Advanced Data Science
- Get Trained by Trainers from ISB, IIT & IIM
- 184 Hours of Intensive Classroom & Online Sessions
- 2 Capstone Live Projects
- Job Placement Assistance

2064 Learners
Academic Partners & International Accreditations
The Indian Data Science Market will be worth 6 million dollars in 2025 and the Data analytics outsourcing market in India is worth $26 Billion." India will undoubtedly witness around three lakh job openings in data science by 2021. India is second to the United States in terms of the number of job openings in Data Science. In 2019, 98,123 positions in data science and analytics were vacant due to a lack of qualified candidates. The top sectors creating the most data science jobs are BFSI, Energy, Pharmaceutical, Healthcare, E-commerce, Media, and Retail. Today large companies, medium sized companies and even startups are willing to hire data scientists in India. The five most sought after digital skills are Big Data, software and user testing, Mobile Development, Cloud Computing, and software engineering management.
Data Science Course Programme Overview
This Data Science course using Python and R endorses the CRISP-DM Project Management methodology and contains a preliminary introduction of the same. Data Science is a 90% statistical analysis and it is only fair that the premier modules should bear an introduction to Statistical Data Business Intelligence and Data Visualization techniques. Students will grapple with Plots, Inferential Statistics, and various Probability Distributions in the module. A brief exposition on Exploratory Data Analysis/ Descriptive Analytics is huddled in between. The core modules commence with a focus on Hypothesis Testing and the "4" must know hypothesis tests. Data Mining with Supervised Learning and the use of Linear Regression and OLS to enable the same find mention in succeeding modules. The prominent use of Multiple Linear Regression to build Prediction Models is elaborated. The theory behind Lasso and Ridge Regressions, Logistic Regression, Multinomial Regression, and Advanced Regression For Count Data is discussed in the subsequent modules.
A separate module is devoted to Data Mining Unsupervised Learning where the techniques of Clustering, Dimension Reduction, and Association Rules are elaborated. The nitty-gritty of Recommendation Engines and Network Analytics are detailed in the following modules. The various Machine Learning algorithms follow next like k-NN Classifier, Decision Tree and Random Forest, Ensemble Techniques, Bagging and Boosting, Adaboost, and Extreme Gradient Boosting. Text Mining, Natural Language Processing, Naive Bayes, Perceptron, and Multilayer Perceptron are the focal points of the succeeding modules.
The fundamentals of Neural Network ANN and Deep Learning Black Box Techniques like CNN, RNN, and SVM find prominent features as well. The concluding modules contain model-driven and data-driven algorithms for Forecasting and Time Series Analysis.
What is Data Science?
Data science is an amalgam of methods derived from statistics, Data Analytics, and Machine Learning that are trained to extract and analyze huge volumes of structured and unstructured data.
Who is a Data Scientist?
A Data Scientist is a researcher who has to prepare huge volumes of big data for analysis, build complex quantitative algorithms to organize and synthesize the information, and present the findings with compelling visualizations to senior management.
A Data Scientist enhances business decision making by introducing greater speed and better direction to the entire process.
A Data Scientist must be a person who loves playing with numbers and figures. A strong analytical mindset coupled with strong industrial knowledge is the skill set most desired in a data scientist. He must possess above the average communication skills and must be adept in communicating the technical concepts to non - technical people. Data Scientists need a strong foundation in Statistics, Mathematics, Linear Algebra, Computer Programming, Data Warehousing, Mining, and modeling to build winning algorithms.
They must be proficient in tools such as Python, R, R Studio, Hadoop, MapReduce, Apache Spark, Apache Pig, Java, NoSQL database, Cloud Computing, Tableau, and SAS.
Data Science Training Learning Outcomes
Who Should Attend
Block Your Time
Who Should Sign Up?
- IT Engineers
- Data and Analytics Manager
- Business Analysts
- Data Engineers
- Banking and Finance Analysts
- Marketing Managers
- Supply Chain Professionals
- HR Managers
- Math, Science and Commerce Graduates
Data Science Certification Course Modules
Extension to logistic regression We have a multinomial regression technique used to predict multiple categorical outcomes. Understand the concept of multi logit equations, baseline, and making classifications using probability outcomes.
Learn about handling multiple categories in output variables including nominal as well as ordinal data.
- Logit and Log-Likelihood
- Category Baselining
- Modeling Nominal categorical data
- Handling Ordinal Categorical Data
- Interpreting the results of coefficient values
- Lasso Regression
- Ridge Regression
- Learn about overfitting and underfitting conditions for prediction models developed. We need to strike the right balance between overfitting and underfitting, learn about regularization techniques L1 norm and L2 norm used to reduce these abnormal conditions. The regression techniques Lasso and Ridge techniques are discussed in this module.
Personalized recommendations made in e-commerce are based on all the previous transactions made. Learn the science of making these recommendations using measuring similarity between customers. The various methods applied for collaborative filtering, their pros, and cons, SVD method used for recommendations of movies by Netflix will be discussed as part of this module.
- User-based Collaborative Filtering
- A measure of distance/similarity between users
- Driver for Recommendation
- Computation Reduction Techniques
- Search based methods/Item to Item Collaborative Filtering
- SVD in recommendation
- The vulnerability of recommendation systems
Learn about improving the reliability and accuracy of decision tree models using ensemble techniques. Bagging and Boosting are the go-to techniques in ensemble techniques. The parallel and sequential approaches are taken in Bagging and Boosting methods are discussed in this module.
- Overfitting
- Underfitting
- Pruning
- Boosting
- Bagging or Bootstrap aggregating
The study of a network with quantifiable values is known as network analytics. The vertex and edge are the node and connection of a network, learn about the statistics used to calculate the value of each node in the network. You will also learn about the google page ranking algorithm as part of this module.
- Definition of a network (the LinkedIn analogy)
- The measure of Node strength in a Network
- Degree centrality
- Closeness centrality
- Eigenvector centrality
- Adjacency matrix
- Betweenness centrality
- Cluster coefficient
- Introduction to Google page ranking
Tools Covered
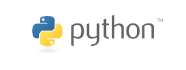
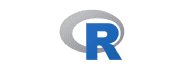
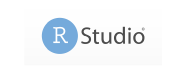
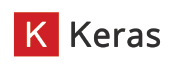
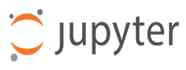
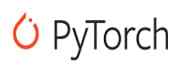
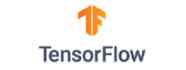
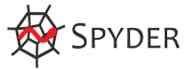
Data Science Course Trends in India
The demand for Data Scientists is predicted to increase by 30% by 2021. With the inclusion of cloud and IoT technologies there has been an exponential growth of Data that has led to the expansion of roles for data scientists in the field of Machine Learning and Big Data technology. In the times to come a Data scientist role will not be just subjected to technical aspects but will rise to more of a collaborator and a facilitators role. An entry-level fresher in Data Science earns around Rs.4.0 lakhs. And if he decides to stay put for another 5 to 10 years on the job, he gets a handsome promotion to the Rs 7 to 11 lakhs per annum layer. If he persists and dedicates a lifetime to data science he can garner anywhere from 25 lakhs to a whopping one crore per annum.
In India, Data scientists have 4 job hops in 8 years with 2 years tenure with each employer. Data Scientists normally get a 60-100% salary increase on job changes. First, the aspirant joins as a Data Scientist intern and as a Junior Data Scientist and then moves on to becoming a Senior Data Scientist. After this, he gets elevated to Principal Data Scientist and finally heads the Data Science vertical as Chief Data Scientist of the company. The top employers in Data Science are IBM, Accenture, JPMorgan Chase, Amex, McKinsey & Co, Impetus, Wipro, and Microsoft. Accenture offers the highest salary of Rs.19.6 lacs per annum.
Why 360DigiTMG?
-
Additional Assignments of over 150+ hours
-
Live Free Webinars
-
Resume and LinkedIn Review Sessions
-
Lifetime LMS Access
-
24/7 Support
-
Job Placements in Data Science fields
-
Complimentary Courses
-
Unlimited Mock Interview and Quiz Session
-
Hands-on Experience in Live Projects
-
Offline Hiring Events
Call us Today!
Data Scientist Course Panel of Coaches
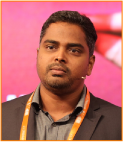
Bharani Kumar Depuru
- Areas of expertise: Data Analytics, Digital Transformation, Industrial Revolution 4.0
- Over 18+ years of professional experience
- Trained over 2,500 professionals from eight countries
- Corporate clients include Deloitte, Hewlett Packard Enterprise, Amazon, Tech Mahindra, Cummins, Accenture, IBM
- Professional certifications - PMP, PMI-ACP, PMI-RMP from Project Management Institute, Lean Six Sigma Master Black Belt, Tableau Certified Associate, Certified Scrum Practitioner, (DSDM Atern)
- Alumnus of Indian Institute of Technology, Hyderabad and Indian School of Business
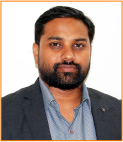
Sharat Chandra Kumar
- Areas of expertise: Data sciences, Machine learning, Business intelligence and Data Visualization
- Trained over 1,500 professional across 12 countries
- Worked as a Data scientist for 18+ years across several industry domains
- Professional certifications: Lean Six Sigma Green and Black Belt, Information Technology Infrastructure Library
- Experienced in Big Data Hadoop, Spark, NoSQL, NewSQL, MongoDB, Python, Tableau, Cognos
- Corporate clients include DuPont, All-Scripts, Girnarsoft (College-, Car-) and many more
.png)
Bhargavi Kandukuri
- Areas of expertise: Business analytics, Quality management, Data
visualisation with Tableau, COBOL, CICS, DB2 and JCL - Electronics and communications engineer with over 19+ years of industry experience
- Senior Tableau developer, with experience in analytics solutions development in domains such as retail, clinical and manufacturing
- Trained over 750+ professionals across the globe in three years
- Worked with Infosys Technologies, iGate, Patni Global Solutions as technology analyst
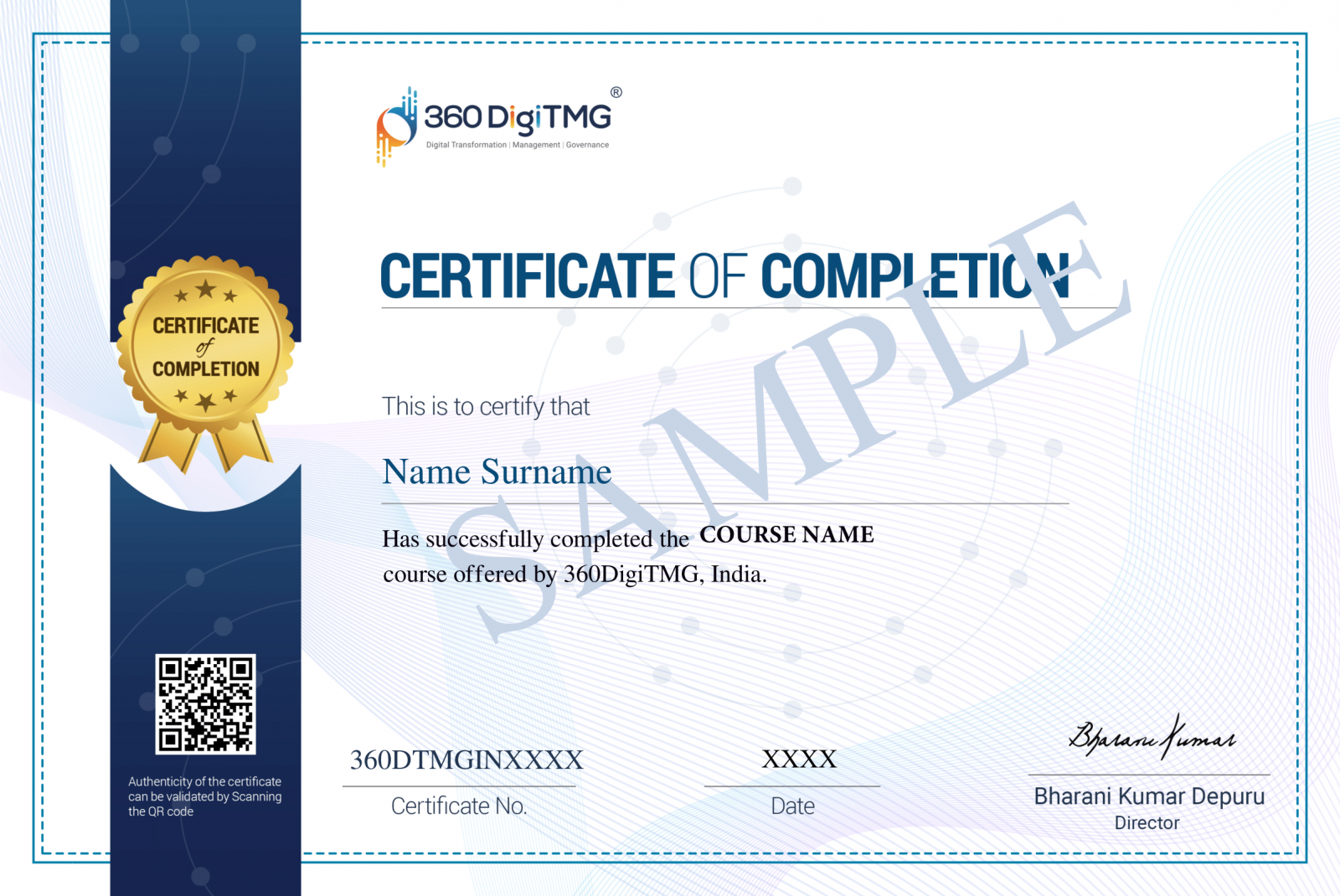
Certificate
Earn a certificate and demonstrate your commitment to the profession. Use it to distinguish yourself in the job market, get recognised at the workplace and boost your confidence. The Data Science Certificate is your passport to an accelerated career path.
Recommended Programmes
Data Science for Beginners using Python & R

2064 Learners
Big Data using Hadoop & Spark Course Training

3021 Learners
Artificial Intelligence (AI) & Deep Learning Course

2915 Learners
Alumni Speak
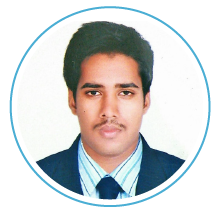
"The training was organised properly, and our instructor was extremely conceptually sound. I enjoyed the interview preparation, and 360DigiTMG is to credit for my successful placement.”
Pavan Satya
Senior Software Engineer

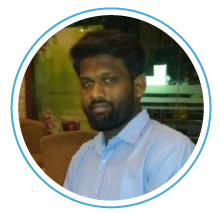
"Although data sciences is a complex field, the course made it seem quite straightforward to me. This course's readings and tests were fantastic. This teacher was really beneficial. This university offers a wealth of information."
Chetan Reddy
Data Scientist

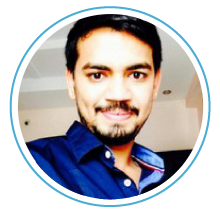
"The course's material and infrastructure are reliable. The majority of the time, they keep an eye on us. They actually assisted me in getting a job. I appreciated their help with placement. Excellent institution.”
Santosh Kumar
Business Intelligence Analyst

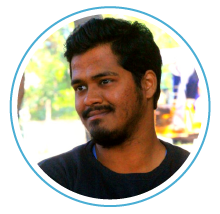
"Numerous advantages of the course. Thank you especially to my mentors. It feels wonderful to finally get to work.”
Kadar Nagole
Data Scientist

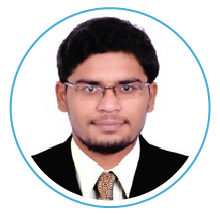
"Excellent team and a good atmosphere. They truly did lead the way for me right away. My mentors are wonderful. The training materials are top-notch.”
Gowtham R
Data Engineer

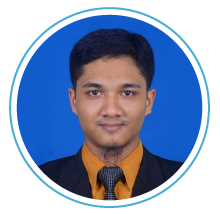
"The instructors improved the sessions' interactivity and communicated well. The course has been fantastic.”
Wan Muhamad Taufik
Associate Data Scientist

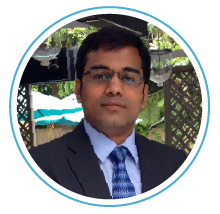
"The instructors went above and beyond to allay our fears. They assigned us an enormous amount of work, including one very difficult live project. great location for studying.”
Venu Panjarla
AVP Technology

Our Alumni Work At
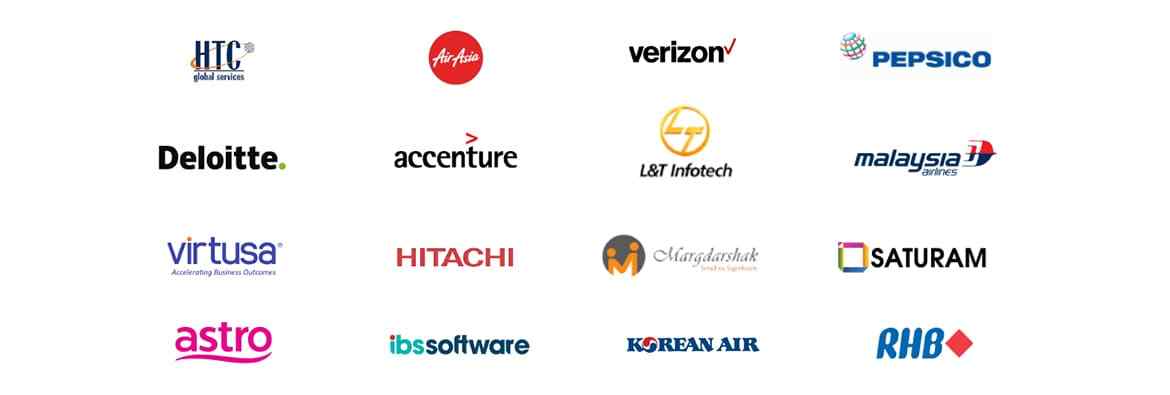
And more...
FAQs for Data Science Certification Training in India
After you have completed the classroom sessions, you will receive assignments through the online Learning Management System that you can access at your convenience. You will need to complete the assignments in order to obtain your data scientist certificate.
Different organisations use different terms for data professionals. You will sometimes find these terms being used interchangeably. Though there are no hard rules that distinguish one from another, you should get the role descriptions clarified before you join an organisation.
With growing demand, there is a scarcity of Data Science Professionals in the market. If you can demonstrate strong knowledge of Data Science concepts and algorithms, then there is a high chance for you to be able to make a career in this profession.
360DigiTMG provides internship opportunities through Innodatatics, our USA-based consulting partner, for deserving participants to help them gain real-life experience. This greatly helps students to bridge the gap between theory and practical.
While there are a number of roles pertaining to Data Professionals, most of the responsibilities overlap. However, the following are some basic job descriptions for each of these roles.
As a Data Analyst, you will be dealing with Data Cleansing, Exploratory Data Analysis and Data Visualisation
As a Data Scientist, you will be building algorithms to solve business problems using statistical tools such as Python, R, SAS, STATA, Matlab, Minitab, KNIME, Weka etc. A Data Scientist also performs predictive modelling to facilitate proactive decision-making.
A Data Engineer primarily does programming using Spark, Python, R etc. It often compliments the role of a Data Scientist.
A Data Architect has a much broader role that involves establishing the hardware and software infrastructure needed for an organisation to perform Data Analysis. They help in selecting the right database, servers, network architecture, GPUs, cores, memory, hard disk etc.
In this blended programme, you will be attending 184 hours of classroom sessions of 6 months. After completion, you will have access to the online Learning Management System for another three months for recorded videos and assignments. The total duration of assignments to be completed online is 150+ hour. Besides this, you will be working on 2 live projects for a month.
There are plenty of jobs available for data professionals. Once you complete the training, assignments and the live projects, we will send your resume to the organisations with whom we have formal agreements on job placements.
We also conduct webinars to help you with your resume and job interviews. We cover all aspects of post-training activities that are required to get a successful placement.
If you miss a class, we will arrange for a recording of the session. You can then access it through the online Learning Management System.
We assign mentors to each student in this programme. Additionally, during the mentorship session, if the mentor feels that you require additional assistance, you may be referred to another mentor or trainer.
No, the cost of the certificate is included in the programme package.
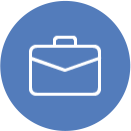
Jobs in the field of Data Science in India
The top sectors creating the most data science jobs are BFSI, Energy, Pharmaceutical, Healthcare, E-commerce, Media, and Retail. The maximum demand for Data Scientists are in the Metros cities like Delhi-NCR and Mumbai. It’s demand is also catching up in emerging cities like Pune and Bangalore.
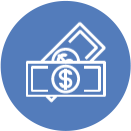
Salaries in India for Data Scientist
The average salary for a data scientist in India is Rs.10 lakhs per annum. Professionals specializing in advanced analytics and predictive modeling can command higher salaries.
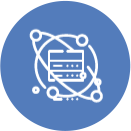
Data Science Projects in India
The Indian government has initiated several data science projects in the fields of Agriculture, Electricity, Water, Healthcare, Education, Road Traffic Safety, and Air Pollution. The Government of India has initiated several data science research initiatives as well.
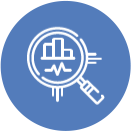
Role of Open Source Tools in Analytics
Python and R are easy to learn and maintain and therefore a Godsend to developers in Data Science. Their extended libraries make it possible to stretch the applications of Python from Big Data Analytics to Machine Learning.
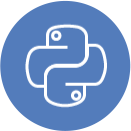
Modes of Training for Data Science with Python
The course in india is designed to suit the needs of students as well as working professionals. We at 360DigiTMG give our students the option of both classroom and online learning. We also support e-learning as part of our curriculum.
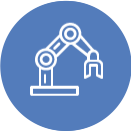
Industry Application of Data Science
Data Science is used for securities fraud early warning, card fraud detection systems, demand enterprise risk management, analysis of healthcare information, seismic interpretation, reservoir characterization, energy exploration, traffic control, and route planning.
Companies That Trust Us
360DigiTMG offers customised corporate training programmes that suit the industry-specific needs of each company. Engage with us to design continuous learning programmes and skill development roadmaps for your employees. Together, let’s create a future-ready workforce that will enhance the competitiveness of your business.
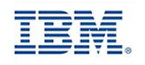
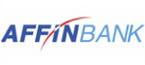
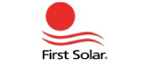
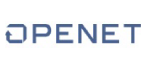
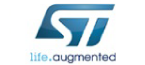
Student Voices