histogram equalization, HAAR cascade for object detection, SIFT, SURF, FAST, BRIEF and seam carving.
- Introduction to Vision
- Importance of Image Processing
- Image Processing Challenges – Interclass Variation, ViewPoint Variation, Illumination, Background Clutter, Occlusion & Number of Large Categories
- Introduction to Image – Image Transformation, Image Processing Operations & Simple Point Operations
- Noise Reduction – Moving Average & 2D Moving Average
- Image Filtering – Linear & Gaussian Filtering
- Disadvantage of Correlation Filter
- Introduction to Convolution
- Boundary Effects – Zero, Wrap, Clamp & Mirror
- Image Sharpening
- Template Matching
- Edge Detection – Image filtering, Origin of Edges, Edges in images as Functions, Sobel Edge Detector
- Effect of Noise
- Laplacian Filter
- Smoothing with Gaussian
- LOG Filter – Blob Detection
- Noise – Reduction using Salt & Pepper Noise using Gaussian Filter
- Nonlinear Filters
- Bilateral Filters
- Canny Edge Detector - Non Maximum Suppression, Hysteresis Thresholding
- Image Sampling & Interpolation – Image Sub Sampling, Image Aliasing, Nyquist Limit, Wagon Wheel Effect, Down Sampling with Gaussian Filter, Image Pyramid, Image Up Sampling
- Image Interpolation – Nearest Neighbour Interpolation, Linear Interpolation, Bilinear Interpolation & Cubic Interpolation
- Introduction to the dnn module
- Deep Learning Deployment Toolkit
- Use of DLDT with OpenCV4.0
- OpenVINO Toolkit
- Introduction
- Model Optimization of pre-trained models
- Inference Engine and Deployment process
Understand the language models for next word prediction, spell check, mobile auto-correct, speech recognition, and machine translation. You will learn the disadvantages of traditional models and MLP. Deep understanding of the architecture of RNN, RNN language model, backpropagation through time, types of RNN - one to one, one to many, many to one and many to many along with different examples for each type.
- Introduction to Adversaries
- Language Models – Next Word Prediction, Spell Checkers, Mobile Auto-Correction, Speech Recognition & Machine Translation
- Traditional Language model
- Disadvantages of MLP
- Introduction to State & RNN cell
- Introduction to RNN
- RNN language Models
- Back Propagation Through time
- RNN Loss Computation
- Types of RNN – One to One, One to Many, Many to One, Many to Many
- Introduction to the CNN and RNN
- Combining CNN and RNN for Image Captioning
- Architecture of CNN and RNN for Image Captioning
- Bidirectional RNN
- Deep Bidirectional RNN
- Disadvantages of RNN
- Frequency-based Word Vectors
- Count Vectorization (Bag-of-Words, BoW), TF-IDF Vectorization
- Word Embeddings
- Word2Vec - CBOW & Skip-Gram
- FastText, GloVe
Faster object detection using YOLO models will be learnt along with setting up the environment. Learn pretrained models as well as building models from scratch.
- YOLO v3
- YOLO v4
- Darknet
- OpenVINO
- ONNX
- Fast R-CNN
- Faster R-CNN
- Mask R-CNN
Understand and implement Long Short-Term Memory, which is used to keep the information intact, unless the input makes them forget. You will also learn the components of LSTM - cell state, forget gate, input gate and the output gate along with the steps to process the information. Learn the difference between RNN and LSTM, Deep RNN and Deep LSTM and different terminologies. You will apply LSTM to build models for prediction.
Gated Recurrent Unit, a variant of LSTM solves this problem in RNN. You will learn the components of GRU and the steps to process the information.
- Introduction to LSTM – Architecture
- Importance of Cell State, Input Gate, Output Gate, Forget Gate, Sigmoid and Tanh
- Mathematical Calculations to Process Data in LSTM
- RNN vs LSTM - Bidirectional vs Deep Bidirectional RNN
- Deep RNN vs Deep LSTM
- Seq2Seq (Encoder - Decoder Model using RNN variants)
- Attention Mechanism
- Transformers (Encoder - Decoder Model by doing away from RNN variants)
- Bidirectional Encoder Representation from Transformer (BERT)
- OpenAI GPT-2 & GPT-3 Models (Generative Pre-Training)
- Text Summarization with T5
- Configurations of BERT
- Pre-Training the BERT Model
- ALBERT, RoBERTa, ELECTRA, SpanBERT, DistilBERT, TinyBERT
You will learn about the components of Autoencoders, steps used to train the autoencoders to generate spatial vectors, types of autoencoders and generation of data using variat
Course Fee Details
Virtual Instructor-led Training (VILT)
Mode of training: Live Online
- Live online classes - weekends & weekdays
- 365 days of access to online classes
- Avail Monthly EMI At zero Interest Rate
- Lifetime validity for LMS acces
- 20+ live hours of industry masterclasses from leading academicians and faculty from FT top 20 universities
- Career support services
INR 40,000
0 Learners
0 Reviews
Employee Upskilling
Mode of training: Onsite or Live Online
- On site or virtual based sessions
- Customised Course
- Curriculum with industry relevant use cases
- Pre & Post assessment service
- Complimentary basic Courses
- Corporate based learning management system with team and individual dashboard and reports
0 Learners
0 Reviews
Payment Accepted

All prices are applicable with 18% taxes.
Call us Today!
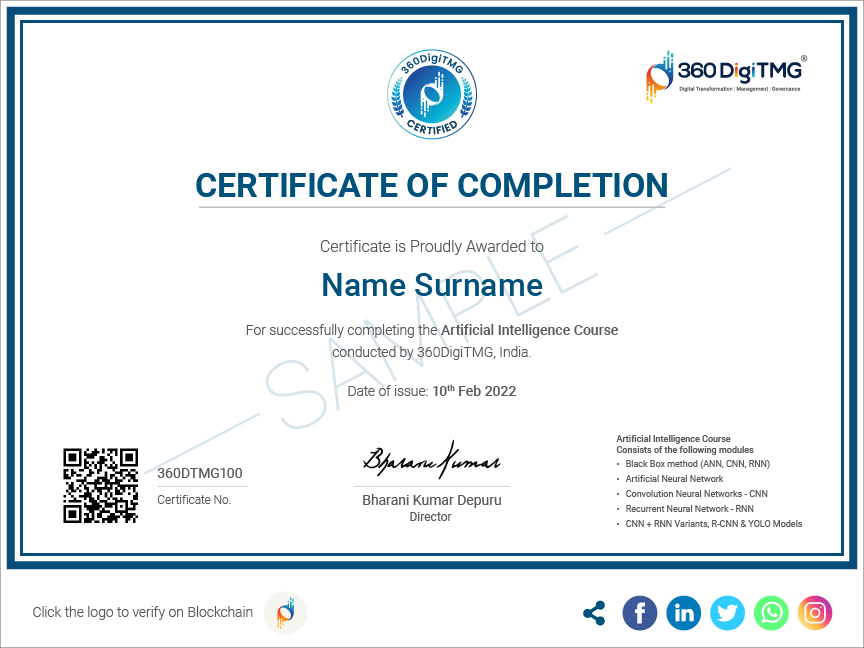
Certificate
Win recognition for your AI skills with the Certification Programme in AI and Deep Learning. This Artificial Intelligence and Deep Learning Course empowers you with knowledge that will help you blaze trails in your career. Distinguish yourself among peers and superiors and win recognition with this certificate from the best AI training institute in Gurgaon-360DigiTMG!
Alumni Speak
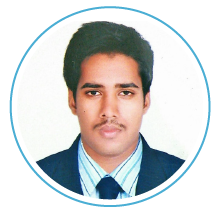
"The training was organised properly, and our instructor was extremely conceptually sound. I enjoyed the interview preparation, and 360DigiTMG is to credit for my successful placement.”
Pavan Satya
Senior Software Engineer

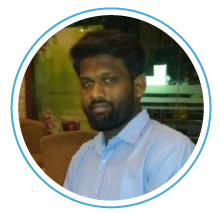
"Although data sciences is a complex field, the course made it seem quite straightforward to me. This course's readings and tests were fantastic. This teacher was really beneficial. This university offers a wealth of information."
Chetan Reddy
Data Scientist

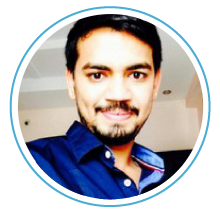
"The course's material and infrastructure are reliable. The majority of the time, they keep an eye on us. They actually assisted me in getting a job. I appreciated their help with placement. Excellent institution.”
Santosh Kumar
Business Intelligence Analyst

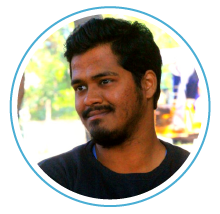
"Numerous advantages of the course. Thank you especially to my mentors. It feels wonderful to finally get to work.”
Kadar Nagole
Data Scientist

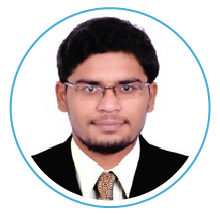
"Excellent team and a good atmosphere. They truly did lead the way for me right away. My mentors are wonderful. The training materials are top-notch.”
Gowtham R
Data Engineer

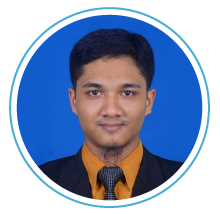
"The instructors improved the sessions' interactivity and communicated well. The course has been fantastic.”
Wan Muhamad Taufik
Associate Data Scientist

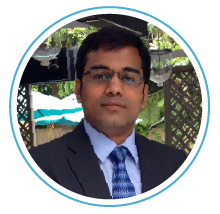
"The instructors went above and beyond to allay our fears. They assigned us an enormous amount of work, including one very difficult live project. great location for studying.”
Venu Panjarla
AVP Technology

Our Alumni Work At
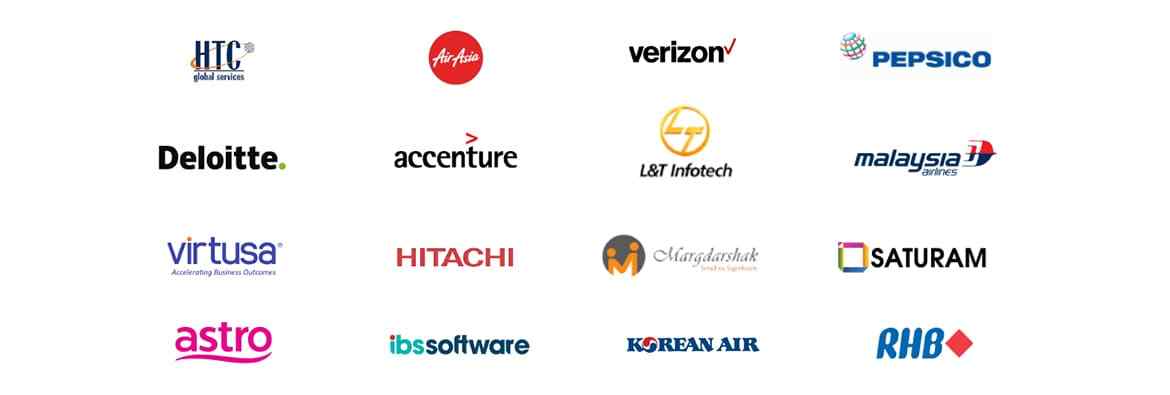
And more...
Companies That Trust Us
360DigiTMG offers customised corporate training programmes that suit the industry-specific needs of each company. Engage with us to design continuous learning programmes and skill development roadmaps for your employees. Together, let’s create a future-ready workforce that will enhance the competitiveness of your business.
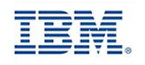
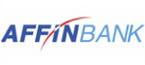
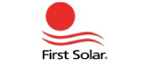
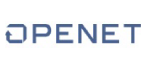
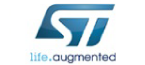
Student Voices