Sent Successfully.
Home / Blog / Data Science Digital Book / Model Evaluation Techniques
Model Evaluation Techniques
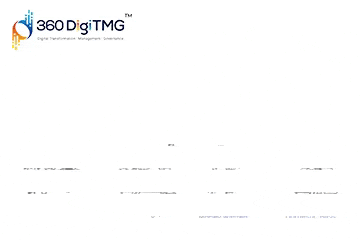
The set of error functions below can be used to assess the model if the output variable 'Y' is continuous.
Error = Predicted Value - Actual Value (Actual Value is also called as Ground Truth Value)
- Mean Error (ME)
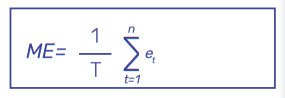
- Mean Absolute Error (MAE) or Mean Absolute Deviation (MAD)
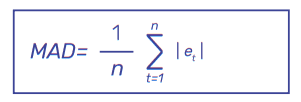
- Mean Squared Error (MSE)
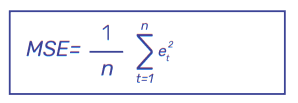
- Root Mean Squared Error (RMSE)
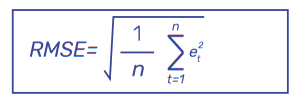
- Mean Percentage Error (MPE)
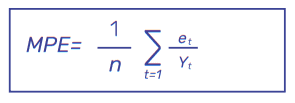
- Mean Absolute Percentage Error (MAPE)
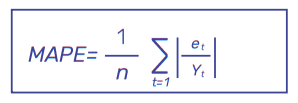
- Mean Absolute Scaled Error (MASE)
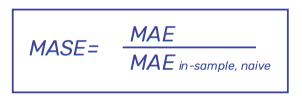
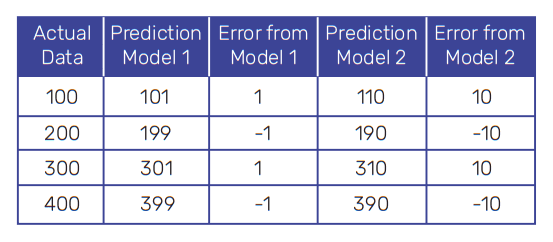
- Correlation Coefficient
Learn the core concepts of Data Science Course video on YouTube:
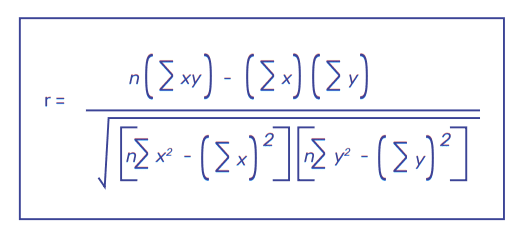
Click here to learn Data Science in Hyderabad
MAEin-sample naive is the mean absolute error produced by a naive forecast
If the ‘Y’ is Discrete variable (Classification Models) then we can use the following list:
Click here to learn Data Analytics in Bangalore
Confusion Matrix:
can be used for multi-class classification models as well as binary classifications.
To compare anticipated values with actual values, confusion matrix is employed.
Binary Classification Confusion Matrix:
Click here to learn Data Analytics in Hyderabad
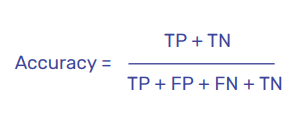
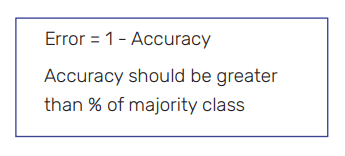
Click here to learn Artificial Intelligence in Bangalore
-
True Positive (TP)
Patient with disease is told that he/she has disease -
True Negative (TN)
Patient with no disease is told that he/she has no disease -
False Positive (FP)
Patient with no disease is told that he/she has disease -
False Negative (FN)
Patient with disease is told that he/she has no disease
Click here to learn Artificial Intelligence in Hyderabad
- Precision = Prob. of correctly identifying a random patient with disease as having disease
Precision is also called as Positive Predictive Value (PPV)
- Sensitivity (Recall or Hit rate or True Positive Rate) = Proportion of people with disease who are correctly identified as having disease
- Specificity (True negative rate) = TN/(TN+FP) = Proportion of people with no disease being characterized as not having disease
- FP rate (Alpha or type I error) = 1 - Specificity
- FN rate (Beta or type II error) = 1 - Sensitivity
- F1 = 2 * ((Precision * Recall) / (Precision + Recall)); F1: 1 to 0 & defines a measure that balances precision & recall
Click here to learn Machine Learning in Hyderabad
F1 score is the harmonic mean of precision and recall. Closer the ‘F1’ value to 1, better the accuracy.
Confusion Matrix
A cross table or contingency table are other names for a confusion matrix. Here is a multi-class categorization issue example.
Values off the diagonal are incorrectly predicted, whereas values along the diagonal are correctly predicted.
ROC Curve
Since World War II, the Receiver Operating Characteristic Curve has been employed to discern between genuine signals and false alarms.
The 'True Positive Rate (TPR)' and 'False Positive Rate (FPR)' are plotted on the Y-axis and X-axis, respectively, of the ROC curve.
Accuracy is represented graphically using the ROC curve.
The cut-off value is also determined using the ROC curve.
Examples include: Risk Neutral: The probability should be > 0.5 as the cut-off value to classify a customer under the "will default" category; Risk Taking: The probability should be > 0.8 as the cut-off value to classify a customer under the "will default" category; or Risk Averse: The probability should be > 0.3 as the cut-off value to classify a customer under the "will default" category.
AUC (Area Under the Curve) may be determined numerically if one needs to assess the accuracy.
0.9 - 1.0 = A (outstanding)
0.8 - 0.9 = B (excellent/good)
0.7 - 0.8 = C (acceptable/fair)
0.6 - 0.7 = D (poor)
0.5 - 0.6 = F (no discrimination)
Click here to learn Data Science Course, Data Science Course in Hyderabad, Data Science Course in Bangalore
Data Science Placement Success Story
Data Science Training Institutes in Other Locations
Agra, Ahmedabad, Amritsar, Anand, Anantapur, Bangalore, Bhopal, Bhubaneswar, Chengalpattu, Chennai, Cochin, Dehradun, Malaysia, Dombivli, Durgapur, Ernakulam, Erode, Gandhinagar, Ghaziabad, Gorakhpur, Gwalior, Hebbal, Hyderabad, Jabalpur, Jalandhar, Jammu, Jamshedpur, Jodhpur, Khammam, Kolhapur, Kothrud, Ludhiana, Madurai, Meerut, Mohali, Moradabad, Noida, Pimpri, Pondicherry, Pune, Rajkot, Ranchi, Rohtak, Roorkee, Rourkela, Shimla, Shimoga, Siliguri, Srinagar, Thane, Thiruvananthapuram, Tiruchchirappalli, Trichur, Udaipur, Yelahanka, Andhra Pradesh, Anna Nagar, Bhilai, Borivali, Calicut, Chandigarh, Chromepet, Coimbatore, Dilsukhnagar, ECIL, Faridabad, Greater Warangal, Guduvanchery, Guntur, Gurgaon, Guwahati, Hoodi, Indore, Jaipur, Kalaburagi, Kanpur, Kharadi, Kochi, Kolkata, Kompally, Lucknow, Mangalore, Mumbai, Mysore, Nagpur, Nashik, Navi Mumbai, Patna, Porur, Raipur, Salem, Surat, Thoraipakkam, Trichy, Uppal, Vadodara, Varanasi, Vijayawada, Vizag, Tirunelveli, Aurangabad
Navigate to Address
360DigiTMG - Data Science, IR 4.0, AI, Machine Learning Training in Malaysia
Level 16, 1 Sentral, Jalan Stesen Sentral 5, Kuala Lumpur Sentral, 50470 Kuala Lumpur, Wilayah Persekutuan Kuala Lumpur, Malaysia
+60 19-383 1378