Sent Successfully.
Home / Blog / Big Data & Analytics / The Future of Data Analytics : Unveiling Tomorrow
The Future of Data Analytics : Unveiling Tomorrow
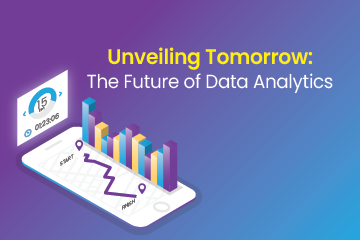
Table of Content
- What is Data Analytics?
- What is Artificial Intelligence?
- Data Analytics and Artificial Intelligence: The Perfect Union
- Data Visualization in the Future: Beyond Charts and Graphs
- The Rise of Explainable AI in Data Analytics
- Data Privacy and Ethics in the Future of Analytics
- Data Analytics for Sustainability: Driving Positive Change
- The Future of Data Analytics: Skills and Careers
In today's data-driven world, the role of data analytics has become increasingly vital across industries. As organizations gather massive amounts of data from various sources, the need to extract meaningful insights and make informed decisions has never been more crucial. However, the landscape of data analytics is continually evolving, driven by advancements in technology, the exponential growth of data, and the quest for deeper understanding. As we stand on the cusp of a new era, it is essential to explore the exciting possibilities and potential that lie ahead. In this blog, we embark on a journey to unveil tomorrow, delving into the future of data analytics and the transformative impact it is set to bring.
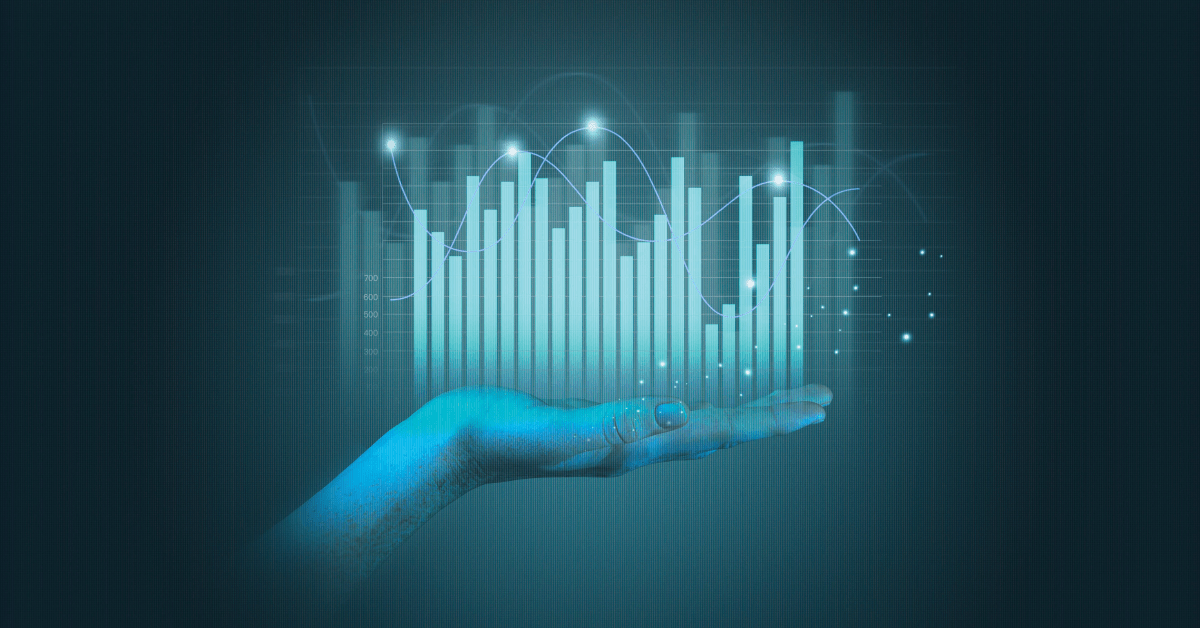
The convergence of data analytics and artificial intelligence (AI) stands at the forefront of this evolution. AI techniques, such as machine learning and deep learning, have the power to revolutionize data analytics by enabling faster, more accurate predictions and automated decision-making. The synergy between AI and data analytics opens up a world of possibilities, empowering organizations to extract insights from vast amounts of data and uncover hidden patterns that were previously beyond human comprehension.
Learn the core concepts of Data Science Course video on YouTube:
What is Data Analytics?
Data analytics is the process of examining and analyzing data to uncover meaningful insights, patterns, and trends. It involves various techniques and methodologies for collecting, transforming, and interpreting data to extract valuable information. Data analytics encompasses tasks such as like the data cleaning, statistical analysis, data modeling, data visualization, and predictive modeling. The goal of data analytics is to make data-driven decisions, improve business performance, identify opportunities, and solve complex problems. By leveraging data analytics, organizations can gain a deeper understanding of their operations, customers, and market dynamics, leading to better strategic planning and informed decision-making.
What is Artificial Intelligence?
Artificial Intelligence (short term AI) refers to simulation of the human intelligence in the machines to perform tasks that are typically require the human intelligence, such as learning, reasoning, problem-solving, and decision-making. AI systems are designed to mimic cognitive functions, including perception, understanding natural language, and adapting to new situations. AI encompasses the various subfields, such as like including machine learning, computer vision, natural language processing, and also robotics. Machine learning, in particular, is a key component of AI that focuses on enabling machines to learn from data and improve their performance without explicit programming. AI technologies have applications across diverse domains, from virtual assistants and autonomous vehicles to healthcare diagnostics and fraud detection.
Data Analytics is a promising career option. Enroll the Data Analytics classes in Chennai offered by 360DigiTMG to become a successful Data Analyst.
Data Analytics and Artificial Intelligence: The Perfect Union
The combination of data analytics and artificial intelligence represents a powerful synergy that amplifies the capabilities of both disciplines. Data analytics provides the foundation by leveraging vast amounts of data to derive meaningful insights and patterns. It encompasses the techniques and tools for data cleaning, transformation, and analysis. On the other hand, artificial intelligence augments data analytics by leveraging advanced algorithms and computational models to automate processes, identify complex patterns, and make predictions.
1. The role of AI in enhancing data analytics capabilities: Artificial intelligence (AI) plays a crucial role in augmenting data analytics capabilities by automating and accelerating various processes. AI algorithms and techniques can handle large volumes of data, identify patterns, and extract meaningful insights at a speed and scale that surpasses human capabilities. With AI, data analytics becomes more efficient and accurate, allowing organizations to gain deeper and more comprehensive insights from their data. AI-powered data analytics tools can automate data preprocessing, perform advanced data analysis, and generate actionable recommendations, enabling businesses to make data-driven decisions with confidence.
2. Machine learning algorithms for predictive analytics: Machine learning algorithms are at the heart of predictive analytics, a branch of data analytics that focuses on forecasting future outcomes based on historical data patterns. By leveraging machine learning techniques, organizations can build predictive models that learn from historical data and make predictions or classifications on new data. These algorithms can identify hidden relationships, detect trends, and forecast future trends and behaviors. Predictive analytics powered by machine learning algorithms has numerous applications across industries, including sales forecasting, customer churn prediction, fraud detection, and demand forecasting.
3. Natural language processing for unstructured data analysis: Unstructured data, such as text documents, social media posts, customer reviews, and emails, presents a significant challenge for traditional data analytics methods. Natural language processing (NLP), a subfield of AI, enables the analysis and interpretation of unstructured data by allowing computers to understand and process human language. NLP techniques can extract valuable information from text data, perform sentiment analysis, identify key topics, and even generate human-like responses. By leveraging NLP in data analytics, organizations can gain insights from unstructured data sources, enabling them to understand customer sentiments, extract market intelligence, and improve decision-making based on textual information.
The integration of AI into data analytics enhances the speed, accuracy, and scalability of analyzing data. AI algorithms can handle large datasets, identify hidden relationships, and generate predictions or recommendations based on historical data. By leveraging AI techniques such as machine learning and natural language processing, data analytics can uncover deeper insights, automate repetitive tasks, and enable predictive analytics.
The perfect union of data analytics and artificial intelligence empowers organizations to unlock the full potential of their data. It enables them to make data-driven decisions with greater confidence, discover new opportunities, optimize operations, and drive innovation. The combination of data analytics and AI opens up possibilities for transformative applications across industries, including personalized marketing, fraud detection, healthcare diagnostics, recommendation systems, and predictive maintenance.
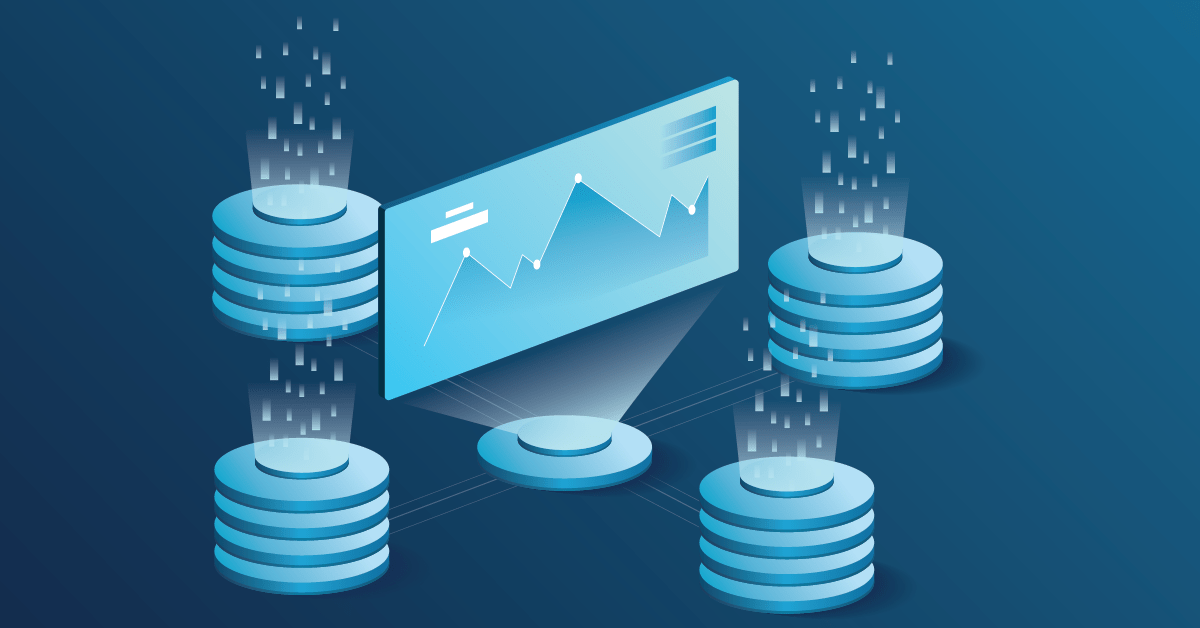
Data Visualization in the Future: Beyond Charts and Graphs
Data visualization has long been a powerful tool for representing data in a visual and accessible manner. However, the future of data visualization holds exciting possibilities that go beyond traditional charts and graphs. With advancements in technology and the increasing complexity of data, data visualization is evolving to provide richer, more immersive, and interactive experiences.
1. Immersive data visualization through virtual and augmented reality: 1.The future of data visualization holds the promise of immersive experiences through virtual reality (VR) and augmented reality (AR) technologies. With VR, users can enter a virtual environment where data visualizations surround them, creating an immersive and interactive experience. They can explore data landscapes, interact with visual elements, and gain a deeper understanding of complex datasets. AR, on the other hand, overlays data visualizations onto the real world, allowing users to see and interact with data in their physical environment. Immersive data visualization opens up new possibilities for data exploration, analysis, and communication, enabling users to gain insights in a more intuitive and engaging manner.
2. Interactive visualizations for enhanced exploration and understanding: In the future, data visualization will become increasingly interactive, enabling users to actively engage with visualizations and explore data from different perspectives. Interactive visualizations empower users to manipulate parameters, filter data, drill down into details, and customize visual representations according to their needs. By providing interactive controls and real-time feedback, users can uncover hidden patterns, identify trends, and gain deeper insights into the data. Interactive data visualization facilitates a more dynamic and exploratory approach to data analysis, enabling users to make discoveries and derive actionable insights.
3. Visual storytelling techniques for impactful data presentations: Visual storytelling techniques will play a pivotal role in the future of data visualization. Instead of simply presenting data, visualizations will be designed to tell compelling stories and convey narratives. Storytelling elements such as narratives, characters, and plotlines will be integrated into data visualizations to create impactful and memorable presentations. By weaving data into a narrative framework, visualizations can evoke emotions, convey complex ideas, and engage audiences on a deeper level. Visual storytelling techniques make data more relatable, accessible, and memorable, allowing stakeholders to understand and connect with data-driven insights in a meaningful way.
360DigiTMG offers the best Data Analytics course in Hyderabad to start a career in Data Analytics. Enroll now!
The Rise of Explainable AI in Data Analytics
The rise of explainable AI in data analytics addresses the growing need for transparency, interpretability, and accountability in AI models. As AI algorithms become more complex and influential in decision-making processes, it is essential to understand how these models arrive at their predictions or recommendations.
Explainable AI (XAI) refers to the methods and techniques that aim to make AI models more transparent and interpretable to humans. Traditional AI models, such as deep neural networks, are often considered "black boxes" because their decision-making processes are not easily understandable. However, in domains where AI is employed, such as finance, healthcare, or legal systems, it is crucial to have insights into the reasoning behind AI-driven insights to ensure fairness, mitigate biases, and establish trust.
1. The need for transparency and interpretability in AI models: As artificial intelligence (AI) models become more complex and powerful, there is a growing need for transparency and interpretability. Traditional AI models, such as deep neural networks, are often referred to as "black boxes" because their decision-making processes are not easily interpretable by humans. However, in many domains, it is crucial to understand how AI models arrive at their predictions or decisions. Transparency and interpretability enable stakeholders to trust AI-driven insights, identify potential biases or errors, and ensure accountability. By shedding light on the inner workings of AI models, organizations can better understand the reasoning behind the results and validate the fairness and validity of their AI-driven analytics.
2. Methods and techniques for explainable AI in data analytics: 2.Explainable AI (XAI) refers to the methods and techniques that aim to make AI models more transparent and interpretable. Various approaches have been developed to achieve explainability in data analytics. One approach is to use simpler, more interpretable models, such as decision trees or rule-based models, in place of complex black box models. Another approach involves generating explanations alongside AI predictions, providing insights into the factors that influenced the model's decision. Techniques like feature importance analysis, model-agnostic methods, and rule extraction algorithms can also be employed to extract explanations from AI models. These methods enable analysts and stakeholders to understand the factors, variables, or rules that contribute to the AI model's predictions, improving transparency and interpretability.
3. Ensuring fairness and accountability in AI-driven insights: Fairness and accountability are critical considerations in AI-driven insights. AI models trained on biased or unrepresentative datasets can perpetuate and amplify existing biases or discrimination. To ensure fairness, it is essential to assess and mitigate biases in data analytics. Techniques such as fairness-aware learning, bias detection, and fairness metrics can be employed to identify and address biases in AI models. Additionally, organizations must establish guidelines, ethical frameworks, and regulatory policies to govern the use of AI in data analytics. This includes establishing accountability for decisions made based on AI-driven insights and ensuring that AI models are transparent, explainable, and subject to scrutiny.
Becoming a Data Analyst is possible now with the 360DigiTMG Data Analytics training in Bangalore. Enroll today.
The rise of explainable AI in data analytics addresses the need for transparency, interpretability, fairness, and accountability in AI models. It emphasizes the importance of understanding how AI models make decisions and enables stakeholders to validate and trust the insights provided. By employing methods and techniques for explainable AI, organizations can enhance the transparency and interpretability of AI models, ensuring fairness and accountability in the deployment of AI-driven insights in various domains.
Data Privacy and Ethics in the Future of Analytics
Data privacy and ethics play a critical role in shaping the future of analytics. As data becomes increasingly abundant and accessible, organizations must prioritize the protection of individuals' privacy rights and ensure ethical practices throughout the entire data analytics process.
1. Privacy challenges in the era of data-driven insights: Privacy challenges in the era of data-driven insights: As data-driven insights become increasingly prevalent in analytics, privacy challenges emerge in relation to the collection, storage, and usage of personal data. With the vast amounts of data being generated, there is a need to ensure that individuals' privacy rights are respected and protected. Challenges include obtaining informed consent for data collection, safeguarding sensitive information, implementing robust security measures, and addressing the risk of data breaches. Organizations must navigate these challenges to strike a balance between deriving valuable insights from data and safeguarding individuals' privacy.
2. Ethical considerations in data collection and usage: The future of analytics brings forth ethical considerations that organizations must address in their data collection and usage practices. Ethical considerations include ensuring data is collected lawfully, respecting individuals' autonomy and consent, maintaining data accuracy and integrity, and preventing unfair discrimination. Organizations must also consider issues such as algorithmic bias, transparency in decision-making processes, and the responsible use of data in sensitive domains like healthcare or finance. Ethical frameworks and guidelines play a crucial role in guiding organizations towards responsible and ethical data collection and usage practices.
3. Regulatory frameworks and best practices for responsible analytics: In response to the evolving data landscape, regulatory frameworks are being established to govern the responsible use of data in analytics. These frameworks include laws and regulations such as the General Data Protection Regulation (GDPR) in Europe or the California Consumer Privacy Act (CCPA) in the United States. These regulations aim to protect individuals' privacy rights, establish guidelines for data collection and usage, and ensure transparency and accountability in analytics practices. Organizations must comply with these regulations, implement privacy-by-design principles, and adopt best practices such as anonymization, data minimization, and secure data storage to ensure responsible analytics.
In summary, as the future of analytics unfolds, data privacy and ethics become paramount considerations. Organizations need to navigate privacy challenges, address ethical considerations in data collection and usage, and adhere to regulatory frameworks. By prioritizing privacy, ethics, and responsible analytics, organizations can build trust with their customers, ensure compliance with regulations, and leverage data insights in an ethical and responsible manner.
Data Analytics for Sustainability: Driving Positive Change
In today's world, the need for sustainable practices and addressing pressing environmental and social challenges has never been more critical. Data analytics, with its ability to analyze vast amounts of information, has emerged as a powerful tool for driving positive change and advancing sustainability efforts. By harnessing the potential of data analytics, organizations can gain valuable insights into sustainability-related issues and make data-driven decisions to address them effectively. Whether it's climate change, resource depletion, waste management, or social inequality, data analytics provides a deeper understanding of these challenges, their root causes, and their impacts.
1. Harnessing data analytics to address environmental and social challenges: Data analytics has the potential to drive positive change by providing valuable insights and solutions to environmental and social challenges. By analyzing large datasets, organizations can identify patterns, trends, and correlations related to sustainability issues such as climate change, pollution, biodiversity loss, and social inequality. Data analytics helps in understanding the causes and impacts of these challenges, enabling policymakers, researchers, and stakeholders to make informed decisions and take effective actions to address them.
2. Sustainable resource management through data-driven insights: 2.Data analytics plays a crucial role in sustainable resource management. By analyzing data on resource consumption, waste generation, energy usage, and supply chain operations, organizations can identify inefficiencies, optimize processes, and minimize environmental impacts. Data-driven insights enable better resource allocation, waste reduction, and energy conservation, leading to more sustainable practices in industries such as manufacturing, agriculture, transportation, and energy.
3. Sustainable business practices empowered by analytics: 3.Data analytics empowers businesses to adopt sustainable practices and make informed decisions that consider environmental and social impacts. Through analytics, companies can assess their carbon footprint, measure their environmental performance, and identify areas for improvement. Data-driven insights can help optimize supply chains, reduce emissions, identify sustainable sourcing options, and develop innovative eco-friendly products or services. Analytics also supports the measurement and reporting of sustainability metrics, enabling businesses to demonstrate their commitment to sustainability to customers, investors, and stakeholders.
In summary, data analytics has the potential to drive positive change by addressing sustainability challenges. By harnessing the power of data, organizations can gain insights into environmental and social issues, promote sustainable resource management, and adopt responsible business practices. Through data-driven decision-making, we can work towards a more sustainable future, where environmental conservation, social equity, and economic prosperity go hand in hand.
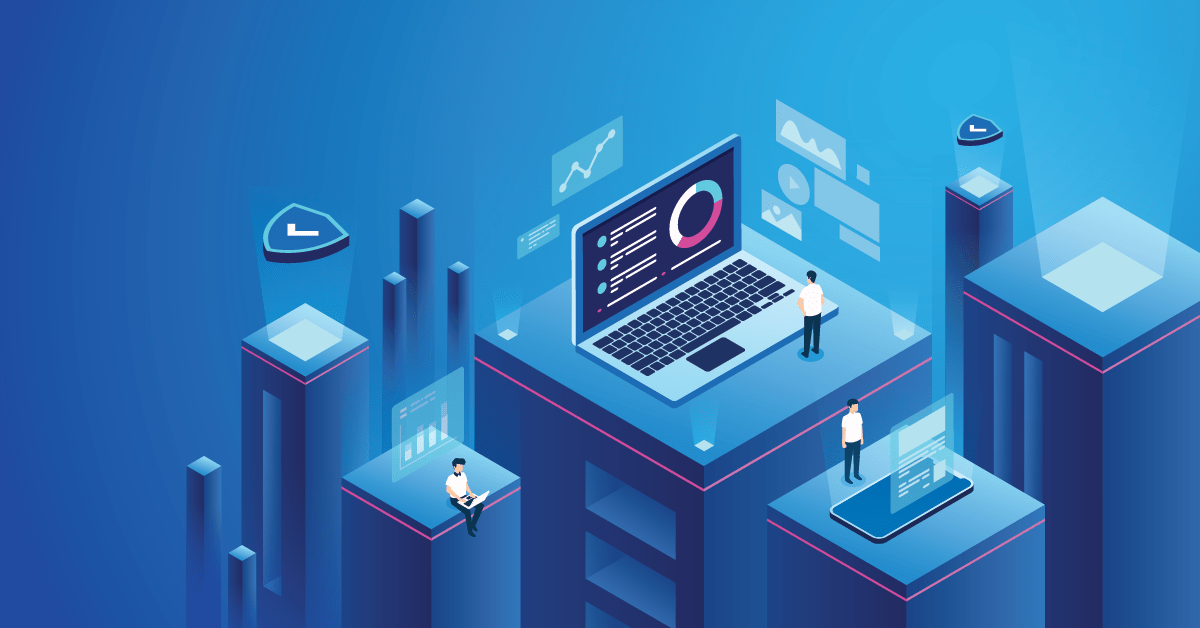
The Future of Data Analytics: Skills and Careers
The future of data analytics holds immense potential for both skills development and career opportunities. As data continues to grow in volume and complexity, organizations are increasingly relying on data analytics to drive strategic decision-making and gain a competitive edge.
1. Emerging roles in data analytics and AI: The future of data analytics holds the promise of new and exciting career opportunities. As technology continues to advance, emerging roles are expected to shape the field of data analytics and AI. Roles such as AI Architect, Data Science Manager, Machine Learning Engineer, and Predictive Analytics Specialist are likely to gain prominence. These roles focus on leveraging advanced analytics techniques, developing AI models, and leading data-driven initiatives within organizations.
1. Essential skills for future data analysts: To thrive in the future of data analytics, professionals need to acquire a diverse set of skills. Apart from a strong foundation in statistics, mathematics, and programming, future data analysts should possess skills in areas like machine learning, deep learning, natural language processing, and data visualization. Proficiency in handling big data, cloud computing, and knowledge of emerging technologies like edge computing and IoT will also be valuable. Additionally, soft skills such as critical thinking, problem-solving, and effective communication will play a crucial role in understanding business requirements and translating data insights into actionable recommendations.
1. Upskilling and professional development in the field of data analytics: Given the rapid evolution of data analytics, continuous upskilling and professional development are essential for individuals to stay relevant in their careers. Professionals should actively seek opportunities to enhance their skills through online courses, specialized certifications, and attending industry conferences and workshops. Keeping up with the latest trends, technologies, and best practices in data analytics ensures that professionals are equipped to handle complex data challenges and contribute effectively to organizational success. Additionally, participating in hands-on projects, collaborating with peers, and engaging in continuous learning platforms can provide valuable practical experience and foster professional growth.
In summary, the future of data analytics presents exciting possibilities for emerging roles and career paths. Professionals who develop a comprehensive skill set, encompassing both technical and soft skills, will be well-positioned to excel in the field. By embracing a culture of continuous learning and upskilling, individuals can adapt to the evolving data analytics landscape, seize new opportunities, and make significant contributions to organizations leveraging data-driven insights for success.
Become a Data Analyst with 360DigiTMG Data Analytics course. Get trained by the alumni from IIT, IIM, and ISB.
Data Analytics Training Institutes in Other Locations
Agra, Ahmedabad, Amritsar, Anand, Anantapur, Bangalore, Bhopal, Bhubaneswar, Chengalpattu, Chennai, Cochin, Dehradun, Dombivli, Durgapur, Ernakulam, Erode, Gandhinagar, Ghaziabad, Gorakhpur, Gwalior, Hebbal, Hyderabad, Jabalpur, Jalandhar, Jammu, Jamshedpur, Jodhpur, Khammam, Kolhapur, Kothrud, Ludhiana, Madurai, Meerut, Mohali, Moradabad, Noida, Pimpri, Pondicherry, Pune, Ranchi, Rohtak, Roorkee, Rourkela, Shimla, Shimoga, Siliguri, Srinagar, Thane, Thiruvananthapuram, Tiruchchirappalli, Trichur, Udaipur, Yelahanka, Andhra Pradesh, Anna Nagar, Bhilai, Borivali, Calicut, Chandigarh, Chromepet, Coimbatore, Dilsukhnagar, ECIL, Faridabad, Greater Warangal, Guduvanchery, Guntur, Gurgaon, Guwahati, Hoodi, Indore, Jaipur, Kalaburagi, Kanpur, Kharadi, Kochi, Kolkata, Kompally, Lucknow, Mangalore, Mumbai, Mysore, Nagpur, Nashik, Navi Mumbai, Patna, Porur, Raipur, Salem, Surat, Thoraipakkam, Trichy, Uppal, Vadodara, Varanasi, Vijayawada, Vizag, Tirunelveli, Aurangabad