Sent Successfully.
Data Science Course in Bangalore
In Collaboration with

Avail Best data science certification course training in Bangalore and kick start your career as a successful Data Scientist within 4 months. Learn the advanced concepts and get your skills upgraded from the pioneers in Data Science Course.
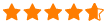
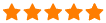

96%
of participants who met the conditions got placed

98%
Program Satisfaction

98%
Program Completion Rate
The dynamic field of Data Science is leading the world to understand extensive data and data analytics better and obtain valuable insights and information. It's a multidisciplinary and broad-spectrum field with significant benefits.
A recent study shows that by 2026, the need for a data scientist will increase by 27.6%. This skill set guarantees greater job security and a good salary. Understand how a Data science certification in Bangalore from 360DigiTMG, in collaboration with SUNY, can accelerate your career with its world-class skills and training in just four months. This course offers a broad exposure and knowledge of recent technologies, including Tableau, Python other machine learning concepts.
Tools Covered
The Data Science course is vast and consists of many interesting concepts like Big Data, Machine Learning, Data Warehousing, Data Mining and Visualization, Forecasting, Cloud Computing, Deep Learning, Neural Networks, and Business Intelligence. The Data Science Training in Bangalore uses various tools for this process.
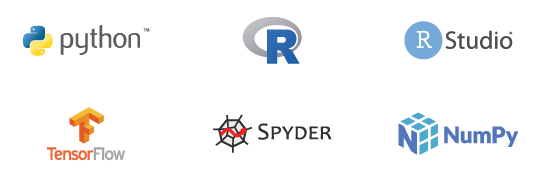
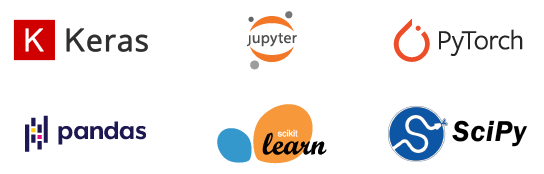
Data Science Course Industry Masterclass by 20+ Years of Experts
360DigiTMG is considered to be the best data science course in Bangalore with 20+ years of experienced professionals and Industry Experts as teaching professionals. Practical knowledge imparting gives way to faster learning; it bridges the gap between traditional theoretical teaching and its application in industries. The expert's positive and effective utilization of the industry network also helps them mentor the students better, get accessible internships, help create a better student portfolio, and provide career counseling.
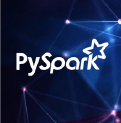
Distributed Computing Spark & PySpark
Processing big data with lightning speed
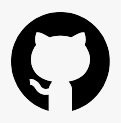
Git Account creation & Google Colab
Master project management and cloud-based GUI
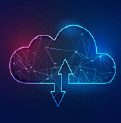
ML on Cloud - AWS, Azure, GCP - AutoML
Build ML algorithms without writing a single line of code
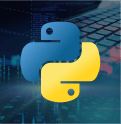
Data Ingestion using Python (Included in Python Programming)
Must know skill to begin data science journey
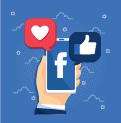
Facebook Prophet & ARIMA Variants
New era of timeseries / forecasting algorithms
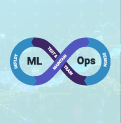
MLOps
New breed of end-to-end seamless pipeline building
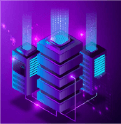
Data Engineering - Data Warehouse, ETL, ELT, Data Lake, Data Lakehouse, Data Pipeline, etc.
Boasts of more in-demand skill than data science
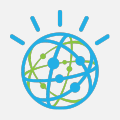
SUNY Watson Cognitive Computing AutoML By IBM
Experience the enhanced SUNY Watson capability
Data Science Certificate from Industry Leaders
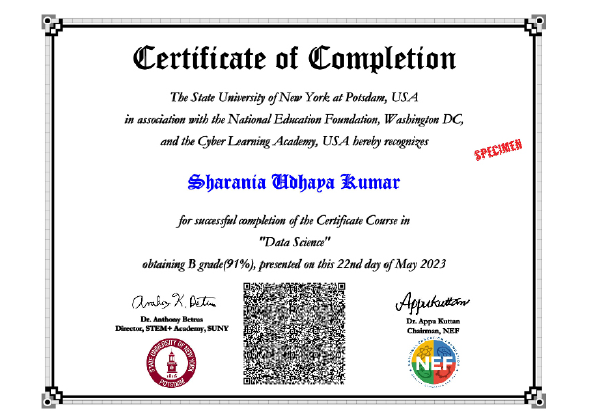
In terms of providing cognitive approaches and consulting services, SUNY is a pioneer.
SUNY invests $6 billion yearly in development and research and has long-standing expertise in data sciences and artificial intelligence.
The goal of 360DigiTMG's partnership with SUNY is to help introduce learners in order integrated blended educational experiences with the aid of our well designed, globally recognised curriculum.
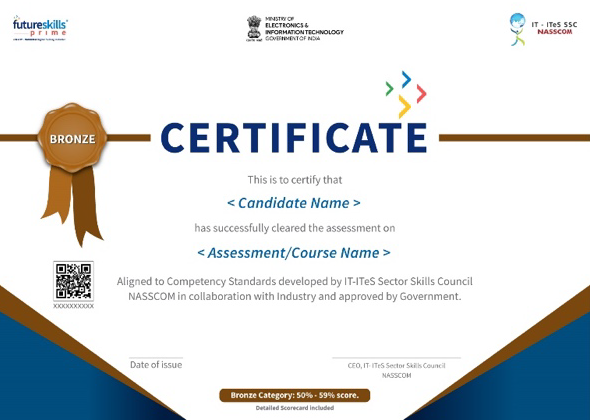
A renowned trade organisation in India that supports and advances the goals of the IT and business process management (BPM) industries is called NASSCOM.
For decades, NASSCOM has been actively engaged in research and development related to data science and artificial intelligence.
The goal of 360DigiTMG's partnership with NASSCOM is to empower students all around the world by introducing them to multimedia blended learning through the use of our top-notch, industry-aligned curriculum.
Data Science Course Fee in Bangalore
Virtual Instructor-led Training (VILT)
- Live online classes - weekends & weekdays
- 365 days of access to online classes
- Avail Monthly EMI At zero Interest Rate
- Lifetime validity for LMS access
- 24+ live hours of industry masterclasses from leading academicians and faculty from FT top 20 universities
- Career support services
Data Science Course Training Overview in Bangalore
Bengaluru is the official Silicon Valley of Asia, with nearly 4.1 million people working in the IT sector. Data Science jobs are amongst the top-paying jobs in the market now. The average salary ranges between 6,00,000-7,00,000 INR Per annum, even for freshers.
Only comprehensive data science courses in Bangalore can quench your thirst for knowledge by providing specially tailored certification courses. The driven staff team prepares you for real-life situations and a better job role. These courses are ideal for data professionals and beginners who want to create or grow their careers in Big Data.
Why 360DigiTMG for Data Science Training in Bangalore?
360DigiTMG brings to you the most comprehensive Data Science Training in Bangalore that will expose the learners to the various stages of the Data Science Life cycle. The program on Data Science using Python will enable learners to gain expertise in analytics using the Python language. This course will cover topics like data exploration, data visualization, descriptive analytics, and predictive analytics techniques that will enable students as well as professionals to take up data science skills into a variety of companies. The goal of the training program is to teach you the various foundational concepts of Statistics, Mathematics, Business Intelligence, and Exploratory Data Analytics.
A module is dedicated to scripting Machine Learning Algorithms and enabling Deep Learning and Neural Networks with Black Box techniques and SVM. All the stages delineated in the CRISP-ML(Q) framework for a Data Science Projects are dealt with in great depth and clarity in this course. Undoubtedly this emerges as one of the best courses due to the live project exposure in INNODATATICS. This gives a golden opportunity for students to apply the various concepts studies to a real-time situation.
What is Data Science?
Data science is an amalgam of methods derived from statistics, data analytics, and machine learning that are trained to extract and analyze huge volumes of structured and unstructured data.
Who is a Data Scientist?
A Data Scientist is a researcher who has to prepare huge volumes of big data for Data Science, build complex quantitative algorithms to organize and synthesize the information, and present the findings with compelling visualizations to senior management. A Data Science enhances business decision making by introducing greater speed and better direction to the entire process.
A Data Scientist is the sexiest job of the 21st century Stated by Harvard Business Review must be a person who loves playing with numbers and figures. A strong analytical mindset coupled with strong industrial knowledge is the skill set most desired in a Data Scientist. He must possess above average communication skills and must be adept in communicating the technical concepts to non-technical people.
Data Scientists need a strong foundation in Statistics, Mathematics, Linear Algebra, Computer Programming, Data Warehousing, Mining, and Modeling to build winning algorithms. Having proficiency in tools such as Python, RStudio, Hadoop, MapReduce, Apache Spark, Apache Pig, Java, NoSQL database, Cloud Computing, Tableau, and SAS is beneficial, but not mandatory.
Learning Outcomes of Data Science Institute in Bangalore
Every organization is looking for ways to deal with its humongous data in this fast-paced environment. The demand for Big data skills and technology is in a surge nowadays. It is one of the leading and competitive fields in the IT sector now. Our Data Science Training Institute in Bangalore helps equip students with relevant and logical programming abilities to meet industry standards.
In the three months, students will explore critical techniques like Regression analysis, Data Mining, Statistical Analysis, Machine learning, and Forecasting within scripting algorithms for Python Programming. Be job ready when you finish this Data Science Certification course in Bangalore.
Work with various data generation sources
Perform Text Mining to generate Customer Sentiment Analysis
Develop an understanding of Descriptive and Predictive Analytics
Apply Data-driven, Machine Learning approaches for business decisions
Build models for day-to-day applicability
Perform Forecasting to take proactive business decisions
Use Data Concepts to represent data for easy understanding
Block Your Time
Who Should Sign Up?
- IT Engineers
- Data and Analytics Manager
- Business Analysts
- Data Engineers
- Banking and Finance Analysts
- Marketing Managers
- Supply Chain Professionals
- HR Managers
Syllabus of Data Scientist Courses in Bangalore
This data science program follows the CRISP-ML(Q) Methodology. The premier modules are devoted to a foundational perspective of Statistics, Mathematics, Business Intelligence, and Exploratory Data Science. The successive modules deal with Probability Distribution, Hypothesis Testing, Data Mining Supervised, Predictive Modelling - Multiple Linear Regression, Lasso And Ridge Regression, Logistic Regression, Multinomial Regression, and Ordinal Regression. Later modules deal with Data Mining Unsupervised Learning, Recommendation Engines, Network Analytics, Machine Learning, Decision Tree and Random Forest, Text Mining, and Natural Language Processing. The final modules deal with Machine Learning - classifier techniques, Perceptron, Multilayer Perceptron, Neural Networks, Deep Learning Black-Box Techniques, SVM, Forecasting, and Time Series algorithms. This is the most enriching training program in terms of the array of topics covered.
- Introduction to Python Programming
- Installation of Python & Associated Packages
- Graphical User Interface
- Installation of Anaconda Python
- Setting Up Python Environment
- Data Types
- Operators in Python
- Arithmetic operators
- Relational operators
- Logical operators
- Assignment operators
- Bitwise operators
- Membership operators
- Identity operators
- Check out the Top Python Programming Interview Questions and Answers here.
- Data structures
- Vectors
- Matrix
- Arrays
- Lists
- Tuple
- Sets
- String Representation
- Arithmetic Operators
- Boolean Values
- Dictionary
- Conditional Statements
- if statement
- if - else statement
- if - elif statement
- Nest if-else
- Multiple if
- Switch
- Loops
- While loop
- For loop
- Range()
- Iterator and generator Introduction
- For – else
- Break
- Functions
-
- Purpose of a function
- Defining a function
- Calling a function
- Function parameter passing
- Formal arguments
- Actual arguments
- Positional arguments
- Keyword arguments
- Variable arguments
- Variable keyword arguments
- Use-Case *args, **kwargs
-
- Function call stack
- Locals()
- Globals()
- Stackframe
- Modules
- Python Code Files
- Importing functions from another file
- __name__: Preventing unwanted code execution
- Importing from a folder
- Folders Vs Packages
- __init__.py
- Namespace
- __all__
- Import *
- Recursive imports
- File Handling
- Exception Handling
- Regular expressions
- Oops concepts
- Classes and Objects
- Inheritance and Polymorphism
- Multi-Threading
- What is a Database
- Types of Databases
- DBMS vs RDBMS
- DBMS Architecture
- Normalisation & Denormalization
- Install PostgreSQL
- Install MySQL
- Data Models
- DBMS Language
- ACID Properties in DBMS
- What is SQL
- SQL Data Types
- SQL commands
- SQL Operators
- SQL Keys
- SQL Joins
- GROUP BY, HAVING, ORDER BY
- Subqueries with select, insert, update, delete statements
- Views in SQL
- SQL Set Operations and Types
- SQL functions
- SQL Triggers
- Introduction to NoSQL Concepts
- SQL vs NoSQL
- Database connection SQL to Python
- Check out the SQL for Data Science One Step Solution for Beginners here.
Learn about insights on how data is assisting organizations to make informed data-driven decisions. Gathering the details about the problem statement would be the first step of the project. Learn the know-how of the Business understanding stage. Deep dive into the finer aspects of the management methodology to learn about objectives, constraints, success criteria, and the project charter. The essential task of understanding business Data and its characteristics is to help you plan for the upcoming stages of development. Check out the CRISP - Business Understanding here.
- All About 360DigiTMG & Innodatatics Inc., USA
- Dos and Don'ts as a participant
- Introduction to Big Data Science
- Data and its uses – a case study (Grocery store)
- Interactive marketing using data & IoT – A case study
- Course outline, road map, and takeaways from the course
- Stages of Analytics - Descriptive, Predictive, Prescriptive, etc.
- Cross-Industry Standard Process for Data Mining
- Typecasting
- Handling Duplicates
- Outlier Analysis/Treatment
- Winsorization
- Trimming
- Local Outlier Factor
- Isolation Forests
- Zero or Near Zero Variance Features
- Missing Values
- Imputation (Mean, Median, Mode, Hot Deck)
- Time Series Imputation Techniques
- 1) Last Observation Carried Forward (LOCF)
- 2) Next Observation Carried Backward (NOCB)
- 3) Rolling Statistics
- 4) Interpolation
- Discretization / Binning / Grouping
- Encoding: Dummy Variable Creation
- Transformation
- Transformation - Box-Cox, Yeo-Johnson
- Scaling: Standardization / Normalization
- Imbalanced Handling
- SMOTE
- MSMOTE
- Undersampling
- Oversampling
In this module, you will learn about dealing with the Data after the Collection. Learn to extract meaningful information about Data by performing Uni-variate analysis which is the preliminary step to churn the data. The task is also called Descriptive Analytics or also known as Exploratory Data Science. In this module, you also are introduced to statistical calculations which are used to derive information along with Visualizations to show the information in graphs/plots
- Machine Learning project management methodology
- Data Collection - Surveys and Design of Experiments
- Data Types namely Continuous, Discrete, Categorical, Count, Qualitative, Quantitative and its identification and application
- Further classification of data in terms of Nominal, Ordinal, Interval & Ratio types
- Balanced versus Imbalanced datasets
- Cross Sectional versus Time Series vs Panel / Longitudinal Data
- Time Series - Resampling
- Batch Processing vs Real Time Processing
- Structured versus Unstructured vs Semi-Structured Data
- Big vs Not-Big Data
- Data Cleaning / Preparation - Outlier Analysis, Missing Values Imputation Techniques, Transformations, Normalization / Standardization, Discretization
- Sampling techniques for handling Balanced vs. Imbalanced Datasets
- What is the Sampling Funnel and its application and its components?
- Population
- Sampling frame
- Simple random sampling
- Sample
- Measures of Central Tendency & Dispersion
- Population
- Mean/Average, Median, Mode
- Variance, Standard Deviation, Range
The raw Data collected from different sources may have different formats, values, shapes, or characteristics. Cleansing, or Data Preparation, Data Munging, Data Wrapping, etc., are the next steps in the Data handling stage. The objective of this stage is to transform the Data into an easily consumable format for the next stages of development.
- Feature Engineering on Numeric / Non-numeric Data
- Feature Extraction
- Feature Selection
- Forward Feature Selection
- Backward Feature Selection
- Exhaustive Feature Selection
- Recursive feature elimination (RFE)
- Chi-square Test
- Information Gain
- What is Power BI?
- Power BI Tips and Tricks & ChatGPT Prompts
- Overview of Power BI
- Architecture of PowerBI
- PowerBI and Plans
- Installation and introduction to PowerBI
- Transforming Data using Power BI Desktop
- Importing data
- Changing Database
- Data Types in PowerBI
- Basic Transformations
- Managing Query Groups
- Splitting Columns
- Changing Data Types
- Working with Dates
- Removing and Reordering Columns
- Conditional Columns
- Custom columns
- Connecting to Files in a Folder
- Merge Queries
- Query Dependency View
- Transforming Less Structured Data
- Query Parameters
- Column profiling
- Query Performance Analytics
- M-Language
Learn the preliminaries of the Mathematical / Statistical concepts which are the foundation of techniques used for churning the Data. You will revise the primary academic concepts of foundational mathematics and Linear Algebra basics. In this module, you will understand the importance of Data Optimization concepts in Machine Learning development. Check out the Mathematical Foundations here.
- Data Optimization
- Derivatives
- Linear Algebra
- Matrix Operations
Data mining unsupervised techniques are used as EDA techniques to derive insights from the business data. In this first module of unsupervised learning, get introduced to clustering algorithms. Learn about different approaches for data segregation to create homogeneous groups of data. In hierarchical clustering, K means clustering is the most used clustering algorithm. Understand the different mathematical approaches to perform data segregation. Also, learn about variations in K-means clustering like K-medoids, and K-mode techniques, and learn to handle large data sets using the CLARA technique.
- Clustering 101
- Distance Metrics
- Hierarchical Clustering
- Non-Hierarchical Clustering
- DBSCAN
- Clustering Evaluation metrics
Dimension Reduction (PCA and SVD) / Factor Analysis Description: Learn to handle high dimensional data. The performance will be hit when the data has a high number of dimensions and machine learning techniques training becomes very complex, as part of this module you will learn to apply data reduction techniques without any variable deletion. Learn the advantages of dimensional reduction techniques. Also, learn about yet another technique called Factor Analysis.
- Prinicipal Component Analysis (PCA)
- Singular Value Decomposition (SVD)
Learn to measure the relationship between entities. Bundle offers are defined based on this measure of dependency between products. Understand the metrics Support, Confidence, and Lift used to define the rules with the help of the Apriori algorithm. Learn the pros and cons of each of the metrics used in Association rules.
- Association rules mining 101
- Measurement Metrics
- Support
- Confidence
- Lift
- User Based Collaborative Filtering
- Similarity Metrics
- Item Based Collaborative Filtering
- Search Based Methods
- SVD Method
The study of a network with quantifiable values is known as network analytics. The vertex and edge are the nodes and connection of a network, learn about the statistics used to calculate the value of each node in the network. You will also learn about the google page ranking algorithm as part of this module.
- Entities of a Network
- Properties of the Components of a Network
- Measure the value of a Network
- Community Detection Algorithms
Learn to analyse unstructured textual data to derive meaningful insights. Understand the language quirks to perform data cleansing, extract features using a bag of words and construct the key-value pair matrix called DTM. Learn to understand the sentiment of customers from their feedback to take appropriate actions. Advanced concepts of text mining will also be discussed which help to interpret the context of the raw text data. Topic models using LDA algorithm, emotion mining using lexicons are discussed as part of NLP module.
- Sources of data
- Bag of words
- Pre-processing, corpus Document Term Matrix (DTM) & TDM
- Word Clouds
- Corpus-level word clouds
- Sentiment Analysis
- Positive Word clouds
- Negative word clouds
- Unigram, Bigram, Trigram
- Semantic network
- Extract, user reviews of the product/services from Amazon and tweets from Twitter
- Install Libraries from Shell
- Extraction and text analytics in Python
- LDA / Latent Dirichlet Allocation
- Topic Modelling
- Sentiment Extraction
- Lexicons & Emotion Mining
- Check out the Text Mining Interview Questions and Answers here.
- Machine Learning primer
- Difference between Regression and Classification
- Evaluation Strategies
- Hyper Parameters
- Metrics
- Overfitting and Underfitting
Revise Bayes theorem to develop a classification technique for Machine learning. In this tutorial, you will learn about joint probability and its applications. Learn how to predict whether an incoming email is spam or a ham email. Learn about Bayesian probability and its applications in solving complex business problems.
- Probability – Recap
- Bayes Rule
- Naïve Bayes Classifier
- Text Classification using Naive Bayes
- Checking for Underfitting and Overfitting in Naive Bayes
- Generalization and Regulation Techniques to avoid overfitting in Naive Bayes
- Check out the Naive Bayes Algorithm here.
k Nearest Neighbor algorithm is a distance-based machine learning algorithm. Learn to classify the dependent variable using the appropriate k value. The KNN Classifier also known as a lazy learner is a very popular algorithm and one of the easiest for application.
- Deciding the K value
- Thumb rule in choosing the K value.
- Building a KNN model by splitting the data
- Checking for Underfitting and Overfitting in KNN
- Generalization and Regulation Techniques to avoid overfitting in KNN
In this tutorial, you will learn in detail about the continuous probability distribution. Understand the properties of a continuous random variable and its distribution under normal conditions. To identify the properties of a continuous random variable, statisticians have defined a variable as a standard, learning the properties of the standard variable and its distribution. You will learn to check if a continuous random variable is following normal distribution using a normal Q-Q plot. Learn the science behind the estimation of value for a population using sample data.
- Probability & Probability Distribution
- Continuous Probability Distribution / Probability Density Function
- Discrete Probability Distribution / Probability Mass Function
- Normal Distribution
- Standard Normal Distribution / Z distribution
- Z scores and the Z table
- QQ Plot / Quantile - Quantile plot
- Sampling Variation
- Central Limit Theorem
- Sample size calculator
- Confidence interval - concept
- Confidence interval with sigma
- T-distribution Table / Student's-t distribution / T table
- Confidence interval
- Population parameter with Standard deviation known
- Population parameter with Standard deviation not known
Learn to frame business statements by making assumptions. Understand how to perform testing of these assumptions to make decisions for business problems. Learn about different types of Hypothesis testing and its statistics. You will learn the different conditions of the Hypothesis table, namely Null Hypothesis, Alternative hypothesis, Type I error, and Type II error. The prerequisites for conducting a Hypothesis test, and interpretation of the results will be discussed in this module.
- Formulating a Hypothesis
- Choosing Null and Alternative Hypotheses
- Type I or Alpha Error and Type II or Beta Error
- Confidence Level, Significance Level, Power of Test
- Comparative study of sample proportions using Hypothesis testing
- 2 Sample t-test
- ANOVA
- 2 Proportion test
- Chi-Square test
Data Mining supervised learning is all about making predictions for an unknown dependent variable using mathematical equations explaining the relationship with independent variables. Revisit the school math with the equation of a straight line. Learn about the components of Linear Regression with the equation of the regression line. Get introduced to Linear Regression analysis with a use case for the prediction of a continuous dependent variable. Understand about ordinary least squares technique.
- Scatter diagram
- Correlation analysis
- Correlation coefficient
- Ordinary least squares
- Principles of regression
- Simple Linear Regression
- Exponential Regression, Logarithmic Regression, Quadratic or Polynomial Regression
- Confidence Interval versus Prediction Interval
- Heteroscedasticity / Equal Variance
- Check out the Linear Regression Interview Questions and Answers here.
In the continuation of the Regression analysis study, you will learn how to deal with multiple independent variables affecting the dependent variable. Learn about the conditions and assumptions to perform linear regression analysis and the workarounds used to follow the conditions. Understand the steps required to perform the evaluation of the model and to improvise the prediction accuracies. You will be introduced to concepts of variance and bias.
- LINE assumption
- Linearity
- Independence
- Normality
- Equal Variance / Homoscedasticity
- Collinearity (Variance Inflation Factor)
- Multiple Linear Regression
- Model Quality metrics
- Deletion Diagnostics
- Check out the Linear Regression Interview Questions here.
You have learned about predicting a continuous dependent variable. As part of this module, you will continue to learn Regression techniques applied to predict attribute Data. Learn about the principles of the logistic regression model, understand the sigmoid curve, and the usage of cut-off value to interpret the probable outcome of the logistic regression model. Learn about the confusion matrix and its parameters to evaluate the outcome of the prediction model. Also, learn about maximum likelihood estimation.
- Principles of Logistic regression
- Types of Logistic regression
- Assumption & Steps in Logistic regression
- Analysis of Simple logistic regression results
- Multiple Logistic regression
- Confusion matrix
- False Positive, False Negative
- True Positive, True Negative
- Sensitivity, Recall, Specificity, F1
- Receiver operating characteristics curve (ROC curve)
- Precision Recall (P-R) curve
- Lift charts and Gain charts
- Check out the Logistic Regression Interview Questions and Answers here.
Learn about overfitting and underfitting conditions for prediction models developed. We need to strike the right balance between overfitting and underfitting, learn about regularization techniques L1 norm and L2 norm used to reduce these abnormal conditions. The regression techniques of Lasso and Ridge techniques are discussed in this module.
- Understanding Overfitting (Variance) vs. Underfitting (Bias)
- Generalization error and Regularization techniques
- Different Error functions, Loss functions, or Cost functions
- Lasso Regression
- Ridge Regression
- Check out the Lasso and Ridge Regression Interview Questions and Answers here.
Extension to logistic regression We have multinomial and Ordinal Logistic regression techniques used to predict multiple categorical outcomes. Understand the concept of multi-logit equations, baseline, and making classifications using probability outcomes. Learn about handling multiple categories in output variables including nominal as well as ordinal data.
- Logit and Log-Likelihood
- Category Baselining
- Modeling Nominal categorical data
- Handling Ordinal Categorical Data
- Interpreting the results of coefficient values
As part of this module, you learn further different regression techniques used for predicting discrete data. These regression techniques are used to analyze the numeric data known as count data. Based on the discrete probability distributions namely Poisson, negative binomial distribution the regression models try to fit the data to these distributions. Alternatively, when excessive zeros exist in the dependent variable, zero-inflated models are preferred, you will learn the types of zero-inflated models used to fit excessive zeros data.
- Poisson Regression
- Poisson Regression with Offset
- Negative Binomial Regression
- Treatment of data with Excessive Zeros
- Zero-inflated Poisson
- Zero-inflated Negative Binomial
- Hurdle Model
Support Vector Machines / Large-Margin / Max-Margin Classifier
- Hyperplanes
- Best Fit "boundary"
- Linear Support Vector Machine using Maximum Margin
- SVM for Noisy Data
- Non- Linear Space Classification
- Non-Linear Kernel Tricks
- Linear Kernel
- Polynomial
- Sigmoid
- Gaussian RBF
- SVM for Multi-Class Classification
- One vs. All
- One vs. One
- Directed Acyclic Graph (DAG) SVM
Kaplan Meier method and life tables are used to estimate the time before the event occurs. Survival analysis is about analyzing the duration of time before the event. Real-time applications of survival analysis in customer churn, medical sciences, and other sectors are discussed as part of this module. Learn how survival analysis techniques can be used to understand the effect of the features on the event using the Kaplan-Meier survival plot.
- Examples of Survival Analysis
- Time to event
- Censoring
- Survival, Hazard, and Cumulative Hazard Functions
- Introduction to Parametric and non-parametric functions
Decision Tree models are some of the most powerful classifier algorithms based on classification rules. In this tutorial, you will learn about deriving the rules for classifying the dependent variable by constructing the best tree using statistical measures to capture the information from each of the attributes.
- Elements of classification tree - Root node, Child Node, Leaf Node, etc.
- Greedy algorithm
- Measure of Entropy
- Attribute selection using Information gain
- Decision Tree C5.0 and understanding various arguments
- Checking for Underfitting and Overfitting in Decision Tree
- Pruning – Pre and Post Prune techniques
- Generalization and Regulation Techniques to avoid overfitting in Decision Tree
- Random Forest and understanding various arguments
- Checking for Underfitting and Overfitting in Random Forest
- Generalization and Regulation Techniques to avoid overfitting in Random Forest
- Check out the Decision Tree Questions here.
Learn about improving the reliability and accuracy of decision tree models using ensemble techniques. Bagging and Boosting are the go-to techniques in ensemble techniques. The parallel and sequential approaches taken in Bagging and Boosting methods are discussed in this module. Random forest is yet another ensemble technique constructed using multiple Decision trees and the outcome is drawn from the aggregating the results obtained from these combinations of trees. The Boosting algorithms AdaBoost and Extreme Gradient Boosting are discussed as part of this continuation module. You will also learn about stacking methods. Learn about these algorithms which are providing unprecedented accuracy and helping many aspiring data scientists win first place in various competitions such as Kaggle, CrowdAnalytix, etc.
- Overfitting
- Underfitting
- Voting
- Stacking
- Bagging
- Random Forest
- Boosting
- AdaBoost / Adaptive Boosting Algorithm
- Checking for Underfitting and Overfitting in AdaBoost
- Generalization and Regulation Techniques to avoid overfitting in AdaBoost
- Gradient Boosting Algorithm
- Checking for Underfitting and Overfitting in Gradient Boosting
- Generalization and Regulation Techniques to avoid overfitting in Gradient Boosting
- Extreme Gradient Boosting (XGB) Algorithm
- Checking for Underfitting and Overfitting in XGB
- Generalization and Regulation Techniques to avoid overfitting in XGB
- Check out the Ensemble Techniques Interview Questions here.
Time series analysis is performed on the data which is collected with respect to time. The response variable is affected by time. Understand the time series components, Level, Trend, Seasonality, Noise, and methods to identify them in a time series data. The different forecasting methods available to handle the estimation of the response variable based on the condition of whether the past is equal to the future or not will be introduced in this module. In this first module of forecasting, you will learn the application of Model-based forecasting techniques.
- Introduction to time series data
- Steps to forecasting
- Components to time series data
- Scatter plot and Time Plot
- Lag Plot
- ACF - Auto-Correlation Function / Correlogram
- Visualization principles
- Naïve forecast methods
- Errors in the forecast and it metrics - ME, MAD, MSE, RMSE, MPE, MAPE
- Model-Based approaches
- Linear Model
- Exponential Model
- Quadratic Model
- Additive Seasonality
- Multiplicative Seasonality
- Model-Based approaches Continued
- AR (Auto-Regressive) model for errors
- Random walk
- Check out the Time Series Interview Questions here.
In this continuation module of forecasting learn about data-driven forecasting techniques. Learn about ARMA and ARIMA models which combine model-based and data-driven techniques. Understand the smoothing techniques and variations of these techniques. Get introduced to the concept of de-trending and de-seasonalize the data to make it stationary. You will learn about seasonal index calculations which are used to re-seasonalize the result obtained by smoothing models.
- ARMA (Auto-Regressive Moving Average), Order p and q
- ARIMA (Auto-Regressive Integrated Moving Average), Order p, d, and q
- ARIMA, ARIMAX, SARIMAX
- AutoTS, AutoARIMA
- A data-driven approach to forecasting
- Smoothing techniques
- Moving Average
- Exponential Smoothing
- Holt's / Double Exponential Smoothing
- Winters / Holt-Winters
- De-seasoning and de-trending
- Seasonal Indexes
- RNN, Bidirectional RNN, Deep Bidirectional RNN
- Transformers for Forecasting
- N-BEATS, N-BEATSx
- N-HiTS
- TFT - Temporal Fusion Transformer
The Perceptron Algorithm is defined based on a biological brain model. You will talk about the parameters used in the perceptron algorithm which is the foundation of developing much complex neural network models for AI applications. Understand the application of perceptron algorithms to classify binary data in a linearly separable scenario.
- Neurons of a Biological Brain
- Artificial Neuron
- Perceptron
- Perceptron Algorithm
- Use case to classify a linearly separable data
- Multilayer Perceptron to handle non-linear data
Neural Network is a black box technique used for deep learning models. Learn the logic of training and weights calculations using various parameters and their tuning. Understand the activation function and integration functions used in developing a Artificial Neural Network.
- Integration functions
- Activation functions
- Weights
- Bias
- Learning Rate (eta) - Shrinking Learning Rate, Decay Parameters
- Error functions - Entropy, Binary Cross Entropy, Categorical Cross Entropy, KL Divergence, etc.
- Artificial Neural Networks
- ANN Structure
- Error Surface
- Gradient Descent Algorithm
- Backward Propagation
- Network Topology
- Principles of Gradient Descent (Manual Calculation)
- Learning Rate (eta)
- Batch Gradient Descent
- Stochastic Gradient Descent
- Minibatch Stochastic Gradient Descent
- Optimization Methods: Adagrad, Adadelta, RMSprop, Adam
- Convolution Neural Network (CNN)
- ImageNet Challenge – Winning Architectures
- Parameter Explosion with MLPs
- Convolution Networks
- Recurrent Neural Network
- Language Models
- Traditional Language Model
- Disadvantages of MLP
- Back Propagation Through Time
- Long Short-Term Memory (LSTM)
- Gated Recurrent Network (GRU)
- Sequence 2 Sequence Models
- Transformers
- Generative AI
- ChatGPT
- DALL-E-2
- Mid Journey
- Crayon
- What Is Prompt Engineering?
- Understanding Prompts: Inputs, Outputs, and Parameters
- Crafting Simple Prompts: Techniques and Best Practices
- Evaluating and Refining Prompts: An Iterative Process
- Role Prompting and Nested Prompts
- Chain-of-Thought Prompting
- Multilingual and Multimodal Prompt Engineering
- Generating Ideas Using "Chaos Prompting"
- Using Prompt Compression
SUNY University Syllabus
- Data Engineering, Machine Learning, & AWS
- Amazon S3 Simple Storage Service
- Data Movement
- Data Pipelines & Workflows
- Jupyter Notebook & Python
- Data Analysis Fundamentals
- Athena, QuickSight, & EMR
- Feature Engineering Overview
- Problem Framing & Algorithm Selection
- Machine Learning in SageMaker
- ML Algorithms in SageMaker
- Advanced SageMaker Functionality
- AI/ML Services
- Problem Formulation & Data Collection
- Data Preparation & SageMaker Security
- Model Training & Evaluation
- AI Services & SageMaker Applications
- Machine Learning
- Machine Learning Services
- Machine Learning Regression Models
- Machine Learning Classification Models
- Machine Learning Clustering Models
- Project Jupyter & Notebooks
- Azure Machine Learning Workspaces
- Azure Data Platform Services
- Azure Storage Accounts
- Storage Strategy
- Azure Data Factory
- Non-relational Data Stores
- Machine Learning Data Stores & Compute
- Machine Learning Orchestration & Deployment
- Model Features & Differential Privacy
- Machine Learning Model Monitoring
- Azure Data Storage Monitoring
- Data Process Monitoring
- Data Solution Optimization
- High Availability & Disaster Recovery
- Certificate Course in Data Science by SUNY
Data Science Training in Bangalore Panel of Coaches
Alumni Speak
About Data Science Classes in Bangalore
The economy is driven by Data. Every industry is harnessing the power of data to succeed in business. Realizing the importance of data, data science has emerged as the number one career in the market. The U.S. Bureau of Labor Statistics suggests that data science skills will drive a hike of around 28% in employment through 2026. There is a huge skill gap in the market and demand for skilled data scientists and related professionals are high.
A Data Scientist analyzes, collects, and interprets large volumes of data using mathematics, statistics, and programming knowledge. Data Scientists can help an organization in designing strategies and business plans that with processing data available to them.
Certified Data Science Course in Bangalore using Python
- Data Science is a booming career and the 360DigiTMG Certified Data Science Program in association with Future Skills Prime accredited by NASSCOM, and approved by the Government of India is your right choice to grow in the career.
- The course curriculum is superior with a focus on Hypothesis Testing and the "4" must-know hypothesis tests.
- Data Mining Unsupervised Learning, Recommendation Engines, Network Analytics with various Machine Learning algorithms, Text Mining, Natural Language Processing, Naive Bayes, Perceptron, and Multilayer Perceptron are dealt with in detail in the course.
- Python modules included in the course add additional value and aid you in scoring the best job in the market.
Highlights of Data Science Training in Bangalore
- Accredited by NASSCOM, Approved by the Government of India
- 184 Hours of Intensive Classroom & Online Sessions
- 2 Capstone Live Projects
- Job Placement Assistance
- Enroll and avail of Government of India (GOI) Incentives after successfully clearing the mandatory Future Skills Prime Assessment
- Learners will get access to multiple resources like NASSCOM Career Fair, NASSCOM industry events, Bootcamps, Career guidance sessions, etc.
- Learners will be eligible to apply for jobs and get job placement assistance through the Talent Connect Portal of Future Skills Prime.
Professional Course in Data Science & AI in Bangalore with Placement Guarantee
- The certification program in Data Science and AI is a unique program that firmly reinforces the fundamentals with concepts like deployment of Python programming, Data Mining, and Machine Learning dealt with in detail.
- The use of NLP libraries and OpenCV to code Machine Learning algorithms are also included in the course.
- This course is a comprehensive package for all IT enthusiasts who wish to design and develop AI applications in their field of study.
- The professionals in data science and Artificial Intelligence will have the liberty to work in the areas of their interest.
- The main areas where Data Science and Artificial Intelligence professionals are in demand are Medicine, Space, Robotics, Automation, Marketing, Information management, Military activities, and many more.
Highlights of Professional Data Science & AI Training in Bangalore
- Get Trained by Trainers from ISB, IIT & IIM
- 300 Hours of Intensive Classroom & Online Sessions
- 300+ Hours of Practical Assignments
- 2+2 Capstone Live Projects
Practical Data Science Course with 100% Job Placement in Bangalore
- Master the Practical Data Science Program with 100% Job Placement* is specifically designed keeping in mind the technological trends in the market and to give professionals an advantage and a chance to grow in their career hassle-free.
- The six-month course comprises 600+ hours of training and 600+ hours of practice sessions.
Highlights Practical Data Science Training with 100% Job Placement in Bangalore
- Get Trained by Trainers from ISB, IIT & IIM
- 500+ Hours of Intensive Classroom & Online Sessions
- 300+ Hours of Practical Assignments
- Learn to Ingest Data from different sources (SQL, NoSQL, Flat files, etc.)
- 30+ Hours of detailed Data Preprocessing
- 21+ Capstone Live Projects
360DigiTMG Offers Best Data Science Course in Bangalore
- With the Certification in Data Science, master all the key tools and techniques in Data Science and pick up domain-specific skills to add more value to your profile.
- In this data-driven environment certification in Data Science prepares you for the surging demand for Big Data skills and technology in all the leading industries.
- There is a huge career prospect available in the field of data science and this Data Science Certification Program is one of the most comprehensive Data Science courses in the industry today.
- This course in India is specially designed to suit both data professionals and beginners who want to make a career in this fast-growing profession.
Highlights of Best Data Science Training in Bangalore
- Get Trained by Trainers from ISB, IIT & IIM
- 40 Hours of Intensive Classroom & Online Sessions
- 40+ Hours of Practical Assignments
- 1 Capstone Live Project
- Authorized by National Educational Alliance for Technology - NEAT
Domain Specific Data Science Courses in Bangalore
Data Scientist in Supply Chain Management: Managing a supply chain in manufacturing can be very complex and unpredictable. Some factors that influence supply include the cost of production, technology, transport conditions, government policies, and the price of inputs. Data scientists in supply chain management are expected to analyze and predict patterns of inputs and outputs to minimize risk and ensure a smooth-running system. Big data in the supply chain helps manufacturers to improve efficiency and make timely decisions. Data scientists in the supply chain industry are responsible for analyzing data to support predictive analysis, make accurate forecasts, and inform risk management strategies.
Data Science with NoSQL: Data Science is the process of analyzing and interpreting complex data sets to extract meaningful insights. NoSQL databases can handle multiple data types, including text, images, and audio, and are particularly useful for processing real-time data from social media and other sources. This makes them ideal for applications such as recommendation systems, customer behaviour analysis, and sentiment analysis. Data Scientists use NoSQL databases to perform big data analysis, machine learning, and data visualization. They can use a variety of tools to extract data from NoSQL databases, including Hadoop, Spark, and MongoDB. NoSQL databases also offer a higher level of scalability and performance compared to traditional relational databases.
Data Science in Customer Analytics: Data science plays a crucial role in customer analytics, as it allows organizations to gather, process, and analyze large amounts of customer data to gain insights into customer behaviour and preferences. The goal of customer analytics is to use data to better understand customer needs and preferences and make informed decisions that improve customer experience and drive business growth. Customer segmentation, Predictive modelling, customer lifetime value analysis, and customer feedback analysis are some examples of how data science can be used for customer analytics.
Data Science in Social Media & Web Analytics: Data Science in Social Media and Web Analytics has become an increasingly important field as social media usage continues to rise. Social media platforms such as Facebook, Twitter, and Instagram generate a vast amount of data which, when analyzed, can be used by businesses to understand marketing strategies, product development, and customer service efforts. Another important aspect of data science in social media and web analytics is its role in understanding customer sentiment. By analyzing the language used in social media posts, comments, and reviews, businesses can gain a deeper understanding of how customers feel about their brand, products, and services. This information can be used to make changes that improve customer satisfaction and loyalty.
Data Science in Retail Analytics: Data science has had a major impact on the Retail industry. Data science can be used to improve customer experience, optimize pricing, assess and improve marketing activities, and more. Retailers can use data science to identify different customer segments based on their shopping behaviour, preferences, and spending patterns via customer segmentation. They can identify opportunities, understand churn, and anticipate demand using predictive analysis. With personalization, they can personalize the shopping experience for customers. This includes targeted product recommendations, personalized emails, and customized discounts. With the help of optimising inventory, they can help retailers optimize their inventory levels by analyzing customer demand and forecasting future trends. This helps retailers minimize losses due to overstocking or stockouts.
Data Science in Marketing: The role of data science in marketing is to unlock the value of big data by turning it into actionable insights. Data scientists use a variety of techniques, including machine learning, predictive analytics, and data visualization, to analyze large and complex data sets and extract meaningful insights. These insights can then be used to inform marketing strategies, target the right customers, and measure the effectiveness of marketing campaigns. Marketers are now able to collect and analyze vast amounts of data from a variety of sources, including social media, web analytics, and customer relationship management (CRM) systems, to gain valuable insights into customer behavior and preferences. Data science has become a critical tool for marketers as they strive to deliver personalized, data-driven marketing campaigns that engage customers and drive business success.
Data Science in Life Sciences and Healthcare: Data science has a significant impact on the field of life sciences and healthcare, offering new opportunities to improve patient outcomes, speed up drug development, and make more informed decisions about patient care. Thanks to Data Science, doctors can now make informed decisions about patient care and treatment by using predictive modeling to learn the progression of the disease. They can also optimize clinical trial designs, reduce drug side effects and identify potential drug targets to improve the life of the patient.
Data Science in HR: Data science in HR can be used to analyze employee retention rates by understanding the factors that contribute to employee retention. When it comes to recruitment data science can be used to enhance the recruitment process. It can help HR professionals identify and attract the best-suited candidates for a particular position by analyzing past recruitment data, resumes, and candidate profiles. Regarding Performance Management, data science can help HR teams measure and monitor employee performance. It can be used to identify areas of improvement, set realistic goals and objectives, and track employee progress. In an aspect of Diversity and Inclusion data science can be used to understand the composition of the workforce and identify any potential gaps in diversity and inclusion.
Data Science in Finance: The finance sector is the backbone of the world economy. And they know the importance of Big Data for making profitable decisions. Regularly, this sector collects a sheer amount of big data. And the challenge lies in gaining insights from this unstructured data. This data contains a lot of noise and lacks a coherent model. Data Scientist uses advanced ML techniques to create insights for customer service solutions. And is further utilized by companies to generate profits and derive financial conclusions. Financial companies use data science to detect fraud and find abnormalities in transactions.
Data Science in Cyber Security Analytics: Data Science plays a crucial role in the field of Cyber Security by providing techniques to detect, prevent and respond to cyber threats. Using techniques like machine learning, big data analytics, and data visualization, data scientists can analyze vast amounts of data from various sources such as network logs, intrusion detection systems, and security information and event management (SIEM) tools to identify patterns and anomalies that may indicate a potential security breach.
Data Scientist in Construction Analytics: Data Science is revolutionizing the construction and architecture industry by providing valuable insights into project performance and identifying areas of improvement. By collecting and analyzing data from various sources such as project schedules, cost data, and resource utilization, construction professionals can make informed decisions that improve project efficiency, reduce waste, and ensure compliance with industry standards. It helps architects to make informed design decisions and ensure that their designs are optimized for performance and sustainability.
Data Science in Aviation Analytics: Data science in the aviation domain has become an increasingly important tool to help airlines and other aviation industry stakeholders better understand their customers and operations, and make more informed decisions. Data science techniques allow airlines to analyze customer data, flight information, and other metrics to gain insights into pricing, customer segmentation, and marketing strategies. Airlines can use predictive analytics to better
Data Science Career Trends in Bangalore
Data Science is a dynamic and significantly evolving field as new technologies are emerging and expanding. The demand for Data Scientists is at an all-time high in India, and it is expected that the Data Science industry will touch nearly USD 230.80 billion by 2026. With Bangalore being the central hub of the IT sector, it's needless to say that data science will be one of the most high-paying jobs in India.
A staggering career awaits everyone who has completed this course. It is an ever-evolving field; therefore, data scientists must keep learning new skills and unlearn redundant skills to always be on track. There are countless resources and courses available to students and professionals who want to learn about this vast field, but knowing where to start can get overwhelming. Choose the Data science course from 360DigiTMG to set up a solid and robust foundation in Data Science.
Some of the top sightseeing places to see in Bangalore, Lalbagh Botanical Garden, ISKCON Shri Radhekrishna Temple, Bengaluru Palace, Cubbon Park, Vidhana Soudha, Bannerghatta biological park and many others.
Why Choose 360DigiTMG for Data Science Training Institute in Bangalore?
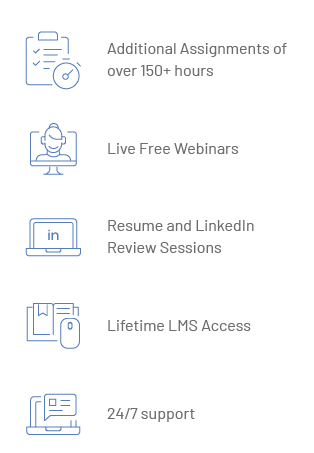
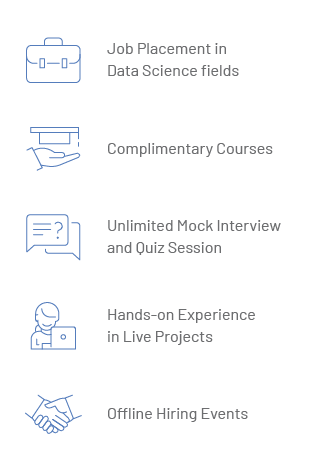
Call us Today!
Recommended Programmes
Data Scientist Course

2064 Learners
Data Engineering Course

3021 Learners
Data Analytics Course

2915 Learners
Our Alumni Work At
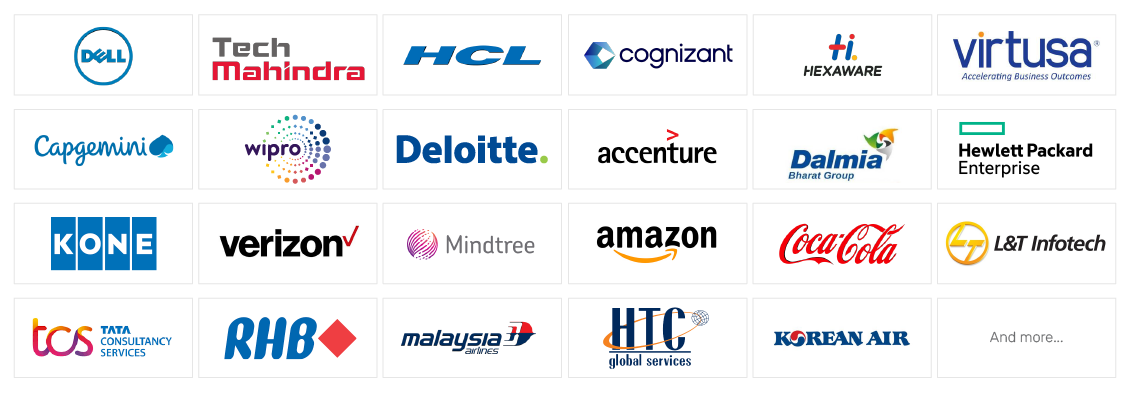
"AI to contribute $16.1 trillion to the global economy by 2030. With 133 million more engaging, less repetitive jobs AI to change the workforce." - (Source). Data Science with Artificial Intelligence (AI) is a revolution in the business industry.. AI is potentially being adopted in automating many jobs leading to higher productivity, less cost, and extensible solutions. It is reported by PWC in a publication that about 50% of human jobs will be taken away by the AI in the next 5 years.
There is already a huge demand for AI specialists and this demand will be exponentially growing in the future. In the past few years, careers in AI have boosted concerning the demands of industries that are digitally transformed. The report of 2018 states that the requirements for AI skills have drastically doubled in the last three years, with job openings in the domain up to 119%.
Data Science Courses in Bangalore FAQs
The best skill for Data Science is a combination of strong statistical knowledge and programming proficiency, particularly in languages like Python or R. This blend allows professionals to effectively manipulate data, apply statistical methods, build models, and derive actionable insights to solve complex problems across diverse domains.
Best stream for becoming a Data Scientist, as the field is highly interdisciplinary. However, streams such as computer science, statistics, mathematics and engineering are commonly pursued due to their focus on analytical thinking, programming skills, and quantitative analysis, all crucial for Data Science.
It's highly likely that Data Science will continue to evolve and remain relevant in the next decade. With the exponential growth of data and its importance across various industries, the demand for data-driven insights and solutions is expected to increase, ensuring the continued presence and evolution of Data Science.
Yes, typically it takes around 6 to 12 months to develop a strong foundation and proficiency in Data Science. While it's possible to learn the basics in three months with dedicated effort, achieving mastery often requires additional time for deeper understanding, practical application, and gaining experience with real-world data sets.
Absolutely! Data science is a multidisciplinary field, and individuals from various backgrounds, including non-IT fields, can learn and excel in it. While a background in IT or computer science may provide a head start, it's not a prerequisite. Many successful data scientists come from diverse academic backgrounds such as mathematics, statistics, engineering, economics, social sciences, and even humanities. What matters most is a strong willingness to learn, curiosity, and dedication to acquiring the necessary skills and knowledge in Data Science.
Yes, beginners can readily explore the field of Data Science. Internet offers lots of free learning materials by organizations such as 360DigiTMG that consists of tutorials, courses and online community focused on inexperienced students. Through teaching basic programming, statistics and data manipulation concepts at the top, novices can acquire a fundamental education that will develop into advanced data analysis skills over time.
There is no set minimum age to begin Data Science studies. People in any fields who want to have an in-depth understanding of this discipline and no matter their age can start with learning its concepts. On the other hand, due to the complicacy of some topics and the prerequisite of the necessary knowledge, it is more popular for individuals of those ages to enrol in formal education or the individual learn the knowledge by themselves in Data Science.
Yes, Data Science can be an excellent career choice for graduates. A wide range of Data Science roles, from entry-level positions to internships, is available for new graduates who may utilize them to gain experience and move forward in their career. By mastering the right skillsets and acquiring knowledge, freshers may have an easier time getting into lucrative jobs in data analysis, machine learning, data engineering and related fields.
The salary of a Data Scientist can go up depending on factors like experience, location, industry, and the company itself. However, according to my last update, the average base salary for a Data Scientist in India is approximately between 6 to 12 lakhs (INR) per annum. While such figure can vary greatly on the factors indicated above. It is recommended to browse through recent ads and other sources of salary information to get the most accurate picture.
Yes, certainly, Data Science is one the most in-demand occupations at Bangalore, India. Bangalore is now considered as the prominent technology cities where many companies and websites which data analytics is involved, often makes decisions. Concurrently, that is why there has been a steep demand for highly skilled data scientists in Bangalore not just in IT but also banking, healthcare, e-commerce, etc.
Data science focuses mainly on the extraction of knowledge and findings from data by means of complex methods, such as statistical analysis, machine learning, data mining, and data visualisation. It is the set of techniques that combines quantitative and qualitative methods, along with computer science, and domain expertise so that we can extract valuable insights and make wise decisions.
Data science is the cornerstone of technology, healthcare, finance, retail, marketing, manufacturing, and telecommunications. It is the innovation accelerator that covers such topics as improved search algorithms, medical research, fraud detection, demand forecasting, innovative customers analysis, predictive maintenance, and internet improvement. These applications serve as tools for decision-making, with numerous businesses relying on them to grow and for insights to be garnered from different areas of commerce.
Data science can be a tough subject as it is multi-disciplinary and thus one needs to be proficient in statistics, programming and domain knowledge to excel in it. On the other hand one will come across only very many strangers with an almost absolute state online tools like e-courses , mailing list and community structures.
Individuals from diverse educational backgrounds can pursue Data Science. Eligibility typically includes having a strong foundation in mathematics, statistics, and programming. Common backgrounds include computer science, mathematics, statistics, engineering, economics, and other related fields. However, with dedication and willingness to learn, individuals from any background can transition into Data Science.
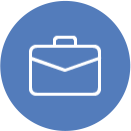
Field of Data Science Jobs in Bangalore
Bangalore presents more than 31,000 openings for Data Scientists freshers making up 21% of India's data science job market.Top recruiters like Tech Mahindra, TCS, Genpact, Wipro, and HCL Infosystems actively seek talent, presenting ample career growth opportunities in evolving roles.
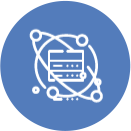
Projects for Data Science in Bangalore
The Indian government has launched numerous data science projects encompassing fraud detection for financial institutions, as well as fields such as agriculture, electricity, healthcare, education, road traffic safety, and air pollution.
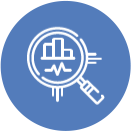
Role Of Open Source Tools In Data Science
Python stands out for its user-friendly nature and ease of maintenance, making it invaluable to developers in the field.Its extended library makes it possible to stretch the applications of Python from Big Data Analytics to Machine Learning.
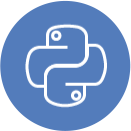
Modes of Training for Data Science
Data science course in Bangalore is meticulously crafted to cater to the requirements of both students and working professionals.We at 360DigiTMG give our students the option of both classroom and online learning. We also support e-learning as part of our curriculum.
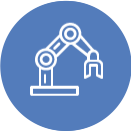
Industry Applications of Data Science
Data Science is used for securities fraud early warning, card fraud detection systems, demand enterprise risk management, analysis of healthcare information, seismic interpretation, reservoir characterization, energy exploration, traffic control and route planning.
Talk to your program advisors today!
Get your profile reviewed
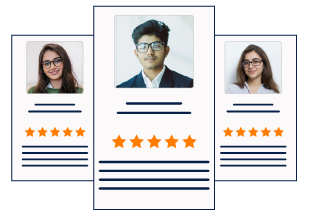
360DigiTMG - Data Science, Data Scientist Course Training in Bangalore
No 23, 2nd Floor, 9th Main Rd, 22nd Cross Rd,7th Sector, HSR Layout, Bangalore, Karnataka-560102.
Data Science Certification Course Training Locations Nearby Bangalore - Google Reviews Data Science Classes in Bangalore, Data Science Course in Hebbal, Best Data Science Institute, Data Science Institute in Banashankari, Data Science Coaching in Bangalore with Placements, Best Data Science Course in Jayanagar, Master Data Science with our specialized training and certification program in Bangalore. Dive into data analysis for efficient model deployment and monitoring. Join us in Bangalore to excel in this dynamic field and propel your career forward.