Certificate Course in
MLOps on Azure
- 60 Hours Interactive Online Sessions
- 80 Hours Assignments & Real-Time Projects
- Complimentary ML on Cloud Modules
- Complimentary Python Programming Course
- Complementary Kubernetes for Beginners
- Complimentary DevOps for Beginners

2064 Learners
Academic Partners & International Accreditations
The past decade has seen an explosion in the world of data science and machine learning with a lot of companies investing in these fields. However, companies and data scientists quickly realized that while building a model is simple, deploying them at scale is the real challenge. Organizations today are trying to incorporate ML and AI in their applications by adopting MLOps to gain agility and serve the real-world online application. MLOps (Machine Learning Operations) facilitates the management of ML Lifecycle by integrating Data Science and Operations. It also helps in building, training, and deploying machine learning models and workflows to get to production faster. To gain in-depth insights on the fundamentals of MLOps, join the MLOps on Azure course from 360DigTMG.
Course Fee
MLOps with Kubeflow Course Overview
This course gives you insights into the architecture of MLOps in the Azure Machine Learning environment. It aims to impart knowledge on how to perform MLOps efficiently using Microsoft cloud-native tools such as Azure Machine Learning, Azure Machine Learning Studio, Databricks, MLflow, Kubeflow, Apache Airflow among others. Students will learn to build ML pipelines to design, deploy, and manage model workflows to drive efficiency and productivity with MLOps.
Learn how monitoring and validation of machine learning models can speed up the pace of model development and deployment with MLOps. This course is designed for Data Scientist and Software Developers who wish to leverage Azure Machine Learning to facilitate MLOps practices. Join the ‘MLOps on Azure’ course with 360DigiTMG and learn to streamline and automate the machine learning life cycle by integrating DevOps processes.
What is MLOps?
Machine learning is quickly gaining a lot of traction and is becoming a technology that every company wants to implement but soon realize that creating and training an ML model is much easier than actually practically deploying that model. Machine Learning Operations (MLOps) is a discipline based on DevOps that accelerates the efficiency of workflows and enhances the quality of machine learning solutions.
MLOps on Azure Learning Outcomes
MLOps facilitates the development, deployment, management, and governance of ML models in production environments. This course introduces students to MLOps tools and best practices for building reproducible workflows and machine learning models. This course aims to be a set of guiding principles for MLOps on Azure. Needless to say, it is the result of experts in the domain distilling their hard work and knowledge and putting together a comprehensive guide to enable ML practitioners and Data Scientists to deploy models with ease. The objective of this course is to introduce learners to the MLDC also known as the Machine Learning Development Cycle using open source frameworks like MLflow, Kubeflow, Apache Airflow, Azure Machine Learning, etc. Participants will also learn about containers like Docker and containerized platforms like Kubernetes. So, prepare yourself for more lucrative career options with Industry-relevant curriculums and hands-on Capstone Project in the MLOps on Azure course. The participants will also learn:
Block Your Time
Who Should Sign Up?
- Data Scientists
- Data and Analytics Manager
- Business Analysts
- Data Engineers
- DevOps Engineers
- IT/Software Engineers
- Machine Learning Architects
- Model Risk Managers/Auditors
Modules for MLOps on Azure
MLOps is a fast-growing subdomain of the larger AI/ML/DS domain. It is a portmanteau of the words Machine Learning (ML) and DevOps, signifying that this domain is essentially an intersection of these two different disciplines. While ML deals with the development of algorithms and models using statistical and machine learning techniques, DevOps is focused on deploying the (ML model or any other) software applications into production using the Continuous Delivery and Continuous Integration principles. There is a need for source control, reproducible ML pipelines, model versioning and storage, model packaging, validation, deployment, and monitoring. Besides, there is also a need for model retraining based on the results of the monitoring activities. Currently, MLOps is being thought of as a concept, not a product or a service although a lot of effort is being put into either productizing it or providing it as a service. This course aims to introduce everyone to MLOps done right using Azure with Azure Machine Learning, MLflow, Databricks, and Kubeflow.
Data Scientists and ML practitioners usually are good at building models and performing complex statistical analysis but may not be good at software engineering skills required to put them into production. Also, a simple DevOps approach is not sufficient to address an ML project. It needs to take into account models, data, experiments, runs and artifacts produced. By way of this module we explore how model deployment is being done now vs how could the ideal state be.
Azure Machine Learning (AML) which is a cloud service offering has built-in MLOps. It can easily take care of the different phases of the Machine Learning Development Cycle (MLDC) and also offers complete integration right out of the box with popular ML frameworks like Scikit-learn, Pytorch, Tensorflow and MXNet. This module will provide a deeper look into how AML provides support for all the different phases of the MLDC.
Using Azure Pipelines, ML Engineers can automatically build and test ML projects. It seamlessly integrates CI (Continuous Integration) and CD (Continuous Delivery) and ships the ML model and supporting code to a target of choice. Azure Pipelines also facilitates CT (Continuous Testing) by helping automate the build, test and deploy processes efficiently.
Docker is a containerization mechanism to package the code and the underlying runtime as a Docker image to enable modularity and portability. The aim is to remove the occurrences of ‘it worked on my laptop’ by containerizing all the required elements of the model. Kubernetes is a container orchestration platform which helps in scheduling and orchestrating all the workloads among the different containers.
Understand how Azure ML helps in deploying the ML models by 1. ensuring that the source code is controlled, 2. Creating reproducible training cycles 3. Data and Environment management 4. Creating and maintaining ML pipelines 5. Utilizing model registry to track models and experiments 6. Evaluate and Validate the model 7. Monitor and retrain it as needed
MLflow is an open-source platform which can run on Azure (or any other public cloud or on-prem infrastructure) which also helps in managing the ML lifecycle. Understand the 4 major components
- MLflow Tracking
- MLflow projects
- MLflow Models
- Model registry and see how MLflow can be used in conjunction with Databricks on Azure to create an efficient MLOps framework.
Kubeflow is another open-source framework for MLOps which is gaining popularity very quickly. It is a framework which works on top of the Azure Kubernetes Service (AKS) and helps in orchestrating the different phases on MLDC without a lot of overhead of managing low level Kubernetes APIs. This module teaches how KFserving, Knative and other sub components of Kubeflow work on Azure to create another awesome MLOps framework.
Tools Covered
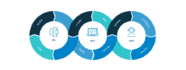
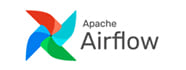
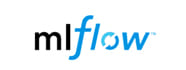
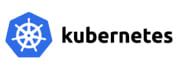
Trends of MLOps on Azure in India
The MLOps market is expected to grow to nearly US$4.1 billion by 2025. MLOps connects the development of the machine learning model and implements it in production. Linking these disparate areas of machine learning requires the right team, continuous integration, and deployment of data along with collaboration and communication between data scientists, developers and platform engineers.
AI and ML practices are soon becoming an intrinsic part of many modern-day business applications. But most organizations have not been successful in delivering AI-based applications and are only hamstrung with transforming data Science models into interactive applications capable of working with large data sets. To address this challenge a new practice called MLOps has emerged that integrates the AI/ML capabilities with DevOps practices. It aims to continuously develop and deliver data along with ML applications. So one of the trends we are going to see will be an increase in the demand for hybrid AI deployments and organizations will use accessible and production-ready data-science platforms.
How We Prepare You
-
Additional Assignments of over 80+ hours
-
Live Free Webinars
-
Resume and LinkedIn Review Sessions
-
Lifetime LMS Access
-
24/7 Support
-
Job Placements in Data Science Fields
-
Complimentary Courses
-
Unlimited Mock Interview and Quiz Session
-
Hands-on Experience in Capstone Projects
-
Life Time Free Access to Industry Webinars
Call us Today!
MLOps on Azure Panel of Coaches
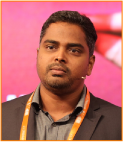
Bharani Kumar Depuru
- Areas of expertise: Data analytics, Digital Transformation, Industrial Revolution 4.0
- Over 18+ years of professional experience
- Trained over 2,500 professionals from eight countries
- Corporate clients include Deloitte, Hewlett Packard Enterprise, Amazon, Tech Mahindra, Cummins, Accenture, IBM
- Professional certifications - PMP, PMI-ACP, PMI-RMP from Project Management Institute, Lean Six Sigma Master Black Belt, Tableau Certified Associate, Certified Scrum Practitioner, (DSDM Atern)
- Alumnus of Indian Institute of Technology, Hyderabad and Indian School of Business
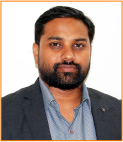
Sharat Chandra Kumar
- Areas of expertise: Data sciences, Machine learning, Business intelligence and Data
- Trained over 1,500 professional across 12 countries
- Worked as a Data scientist for 18+ years across several industry domains
- Professional certifications: Lean Six Sigma Green and Black Belt, Information Technology Infrastructure Library
- Experienced in Big Data Hadoop, Spark, NoSQL, NewSQL, MongoDB, Python, Tableau, Cognos
- Corporate clients include DuPont, All-Scripts, Girnarsoft (College-, Car-) and many more
.png)
Bhargavi Kandukuri
- Areas of expertise: Business analytics, Quality management, Data
visualisation with Tableau, COBOL, CICS, DB2 and JCL - Electronics and communications engineer with over 19+ years of industry experience
- Senior Tableau developer, with experience in analytics solutions development in domains such as retail, clinical and manufacturing
- Trained over 750+ professionals across the globe in three years
- Worked with Infosys Technologies, iGate, Patni Global Solutions as technology analyst
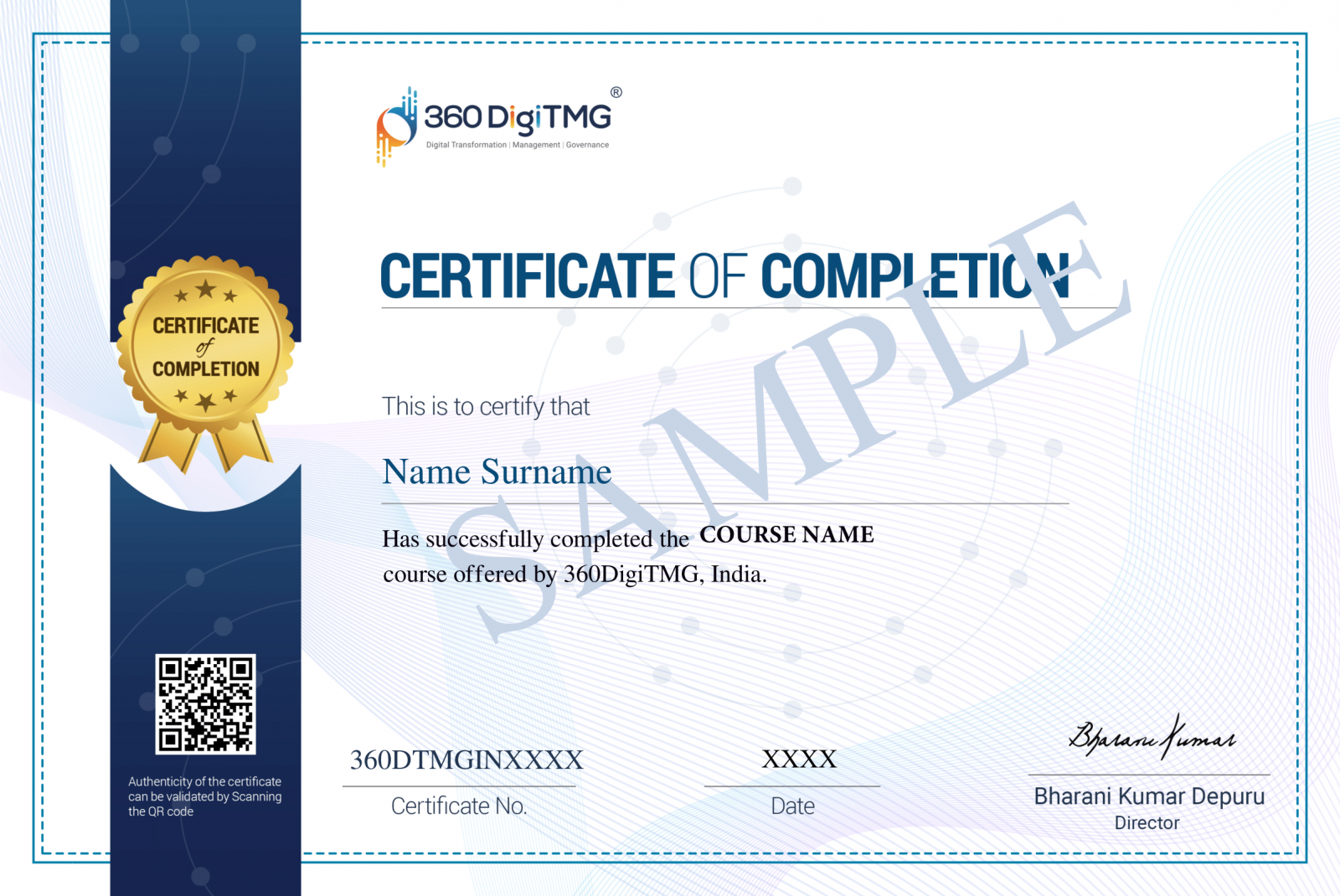
Certificate
Earn a certificate and demonstrate your commitment to the profession. Use it to distinguish yourself in the job market, get recognised at the workplace and boost your confidence. The MLOps on Azure Course Certificate is your passport to an accelerated career path.
Recommended Programmes
Data Science for Beginners using Python & R

2064 Learners
Big Data using Hadoop & Spark Course Training

3021 Learners
Artificial Intelligence (AI) & Deep Learning Course

2915 Learners
Alumni Speak
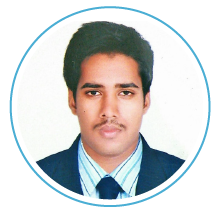
"The training was organised properly, and our instructor was extremely conceptually sound. I enjoyed the interview preparation, and 360DigiTMG is to credit for my successful placement.”
Pavan Satya
Senior Software Engineer

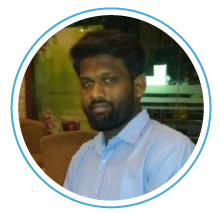
"Although data sciences is a complex field, the course made it seem quite straightforward to me. This course's readings and tests were fantastic. This teacher was really beneficial. This university offers a wealth of information."
Chetan Reddy
Data Scientist

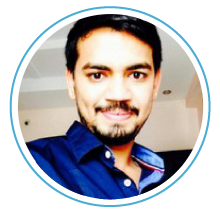
"The course's material and infrastructure are reliable. The majority of the time, they keep an eye on us. They actually assisted me in getting a job. I appreciated their help with placement. Excellent institution.”
Santosh Kumar
Business Intelligence Analyst

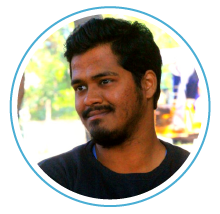
"Numerous advantages of the course. Thank you especially to my mentors. It feels wonderful to finally get to work.”
Kadar Nagole
Data Scientist

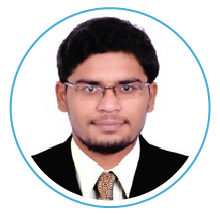
"Excellent team and a good atmosphere. They truly did lead the way for me right away. My mentors are wonderful. The training materials are top-notch.”
Gowtham R
Data Engineer

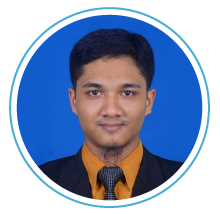
"The instructors improved the sessions' interactivity and communicated well. The course has been fantastic.”
Wan Muhamad Taufik
Associate Data Scientist

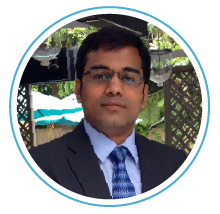
"The instructors went above and beyond to allay our fears. They assigned us an enormous amount of work, including one very difficult live project. great location for studying.”
Venu Panjarla
AVP Technology

Our Alumni Work At
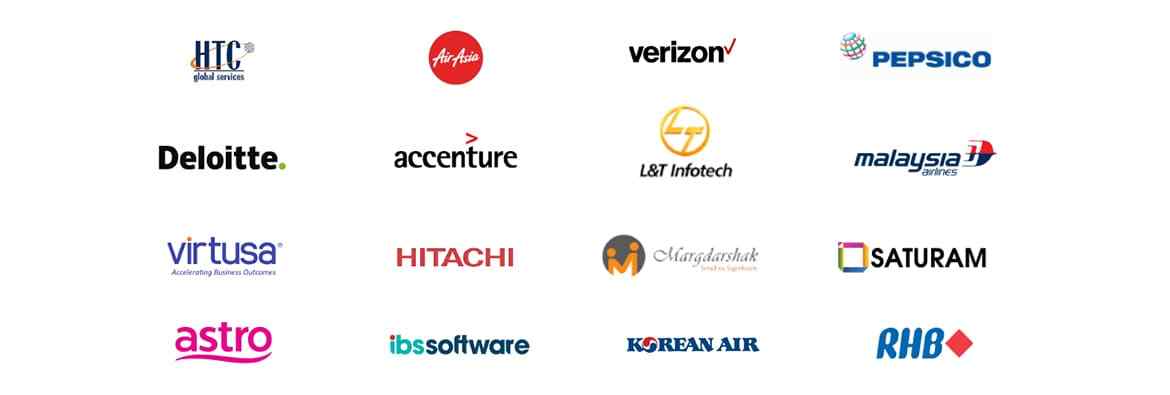
And more...
FAQs for MLOps on Azure Course Training
Basic Degree is required. Basic knowledge of Maths and Statistics is needed to learn the tools.
More than 20 assignments are provided for the students to make them proficient. A dedicated team of mentors will guide the students throughout their learning process.
MLOps is needed to enhance the machine learning-driven applications in business. This enables Data Scientists to concentrate on their work and empowers MLOps engineers to take responsibility and handle machine learning in production.
Machine Learning operations are considered to be the most valuable practices any company can have. It helps in improving quality and delivering better performance.
We provide a career coach to help you to build your portfolio and prepare you for facing interviews. Job placement Assistance.
Yes, you can attend a free demo class and can interact with the trainer to clarify your queries.
Yes, students will be given more than 3 real-time projects under the guidance of industry experts.
Students after completing the course will be prepared for interviews. Guidance will be given by conducting mock interviews and questionnaires. This session will help students to boost their confidence and improve their communication skills.
We provide online training with flexible timings along with online sessions.
You can clarify your doubts with trainers, and mentors are provided for the students to whom you can approach at any time.
You will be given LMS access, which helps you to revise the course and if you miss any class you can see the recorded version of the class. You can attend webinars for free that will be conducted on trending topics for a lifetime and many more.
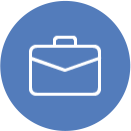
Jobs in the Field of MLOps on Azure
The various job profiles include Machine Learning Engineer, Machine Learning Analyst, NLP Data Scientist, Data Scientist, Data Mining Specialists, Cloud Architects, and Machine Learning Scientist, etc.
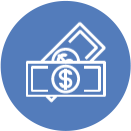
Salaries in India for MLOps on Azure
Tech-roles, particularly in the field of Data Science, ML and AL are gaining importance. A fresher in this field can fetch anything between ? 599,807- 791,326 and an experienced ML professional can get 13 to 22 lakhs per annum.
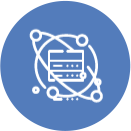
MLOps on Azure Projects in India
Practical insight into any technology is only possible through projects that help you master the technology. Some of the projects that one can undertake to gain practical experience include ‘Performing Data Visualization on Uber Data’ or ‘Game Prediction Project’ that involves predicting the interest of the users.
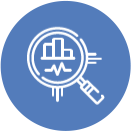
Role of Open Source Tools in MLOps
The various open-source MLOps tools give users and organizations a platform to collaborate. Some open-source tools include MetaFlow, MLRun, ML flow, MLReef, Seldon, etc.
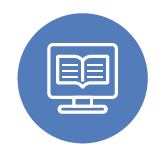
Modes of Training for MLOps on Azure
The course is designed to suit the needs of students as well as working professionals. We at 360DigiTMG give our students the option of interactive live online learning. We also support e-learning as part of our curriculum.
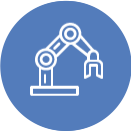
Industry Applications of MLOps
Machine Learning and AI are increasingly becoming key agents that are transforming the performance of businesses today who are leveraging the capabilities of MLOps for quicker deployment of ML models.
Companies That Trust Us
360DigiTMG offers customised corporate training programmes that suit the industry-specific needs of each company. Engage with us to design continuous learning programmes and skill development roadmaps for your employees. Together, let’s create a future-ready workforce that will enhance the competitiveness of your business.
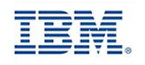
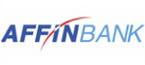
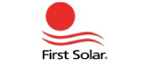
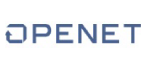
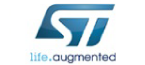
Student Voices