Sent Successfully.
Home / Blog / Interview Questions on Data Engineering / Top 35 Data Architect Interview Questions
Top 35 Data Architect Interview Questions
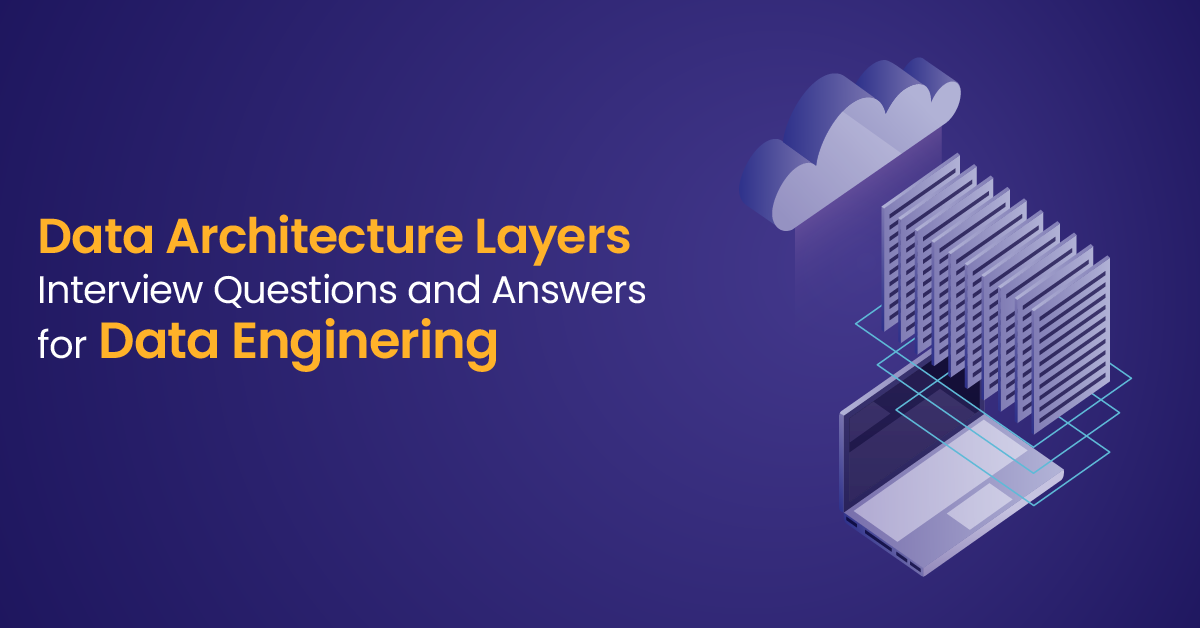
Table of Content
- What is Data Architecture, and why is it important in data engineering?
- Describe the layers in a typical Data Architecture.
- What role do data lakes play in Data Architecture?
- How does a data warehouse fit into Data Architecture?
- What is the significance of data modeling in Data Architecture?
- Explain the concept of ETL in Data Architecture.
- How do data ingestion layers function in Data Architecture?
- Discuss the role of data pipelines in Data Architecture.
- What is the purpose of data orchestration in Data Architecture?
- Describe real-time data processing in the context of Data Architecture.
- How are APIs used in Data Architecture?
- What are the considerations for data security in Data Architecture?
- How does cloud computing impact Data Architecture?
- Explain the concept of data federation in Data Architecture.
- Discuss the importance of metadata in Data Architecture.
- What is data governance, and how is it integrated into Data Architecture?
- How do Master Data Management (MDM) systems fit into Data Architecture?
- What are the challenges of integrating Big Data into Data Architecture?
- Describe the role of data virtualization in Data Architecture.
- How is business intelligence (BI) incorporated into Data Architecture?
- What is the importance of scalability in Data Architecture?
- Explain the role of NoSQL databases in Data Architecture.
- Discuss the concept of data lakes and data warehouses convergence.
- What are data marts, and how do they fit into Data Architecture?
- How do event-driven architectures influence Data Architecture?
- What is the impact of AI and machine learning on Data Architecture?
- How do you ensure data quality in Data Architecture?
- Explain the importance of disaster recovery in Data Architecture.
- What are the best practices for data backup in Data Architecture?
- How do you address data latency in Data Architecture?
- What is the role of data compression in Data Architecture?
- Discuss the integration of external data sources in Data Architecture.
- How does data de-duplication work in Data Architecture?
- Explain the role of streaming data in Data Architecture.
- What are the trends shaping the future of Data Architecture?
-
What is Data Architecture, and why is it important in data engineering?
Data Architecture refers to the models, policies, rules, and standards governing the collection, storage, arrangement, and use of data in organizations. It's crucial for ensuring data is managed effectively and aligns with business goals.
-
Describe the layers in a typical Data Architecture.
Typical layers include data sources, data ingestion, data storage, data processing, data orchestration, data services/APIs, and data consumption.
-
What role do data lakes play in Data Architecture?
Data lakes store vast amounts of raw data in its native format. They are a flexible layer in Data Architecture, allowing for storage of structured, semi-structured, and unstructured data.
-
How does a data warehouse fit into Data Architecture?
A central store for integrated data of several sources is called a data warehouse. It functions is a structured storage layer in data architecture that is enhanced for reporting and analysis.
-
What is the significance of data modeling in Data Architecture?
Data modeling is crucial for designing the data structures and schema within databases and warehouses. It ensures that data is organized logically and efficiently for access and analysis.
-
Explain the concept of ETL in Data Architecture.
The process of obtaining data from several sources, changing it into an appropriate format, and then putting it into a data warehouse and other storage system is known as ETL (Extract, Transform, Load).
-
How do data ingestion layers function in Data Architecture?
The data ingestion layer is responsible for importing data from various sources into the system. It handles the initial collection, extraction, and transportation of data.
-
Discuss the role of data pipelines in Data Architecture.
Data pipelines, which usually involve operations including ingestion, transformation, and loading, automate the movement of data from its source to its destination. They are essential to the effective processing and transportation of data.
-
What is the purpose of data orchestration in Data Architecture?
Data orchestration coordinates various data processing tasks, ensuring they occur in the correct order and managing dependencies between different data flows.
-
Describe real-time data processing in the context of Data Architecture.
Real-time data processing involves the continuous input, processing, and output of data. It's crucial for scenarios where immediate processing and insights are required.
-
How are APIs used in Data Architecture?
APIs (Application Programming Interfaces) are used to enable integration between different systems and layers in Data Architecture, allowing different components to communicate and exchange data.
-
What are the considerations for data security in Data Architecture?
Data security considerations include encryption, access controls, compliance with data protection regulations, and implementing secure data transmission and storage practices.
-
How does cloud computing impact Data Architecture?
Cloud computing offers scalable, on-demand resources and services for data storage, processing, and analysis, allowing for more flexible and cost-effective Data Architectures.
-
Explain the concept of data federation in Data Architecture.
Data federation is the process of aggregating data from disparate sources to create a single, virtual view. It allows for unified data access without physically integrating data.
-
Discuss the importance of metadata in Data Architecture.
Metadata provides information about data, like its source, format, and structure. In Data Architecture, it's vital for understanding, managing, and using data effectively.
-
What is data governance, and how is it integrated into Data Architecture?
Data governance involves managing the availability, integrity, and security of the data. It's integrated into Data Architecture through policies, standards, and procedures that guide data management.
-
How do Master Data Management (MDM) systems fit into Data Architecture?
MDM systems ensure the uniformity, accuracy, stewardship, and consistency of an enterprise's official shared master data. They integrate into Data Architecture as a central source of master data.
-
What are the challenges of integrating Big Data into Data Architecture?
Challenges include handling the volume, variety, and velocity of Big Data, ensuring data quality, integrating diverse data sources, and scaling data infrastructure.
-
Describe the role of data virtualization in Data Architecture.
Data virtualization creates a virtual layer that provides unified data access and retrieval across different sources, without needing to move or replicate data.
-
How is business intelligence (BI) incorporated into Data Architecture?
BI tools and systems are incorporated into Data Architecture for analyzing data, generating reports, and supporting decision-making processes based on data stored in warehouses or lakes.
-
What is the importance of scalability in Data Architecture?
Scalability ensures that the data infrastructure can handle growth in data volume, velocity, and variety, maintaining performance and avoiding system overloads.
-
Explain the role of NoSQL databases in Data Architecture.
NoSQL databases handle a variety of data formats and are designed for high scalability and flexibility. They are used in Data Architectures for unstructured or semi-structured data.
-
Discuss the concept of data lakes and data warehouses convergence.
The convergence of data lakes and warehouses combines the flexible storage and processing of a data lake with the structured environment of a data warehouse, enhancing analytical capabilities.
-
What are data marts, and how do they fit into Data Architecture?
Data marts are subsets of data warehouses tailored to specific business lines or departments. They fit into Data Architecture as focused areas for specific analytics needs.
-
How do event-driven architectures influence Data Architecture?
Event-driven architectures trigger actions and data processing in response to events. They influence Data Architecture by introducing real-time data processing and responsive data flows.
-
What is the impact of AI and machine learning on Data Architecture?
AI and machine learning require Data Architectures to support large datasets, complex analytics, and real-time processing, influencing design and technology choices.
-
How do you ensure data quality in Data Architecture?
Data quality is maintained through validation rules, consistency checks, data profiling and cleansing, and ensuring accurate data transformations and mappings.
-
Explain the importance of disaster recovery in Data Architecture.
Disaster recovery plans are essential to ensure data availability and continuity of operations in case of system failures, data corruption, or other disasters.
-
What are the best practices for data backup in Data Architecture?
Best practices include regular backups, off-site storage, using reliable backup systems, and testing recovery processes to ensure data can be restored effectively.
-
How do you address data latency in Data Architecture?
Addressing data latency involves optimizing data processing and transfer processes, using faster storage solutions, and implementing efficient data caching strategies.
-
What is the role of data compression in Data Architecture?
Data compression reduces the size of data, making storage more efficient and improving transfer speeds. It's important for managing large volumes of data.
-
Discuss the integration of external data sources in Data Architecture.
Integration involves connecting and harmonizing external data with internal systems, often using APIs, ETL processes, or data federation techniques.
-
How does data de-duplication work in Data Architecture?
Data de-duplication involves identifying and removing duplicate records, improving data quality and reducing storage requirements.
-
Explain the role of streaming data in Data Architecture.
Streaming data involves continuous data flow and processing, commonly used in real-time analytics. It's integrated into Data Architecture to enable immediate insights and actions.
-
What are the trends shaping the future of Data Architecture?
Trends include the increasing adoption of cloud services, the growth of edge computing, advancements in AI and machine learning, and the increasing importance of data privacy and security.
Navigate to Address
360DigiTMG - Data Analytics, Data Science Course Training in Chennai
1st Floor, Santi Ram Centre, Tirumurthy Nagar, Opposite to Indian Oil Bhavan, Nungambakkam, Chennai - 600006
1800-212-654-321
Get Direction: Data Science Course