Sent Successfully.
Home / Blog / Generative AI / Qdrant Vector Database
Qdrant Vector Database
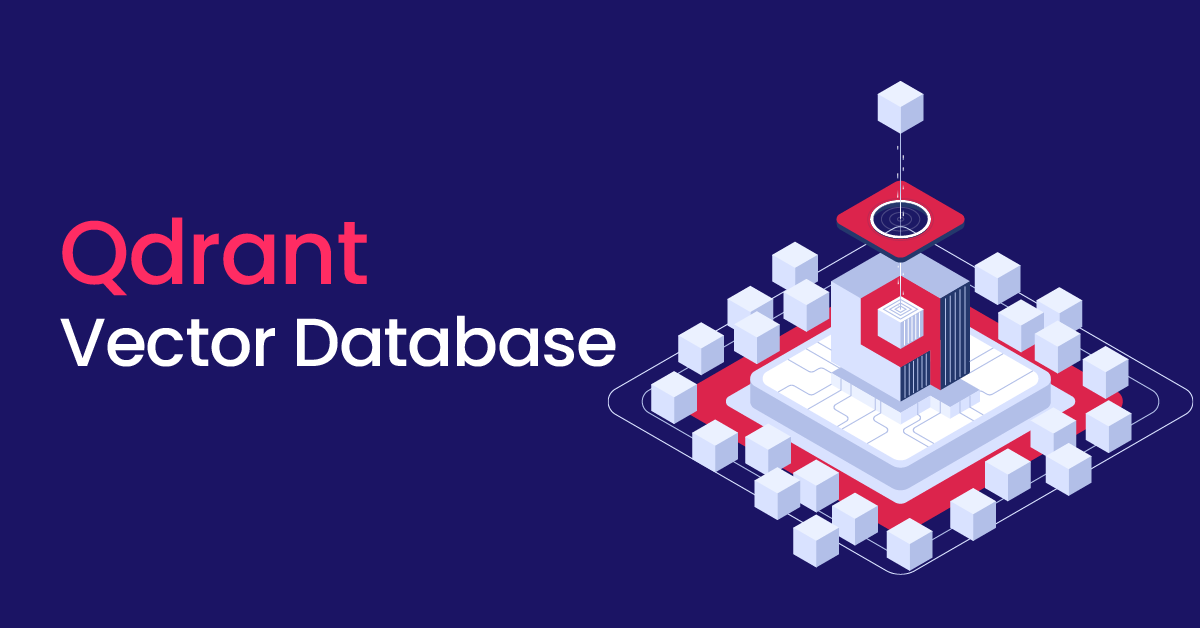
Table of Content
Introduction
In the ever-evolving landscape of data management and retrieval, the demand for efficient and scalable solutions is at an all-time high. One such groundbreaking technology that has emerged to meet these demands is the Qdrant Vector Database.
This extensive manual takes an in-depth look at Qdrant, examining its functionalities, strengths, and distinguishing factors when compared to alternative solutions such as Milvus. Furthermore, we'll offer guidance on incorporating Qdrant with Python and analyze its significance within the realm of OpenAI.
Understanding Qdrant Vector Database :
Qdrant Vector Database is a versatile and high-performance database designed specifically for managing and querying large-scale vector data. What sets it apart is its ability to handle vast amounts of vector embeddings, making it ideal for applications in fields such as natural language processing, computer vision, recommendation systems, and more.
Key Features of Qdrant Vector Database:
1. Scalability:
It is a key feature of Qdrant, designed for seamless horizontal expansion. Users can effortlessly increase their storage and processing capacities to accommodate growing data needs. This scalability is particularly vital for applications that handle extensive datasets.
2. Efficient Vector Search:
Qdrant excels in vector similarity search, enabling users to find similar vectors within large datasets quickly and accurately. This is a pivotal feature for applications like image retrieval, content recommendation, and similarity-based search.
3. Flexible Query Language:
The database supports a flexible query language that enables users to express complex search criteria for vector data. This flexibility is crucial for applications with diverse and dynamic search requirements.
4. Real-time Updates:
Qdrant is designed to accommodate real-time updates to vector data, ensuring that the database remains synchronized with changing data sources. This feature is valuable for applications where data is constantly evolving.
Qdrant vs. Milvus: A Comparative Analysis
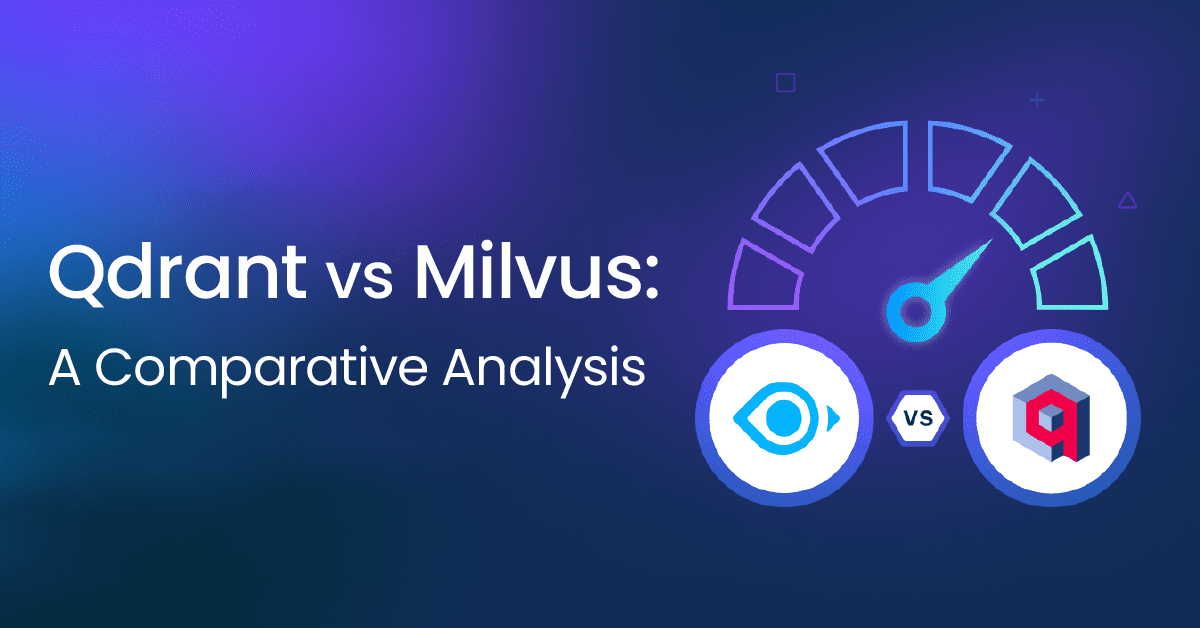
Milvus is another popular open-source vector database that shares similarities with Qdrant. Both databases are designed to handle large-scale vector data, but they have differences in terms of architecture, features, and use cases.
1. Architecture:
Qdrant employs a microservices architecture, offering flexibility and modularity. Each component in the Qdrant system is a separate service, enabling easy customization and scaling.
Milvus, on the other hand, follows a monolithic architecture. While this approach may simplify deployment, it might limit flexibility and scalability in certain scenarios.
2. Query Language:
Qdrant boasts a versatile and robust query language, empowering users to articulate intricate search criteria effectively. This adaptability renders it well-suited for a variety of applications with diverse and sophisticated search demands.
Milvus primarily uses SQL-like queries, which might be more familiar to users accustomed to relational databases. However, the flexibility of Qdrant's query language can be advantageous in certain use cases.
3. Scalability:
Both Qdrant and Milvus offer horizontal scalability, allowing users to add more nodes to their clusters for increased storage and processing capacity. However, the implementation details and ease of scalability may vary between the two databases.
4. Community and Support:
Both Qdrant and Milvus have active open-source communities. The level of community support, documentation, and third-party integrations can influence the ease of adoption and troubleshooting for users.
Qdrant and OpenAI: A Synergistic Approach
The integration of Qdrant with OpenAI technologies can lead to powerful and intelligent applications. Qdrant's efficient handling of vector data aligns seamlessly with the capabilities of OpenAI's language models and other AI services. By combining the strengths of Qdrant and OpenAI, developers can create applications that leverage the power of both vector similarity search and advanced natural language understanding.
Integrating Qdrant with Python
Qdrant provides a Python client that makes it seamless to integrate the database into Python applications. Here's a step-by-step guide on how to get started:
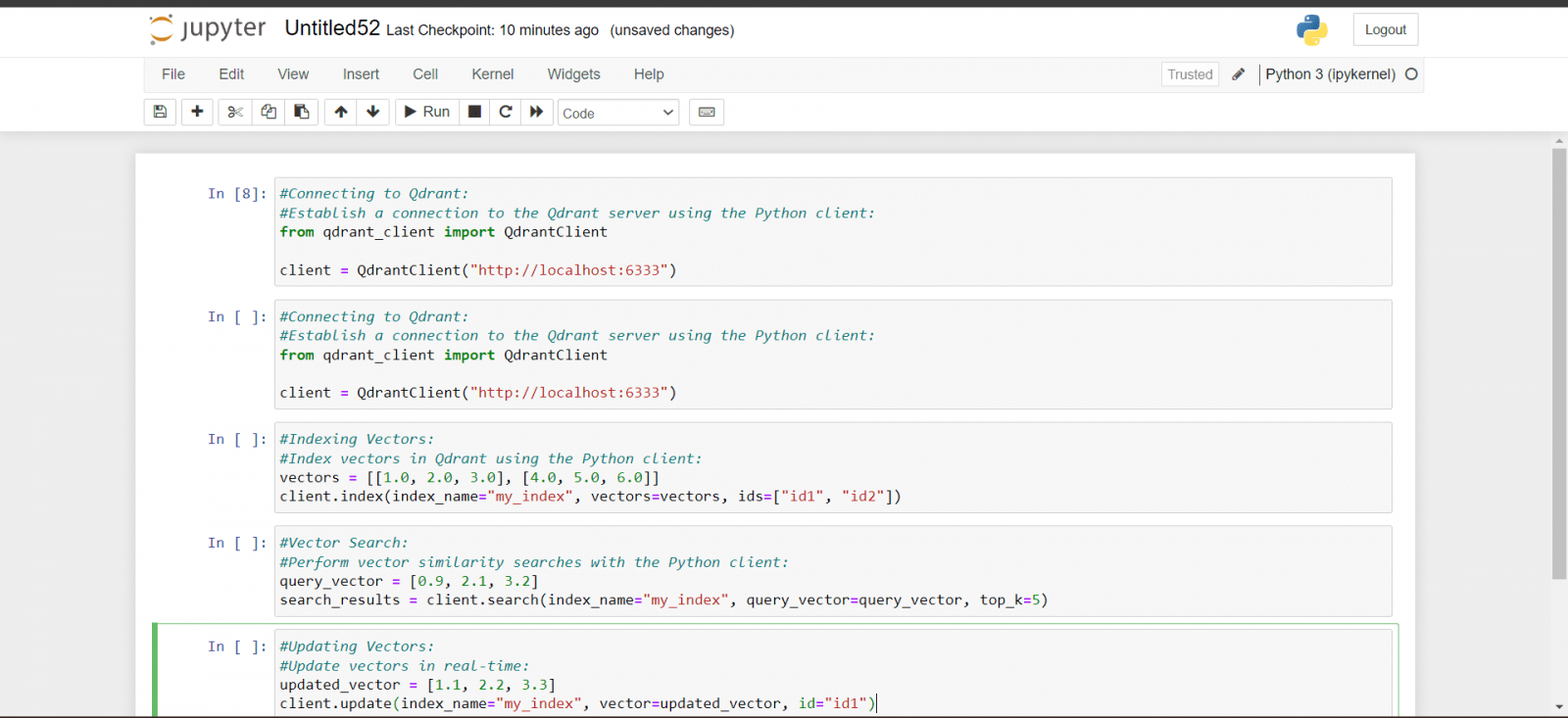
Conclusion:
Qdrant Vector Database stands out as a robust and scalable solution for managing large-scale vector data, offering efficient vector search, a flexible query language, and real-time updates. Its comparative analysis with alternatives like Milvus highlights its unique strengths, while seamless integration with Python and the potential synergy with OpenAI technologies position Qdrant as a versatile choice for developers.
As the demand for advanced data management solutions continues to grow, Qdrant's open-source nature and active community support make it a compelling candidate for applications ranging from natural language processing to recommendation systems, promising to shape the landscape of vector data management in the years to come.
Data Science Training Institutes in Other Locations
Agra, Ahmedabad, Amritsar, Anand, Anantapur, Bangalore, Bhopal, Bhubaneswar, Chengalpattu, Chennai, Cochin, Dehradun, Malaysia, Dombivli, Durgapur, Ernakulam, Erode, Gandhinagar, Ghaziabad, Gorakhpur, Gwalior, Hebbal, Hyderabad, Jabalpur, Jalandhar, Jammu, Jamshedpur, Jodhpur, Khammam, Kolhapur, Kothrud, Ludhiana, Madurai, Meerut, Mohali, Moradabad, Noida, Pimpri, Pondicherry, Pune, Rajkot, Ranchi, Rohtak, Roorkee, Rourkela, Shimla, Shimoga, Siliguri, Srinagar, Thane, Thiruvananthapuram, Tiruchchirappalli, Trichur, Udaipur, Yelahanka, Andhra Pradesh, Anna Nagar, Bhilai, Borivali, Calicut, Chandigarh, Chromepet, Coimbatore, Dilsukhnagar, ECIL, Faridabad, Greater Warangal, Guduvanchery, Guntur, Gurgaon, Guwahati, Hoodi, Indore, Jaipur, Kalaburagi, Kanpur, Kharadi, Kochi, Kolkata, Kompally, Lucknow, Mangalore, Mumbai, Mysore, Nagpur, Nashik, Navi Mumbai, Patna, Porur, Raipur, Salem, Surat, Thoraipakkam, Trichy, Uppal, Vadodara, Varanasi, Vijayawada, Vizag, Tirunelveli, Aurangabad
Data Analyst Courses in Other Locations
ECIL, Jaipur, Pune, Gurgaon, Salem, Surat, Agra, Ahmedabad, Amritsar, Anand, Anantapur, Andhra Pradesh, Anna Nagar, Aurangabad, Bhilai, Bhopal, Bhubaneswar, Borivali, Calicut, Cochin, Chengalpattu, Dehradun, Dombivli, Durgapur, Ernakulam, Erode, Gandhinagar, Ghaziabad, Gorakhpur, Guduvanchery, Gwalior, Hebbal, Hoodi , Indore, Jabalpur, Jaipur, Jalandhar, Jammu, Jamshedpur, Jodhpur, Kanpur, Khammam, Kochi, Kolhapur, Kolkata, Kothrud, Ludhiana, Madurai, Mangalore, Meerut, Mohali, Moradabad, Pimpri, Pondicherry, Porur, Rajkot, Ranchi, Rohtak, Roorkee, Rourkela, Shimla, Shimoga, Siliguri, Srinagar, Thoraipakkam , Tiruchirappalli, Tirunelveli, Trichur, Trichy, Udaipur, Vijayawada, Vizag, Warangal, Chennai, Coimbatore, Delhi, Dilsukhnagar, Hyderabad, Kalyan, Nagpur, Noida, Thane, Thiruvananthapuram, Uppal, Kompally, Bangalore, Chandigarh, Chromepet, Faridabad, Guntur, Guwahati, Kharadi, Lucknow, Mumbai, Mysore, Nashik, Navi Mumbai, Patna, Pune, Raipur, Vadodara, Varanasi, Yelahanka