Sent Successfully.
Home / Blog / Big Data & Analytics / Data Analytics Trends : Exploring the Most Important Trends
Data Analytics Trends : Exploring the Most Important Trends
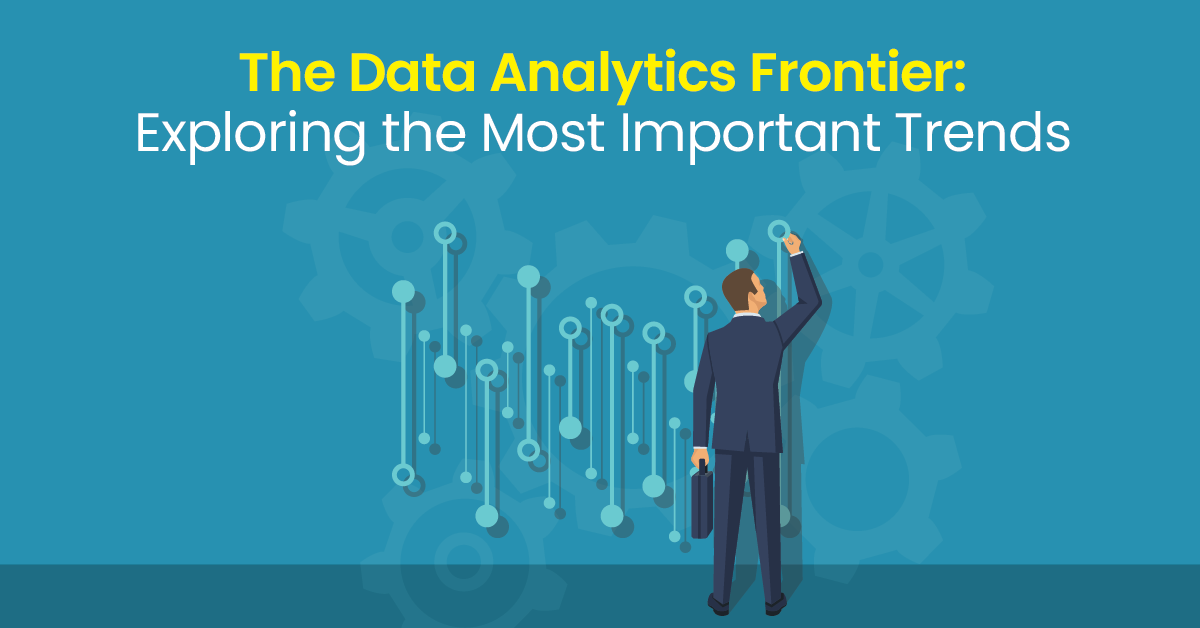
Table of Content
In today's rapidly evolving digital landscape, data analytics has emerged as a powerful tool for extracting meaningful insights and driving informed decision-making. As technology advances and new data sources become available, staying up-to-date with the latest trends in data analytics is crucial for organizations and professionals in the field. This introduction sets the stage for exploring the most important data analytics trends that are shaping the industry and provides an overview of why keeping abreast of these trends is essential.
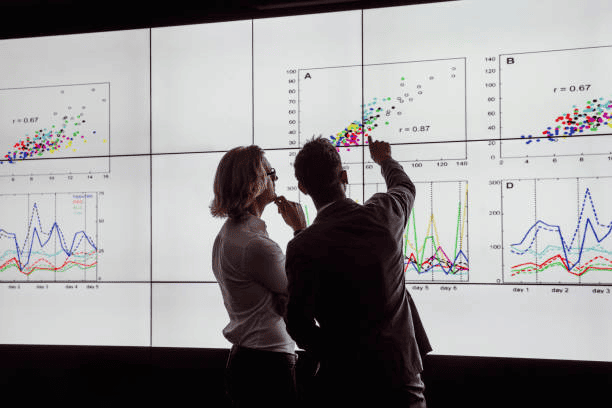
Data analytics trends are the innovative approaches, techniques, and technologies that are revolutionizing the way organizations analyze and leverage data. These trends have the potential to unlock new opportunities, enhance operational efficiency, and generate valuable insights that drive strategic growth. By understanding and embracing these trends, businesses can gain a competitive advantage, optimize processes, and make data-driven decisions with confidence.
Are you looking to become a Data Analytics Expert? Go through 360DigiTMG's Data Analytics course in Hyderabad!
Learn the core concepts of Data Science Course video on YouTube:
The significance of staying updated with data analytics trends
The significance of staying updated with data analytics trends cannot be overstated in today's data-driven world. Here are some key reasons why it is crucial to stay informed and proactive in keeping up with these trends
1. Competitive Advantage: By staying updated with data analytics trends, organizations can gain a competitive edge over their peers. The adoption of emerging technologies and techniques allows businesses to harness data in innovative ways, uncover hidden patterns, and make informed decisions faster. Staying ahead of the curve ensures that organizations are better equipped to respond to market changes, customer demands, and industry disruptions.
2. Improved Decision-Making: Data analytics trends often introduce more advanced and sophisticated techniques for analyzing data. Keeping up with these trends enables organizations to refine their decision-making processes by leveraging the latest methodologies and tools. By harnessing the power of predictive and prescriptive analytics, organizations can make data-driven decisions that are based on accurate forecasts and insights, leading to improved outcomes and performance.
3. Enhanced Operational Efficiency: Data analytics trends frequently bring about new approaches to streamline and optimize operations. From automation and machine learning to process optimization and real-time analytics, these trends enable organizations to identify inefficiencies, reduce costs, and improve overall efficiency. Staying updated with these trends ensures that organizations can leverage the latest solutions to enhance productivity and maximize their resources.
4. Better Customer Understanding: Data analytics provides valuable insights into customer behavior, preferences, and needs. By staying updated with trends in customer analytics, organizations can gain a deeper understanding of their target audience, tailor their products and services to meet customer expectations, and deliver personalized experiences. This customer-centric approach can foster loyalty, drive customer satisfaction, and ultimately lead to business growth.
5. Innovation and Agility: The field of data analytics is constantly evolving, with new trends continuously emerging. Staying updated with these trends encourages a culture of innovation and agility within organizations. It allows for the exploration of new methodologies, tools, and approaches, fostering a mindset of continuous improvement and learning. Embracing innovative data analytics trends positions organizations as early adopters, enabling them to quickly adapt to changing business landscapes and seize emerging opportunities.
6. Future-Proofing Careers: For professionals in the data analytics field, staying updated with trends is crucial for career growth and future-proofing. Employers seek individuals who possess the latest skills, knowledge, and expertise in data analytics. By actively staying informed and upskilling in line with trends, professionals can enhance their value in the job market, stay relevant in their roles, and open doors to exciting career opportunities.
How trends shape the future of data analytics
Trends play a pivotal role in shaping the future of data analytics. They drive innovation, influence technological advancements, and redefine the way organizations approach data analysis. Here are some ways in which trends shape the future of data analytics
1. Technological Advancements: Trends in data analytics often coincide with advancements in technology. As new technologies emerge and mature, they enable more sophisticated data collection, storage, processing, and analysis. Trends such as cloud computing, edge computing, artificial intelligence, and machine learning are reshaping the data analytics landscape by providing organizations with powerful tools and capabilities to tackle complex data challenges. These advancements unlock new possibilities and fuel the development of more advanced analytical techniques and methodologies.
2. Data Volume and Variety: With the exponential growth of data, trends in data analytics are driven by the need to handle vast volumes and diverse types of data. The rise of big data and the proliferation of data sources, including IoT devices, social media platforms, and sensors, have necessitated the development of scalable and efficient analytics solutions. Trends in data analytics focus on addressing the challenges of managing and analyzing large datasets, integrating structured and unstructured data, and extracting insights from diverse data sources.
3. Real-Time Analytics: The demand for real-time insights has become increasingly crucial for organizations across various industries. Trends in data analytics are centered around enabling real-time analytics capabilities. Organizations require timely and actionable insights to make informed decisions, detect anomalies, respond to events, and optimize operations in real-time. As a result, trends focus on technologies like in-memory computing, stream processing, and event-driven architectures to support real-time analytics and decision-making.
4. Advanced Analytical Techniques: Trends in data analytics are continuously pushing the boundaries of analytical techniques. Traditional descriptive analytics has expanded to include predictive and prescriptive analytics. Predictive analytics leverages statistical modeling and machine learning algorithms to forecast future outcomes and trends, while prescriptive analytics provides actionable recommendations for optimal decision-making. Trends also encompass advanced techniques such as natural language processing, network analytics, and graph analytics, enabling organizations to derive deeper insights from their data.
5. Ethical and Responsible Analytics: The increasing awareness of ethical considerations in data analytics has influenced trends in the field. With the rising concerns surrounding data privacy, bias, and fairness, trends now emphasize ethical and responsible analytics practices. Organizations are expected to handle data ethically, ensure transparency, and implement frameworks that address privacy concerns and promote fair and unbiased data analysis. Trends in data analytics include methods and techniques for ensuring data governance, data quality, and compliance with regulations.
6. Democratization of Data Analytics: Data analytics trends are also focused on democratizing access to data and analytics capabilities. The aim is to empower a broader range of users within organizations to leverage data and make data-driven decisions. Trends encompass user-friendly analytics tools, self-service analytics platforms, and intuitive visualizations that enable business users, domain experts, and non-technical professionals to explore data, generate insights, and derive value from analytics without heavy reliance on data scientists or IT departments.
Want to learn more about Data Anlytics? Enroll in this Data Analytics training in Bangalore to do so.
Artificial Intelligence and Machine Learning
Artificial Intelligence (AI) and Machine Learning (ML) are revolutionizing the field of data analytics, enabling organizations to extract valuable insights from vast amounts of data. AI refers to the development of intelligent machines that can mimic human cognitive abilities, while ML focuses on the ability of machines to learn from data and improve their performance without explicit programming. Together, AI and ML play a crucial role in enhancing data analytics capabilities. Here's a closer look at their significance:
1. Enhancing Data Analysis: AI and ML techniques bring advanced analytical capabilities to data analytics. These technologies can process and analyze large datasets with speed and efficiency, identifying patterns, correlations, and trends that may not be apparent to human analysts.
2. Predictive Analytics: One of the key applications of AI and ML in data analytics is predictive analytics. ML algorithms can analyze historical data, identify patterns, and build models that predict future outcomes.
3. Automation and Efficiency: AI and ML technologies automate repetitive and time-consuming data analysis tasks, enabling organizations to improve efficiency and productivity. By automating tasks like data cleaning, feature selection, and model training, data analysts can focus on higher-level analysis and interpretation of results.
4. Personalization and Recommendation Systems: AI and ML have transformed the way organizations deliver personalized experiences to customers.
5. Anomaly Detection and Fraud Prevention: AI and ML techniques are effective in detecting anomalies and identifying potential fraudulent activities.
6. Natural Language Processing (NLP): NLP is a subset of AI that focuses on the interaction between computers and human language. It enables machines to understand, interpret, and generate human language, opening up possibilities for analyzing unstructured text data such as customer reviews, social media posts, and emails.
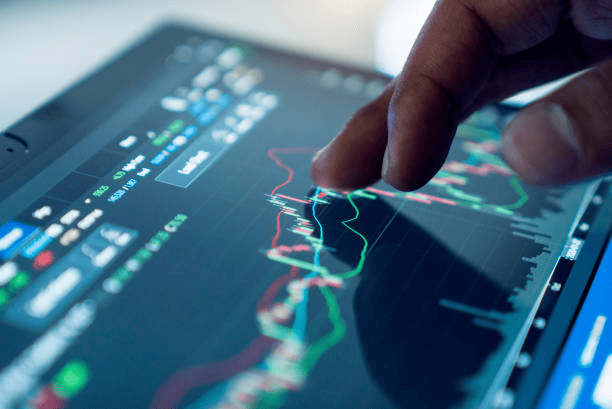
Big Data and Cloud Computing
Big Data and Cloud Computing are two interconnected concepts that are revolutionizing the way organizations store, process, and analyze large volumes of data. Here's a description of each and how they are related.
1. Big Data: Big Data refers to the massive amounts of structured, semi-structured, and unstructured data that organizations generate and collect from various sources. This data is characterized by its volume, velocity, and variety, making it challenging to process and analyze using traditional data management and analysis tools. Big Data encompasses diverse data types such as text, images, videos, sensor data, social media posts, and more. It offers valuable insights and business opportunities when effectively analyzed.
2. Cloud Computing: Cloud Computing involves the delivery of computing resources, including storage, processing power, and applications, over the internet. Instead of relying on local servers and infrastructure, organizations can leverage cloud service providers to access and utilize these resources on-demand. Cloud Computing offers scalability, flexibility, and cost-effectiveness, as organizations can pay for the resources they use without the need for significant upfront investments in hardware or maintenance.
3. The Relationship: Big Data and Cloud Computing are closely intertwined and often go hand in hand. Here's how they are related:
a) Storage and Scalability: Big Data requires significant storage capacity, and cloud platforms provide scalable storage solutions that can handle massive data volumes. Organizations can leverage cloud storage to accommodate their growing data needs, easily expanding or reducing storage resources as required.
b) Processing Power: Analyzing Big Data necessitates considerable processing power. Cloud Computing offers high-performance computing resources that can handle complex data analytics tasks efficiently. Cloud-based processing allows organizations to parallelize computations, distribute workloads, and achieve faster data processing and analysis.
c) Elasticity and Cost Savings: Cloud platforms offer elasticity, allowing organizations to scale computing resources up or down based on data processing demands. With Big Data workloads that have variable processing requirements, organizations can leverage the scalability of cloud resources, paying only for the resources they use. This flexibility helps optimize costs and ensures efficient resource utilization
d) Data Integration and Collaboration: Big Data often originates from multiple sources and systems within an organization. Cloud Computing provides integration capabilities, enabling organizations to ingest, store, and integrate data from diverse sources in a centralized cloud environment. This promotes data collaboration and enables analysts and data scientists to work together seamlessly on shared datasets.
e) Analytical Capabilities: Cloud platforms provide a wide range of analytics tools and services that support Big Data processing and analysis. These tools leverage distributed computing and parallel processing capabilities to extract insights from large datasets. Organizations can utilize cloud-based analytics services to apply machine learning algorithms, perform advanced analytics, and gain actionable insights from Big Data.
f) Accessibility and Availability: Cloud Computing facilitates remote access to data and analytics resources. This allows geographically dispersed teams to collaborate on Big Data projects, access analytics tools, and share insights seamlessly. Cloud-based solutions enable organizations to make data and analytics capabilities available to a broader user base, fostering a data-driven culture across the organization.
Earn yourself a promising career in Data Analytics by enrolling Best Data Analyst Course in Chennai offered by 360DigiTMG.
Predictive Analytics and Prescriptive Analytics
Predictive Analytics and Prescriptive Analytics are two branches of advanced data analytics that enable organizations to make data-driven decisions based on insights derived from historical and real-time data. Here's a description of each:
1. Predictive Analytics: Predictive Analytics involves the use of statistical modeling and machine learning algorithms to forecast future outcomes and trends based on historical data patterns. It aims to predict what is likely to happen in the future, allowing organizations to anticipate and prepare for various scenarios. Predictive Analytics leverages advanced algorithms to analyze historical data, identify patterns, and build predictive models that can be used to make informed predictions and projections. By analyzing historical data, organizations can uncover relationships, correlations, and trends that help predict future events or outcomes. Predictive Analytics is commonly used in various domains, such as sales forecasting, demand planning, risk assessment, fraud detection, customer churn prediction, and healthcare outcomes prediction. It provides organizations with insights into potential future outcomes, enabling them to make proactive decisions, optimize processes, and improve operational efficiency.
2. Prescriptive Analytics: Prescriptive Analytics takes the insights provided by Predictive Analytics a step further by recommending optimal actions or decisions to achieve desired outcomes. It goes beyond predicting what will happen and provides recommendations on what actions should be taken to maximize desired results or minimize negative outcomes. Prescriptive Analytics utilizes mathematical models, optimization techniques, and simulation algorithms to evaluate multiple scenarios and determine the best course of action. Prescriptive Analytics considers various factors, constraints, and objectives to provide decision-makers with actionable insights and recommendations. It helps organizations optimize their decision-making processes, allocate resources efficiently, and identify the most effective strategies. Prescriptive Analytics is widely used in supply chain optimization, resource allocation, pricing optimization, risk management, and strategic planning.
3. The Relationship: Predictive Analytics and Prescriptive Analytics are closely related and often work together in a complementary manner
- Predictive Analytics forms the foundation for Prescriptive Analytics. By analyzing historical data and identifying patterns and trends, Predictive Analytics provides the necessary insights to understand what is likely to happen in the future.
- Prescriptive Analytics builds upon Predictive Analytics by incorporating optimization techniques and decision models to recommend the best course of action. It takes into account various factors, constraints, and objectives to provide actionable recommendations.
- The insights generated by Predictive Analytics feed into the decision-making process of Prescriptive Analytics. By understanding potential future outcomes, Prescriptive Analytics can evaluate different scenarios and determine the optimal actions to achieve desired results.
- Predictive Analytics and Prescriptive Analytics are iterative processes. As new data becomes available and new insights are gained, the models and recommendations can be updated to continuously improve the accuracy and effectiveness of predictions and prescriptions.
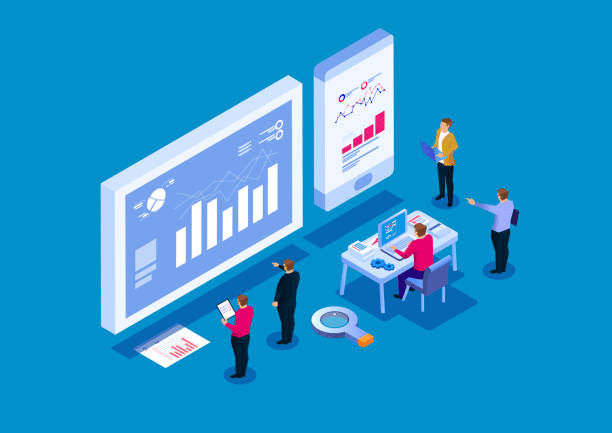
Looking forward to becoming a Data Analytics expert? Check out the Data Analytics Course and get certified today.
Data Visualization and Interactive Dashboards
Data Visualization and Interactive Dashboards are essential tools in data analytics that help organizations effectively communicate insights and make data-driven decisions. Here's a description of each:
1. Data Visualization: Data Visualization involves the representation of data in visual formats, such as charts, graphs, maps, and infographics. It is the process of transforming the complex data into the visual representations that are both easily understandable and interpretable. Data Visualization aims to communicate patterns, trends, and relationships in the data, allowing users to gain insights and make informed decisions.
Effective data visualization utilizes design principles, such as the selection of appropriate visual elements, color schemes, and layouts, to present data in a visually appealing and meaningful way. It helps to uncover patterns, outliers, and correlations that may not be apparent in raw data. By presenting data visually, organizations can quickly grasp information, identify insights, and communicate findings to a wide range of stakeholders.
2. Interactive Dashboards: Interactive Dashboards are dynamic and user-friendly interfaces that provide real-time access to visualized data and allow users to explore and interact with the data. Dashboards consolidate relevant metrics, KPIs (Key Performance Indicators), and data visualizations into a single interface, enabling users to monitor performance, track trends, and gain insights at a glance.
Interactive dashboards offer features such as filtering, drill-down, and data exploration capabilities, empowering users to customize their data views and focus on specific areas of interest. They facilitate data exploration and enable users to interactively manipulate visualizations to gain deeper insights or answer specific questions. Interactive dashboards enhance data analysis, support decision-making, and promote data-driven collaboration within organizations.
3. The Relationship: Data Visualization and Interactive Dashboards are closely related and often used together to enhance data analysis and decision-making processe
i) Visualizing Complex Data: Data Visualization techniques are applied to present complex data in a simplified and understandable format. Visualizations, such as charts, graphs, and diagrams, help users identify patterns, trends, and outliers quickly. These visual representations are often embedded within interactive dashboards, providing users with a comprehensive view of the data.
ii) Enhancing User Experience: Interactive Dashboards leverage Data Visualization techniques to create engaging and interactive interfaces. Users can explore and interact with visualized data, drill down into specific details, apply filters, and adjust parameters to gain deeper insights. The combination of data visualization and interactivity enhances the user experience, making it easier for users to analyze and interpret data.
iii) Facilitating Data Exploration: Interactive Dashboards enable users to manipulate visualizations dynamically, allowing them to interactively explore the data from different angles. Users can change variables, apply filters, and drill down into specific data subsets to uncover insights and answer ad-hoc questions. This flexibility promotes data exploration and empowers users to derive meaningful insights from the data.
iv) Effective Communication: Both Data Visualization and Interactive Dashboards play a crucial role in effectively communicating insights and findings to stakeholders. By presenting data in a visually appealing and interactive manner, organizations can convey complex information in a way that is easily understandable and actionable. Interactive dashboards enable users to share specific visualizations, filter data, and collaborate with others, fostering data-driven decision-making across the organization.
Data Analytics Placement Success Story
Data Analytics Training Institutes in Other Locations
Agra, Ahmedabad, Amritsar, Anand, Anantapur, Bangalore, Bhopal, Bhubaneswar, Chengalpattu, Chennai, Cochin, Dehradun, Malaysia, Dombivli, Durgapur, Ernakulam, Erode, Gandhinagar, Ghaziabad, Gorakhpur, Gwalior, Hebbal, Hyderabad, Jabalpur, Jalandhar, Jammu, Jamshedpur, Jodhpur, Khammam, Kolhapur, Kothrud, Ludhiana, Madurai, Meerut, Mohali, Moradabad, Noida, Pimpri, Pondicherry, Pune, Rajkot, Ranchi, Rohtak, Roorkee, Rourkela, Shimla, Shimoga, Siliguri, Srinagar, Thane, Thiruvananthapuram, Tiruchchirappalli, Trichur, Udaipur, Yelahanka, Andhra Pradesh, Anna Nagar, Bhilai, Borivali, Calicut, Chandigarh, Chromepet, Coimbatore, Dilsukhnagar, ECIL, Faridabad, Greater Warangal, Guduvanchery, Guntur, Gurgaon, Guwahati, Hoodi, Indore, Jaipur, Kalaburagi, Kanpur, Kharadi, Kochi, Kolkata, Kompally, Lucknow, Mangalore, Mumbai, Mysore, Nagpur, Nashik, Navi Mumbai, Patna, Porur, Raipur, Salem, Surat, Thoraipakkam, Trichy, Uppal, Vadodara, Varanasi, Vijayawada, Vizag, Tirunelveli, Aurangabad