Sent Successfully.
Home / Blog / Data Science / What is Dimensionality Reduction? : Techniques, Applications and Challenges
What is Dimensionality Reduction? : Techniques, Applications and Challenges
.png)
Table of Content
Introduction
In the era of big data, businesses and researchers are inundated with massive data sets. Although this data contains valuable information, it often becomes impractical and challenging to extract meaningful insights from it. This is where Dimensionality reduction techniques come to the rescue.
Data Science, AI and Data Engineering is a promising career option. Enroll in Data Science course in Chennai Program offered by 360DigiTMG to become a successful Career.
This blog deeply explores dimensionality reduction, exploring its concepts, methods, and real-world applications.
Learn the core concepts of Data Science Course video on YouTube:
What is Dimensionality Reduction?
Imagine you have a dataset with hundreds or thousands of features or variables. Each feature can add complexity to your data, making it more challenging to analyze, visualize, and interpret. This is where size reduction comes in. Dimensionality reduction is a set of techniques to reduce the number of features in a data sets while preserving its basic information.
Why Do We Need Dimensionality Reduction
1. Curse of dimensionality: As the number of elements increases, the amount of data in the element space grows exponentially.This can lead to computational inefficiencies and an increased risk of overfitting machine learning models.
2. Visualization: It is challenging to visualize high-dimensional data effectively. Reducing Dimensionalitys allows us to plot and explore data more intuitively.
Become a Data Science Course expert with a single program. Go through 360DigiTMG's Data Science Course Course in Hyderabad. Enroll today!
3. Interpretability: Simplified data is more accessible and can lead to more actionable insights.
4. Feature Engineering: Reducing the number of features can help in feature selection and engineering, making models more robust.
Dimensionality Reduction Techniques
There are two primary categories of Dimensionality reduction techniques: feature selection and feature extraction.
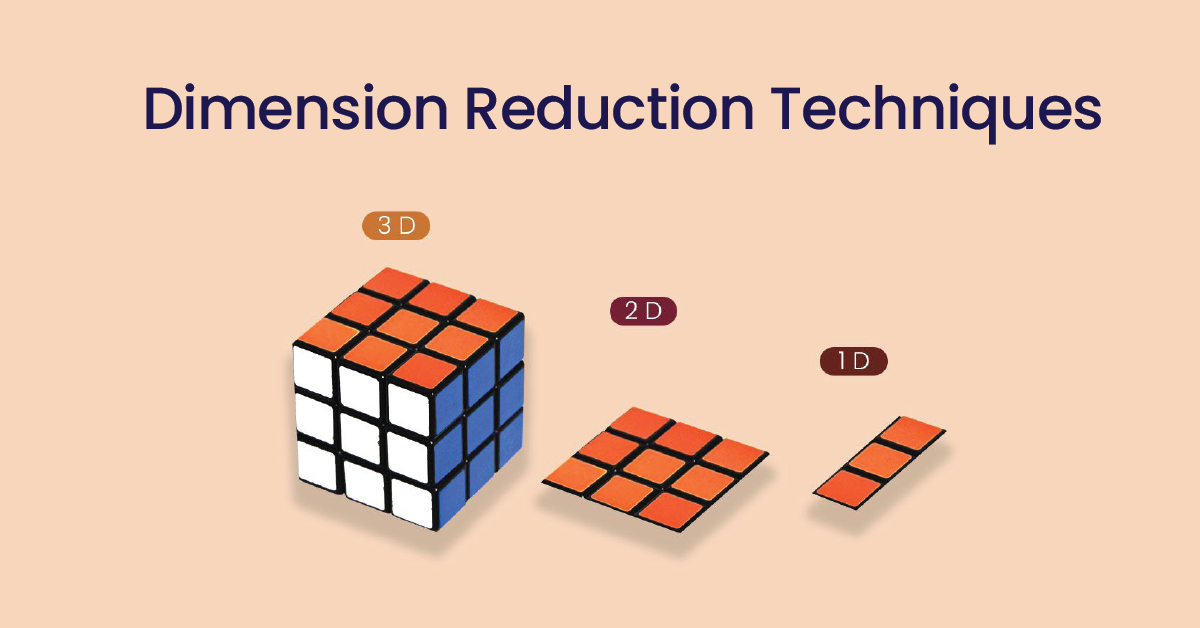
Feature Selection
Two primary categories of Dimensionality reduction techniques exist: feature selection and feature extraction.
Feature selection is a process in which we select some relevant features from the original dataset. Common methods include:
1) Filtering methods Use statistical measures such as correlation or mutual information to evaluate and select features.
2) Wrapper methods: Wrapper methods evaluate the performance of a machine learning model with different subsets of features and select the best-performing subset.
3) Built-in methods: These methods select features during the model training process. For example, L1 regulation can automatically reduce the coefficients and perform element selection efficiently.
Autoencoders: These neural networks learn a compressed representation of data by encoding it into a lower dimensional space and then decoding it back into the original dimension.
Real-World Applications
Dimensionality reduction techniques find application in various areas:
1) Image and video processing
In image and video analysis, dimensionality reduction helps reduce the computational burden and extract essential features. For example, face recognition systems often use PCA to remove facial features efficiently.
2) Natural Language Processing (NLP)
In NLP, word embeddings like Word2Vec and GloVe perform Dimensionality reduction to represent words in a lower-dimensional space while preserving their semantic relationships.
Challenges and Considerations
While Dimensionality reduction offers many benefits, it is not without challenges:
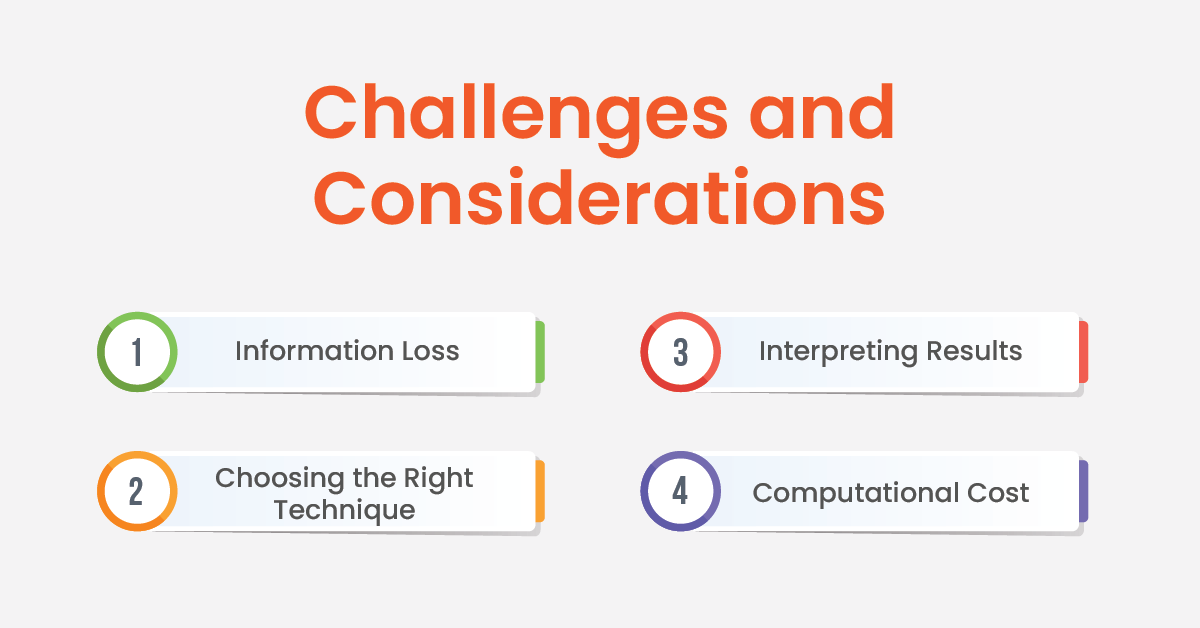
1) Information Loss: Dimensionality reduction can lead to the loss of some information, which may affect the performance of machine learning models.
2) Choosing the Right Technique: Selecting the appropriate Dimensionality reduction technique depends on the nature of the data and the problem at hand. It requires careful consideration.
3) Interpreting Results: Interpreting the reduced-dimensional data can be challenging, especially in nonlinear techniques like t-SNE.
4) Computational Cost: Some Dimensionality reduction techniques, especially those based on iterative optimization, can be computationally expensive for large datasets.
Conclusion
Dimensionality reduction is a powerful tool that simplifies complex data, making it more manageable and interpretable. By choosing the right technique and understanding its implications, businesses and researchers can unlock hidden insights within their vast datasets.
Earn yourself a promising career in Data Science by enrolling in Data Science Course in Bangalore offered by 360DigiTMG.
Whether you're dealing with images, text, financial data, or any other type of high-dimensional data, Dimensionality reduction techniques can be your secret weapon for uncovering valuable knowledge in the data deluge of the 21st century.
Data Science Placement Success Story
Data Science Training Institutes in Other Locations
Agra, Ahmedabad, Amritsar, Anand, Anantapur, Bangalore, Bhopal, Bhubaneswar, Chengalpattu, Chennai, Cochin, Dehradun, Malaysia, Dombivli, Durgapur, Ernakulam, Erode, Gandhinagar, Ghaziabad, Gorakhpur, Gwalior, Hebbal, Hyderabad, Jabalpur, Jalandhar, Jammu, Jamshedpur, Jodhpur, Khammam, Kolhapur, Kothrud, Ludhiana, Madurai, Meerut, Mohali, Moradabad, Noida, Pimpri, Pondicherry, Pune, Rajkot, Ranchi, Rohtak, Roorkee, Rourkela, Shimla, Shimoga, Siliguri, Srinagar, Thane, Thiruvananthapuram, Tiruchchirappalli, Trichur, Udaipur, Yelahanka, Andhra Pradesh, Anna Nagar, Bhilai, Borivali, Calicut, Chandigarh, Chromepet, Coimbatore, Dilsukhnagar, ECIL, Faridabad, Greater Warangal, Guduvanchery, Guntur, Gurgaon, Guwahati, Hoodi, Indore, Jaipur, Kalaburagi, Kanpur, Kharadi, Kochi, Kolkata, Kompally, Lucknow, Mangalore, Mumbai, Mysore, Nagpur, Nashik, Navi Mumbai, Patna, Porur, Raipur, Salem, Surat, Thoraipakkam, Trichy, Uppal, Vadodara, Varanasi, Vijayawada, Vizag, Tirunelveli, Aurangabad
Data Analyst Courses in Other Locations
ECIL, Jaipur, Pune, Gurgaon, Salem, Surat, Agra, Ahmedabad, Amritsar, Anand, Anantapur, Andhra Pradesh, Anna Nagar, Aurangabad, Bhilai, Bhopal, Bhubaneswar, Borivali, Calicut, Cochin, Chengalpattu , Dehradun, Dombivli, Durgapur, Ernakulam, Erode, Gandhinagar, Ghaziabad, Gorakhpur, Guduvanchery, Gwalior, Hebbal, Hoodi , Indore, Jabalpur, Jaipur, Jalandhar, Jammu, Jamshedpur, Jodhpur, Kanpur, Khammam, Kochi, Kolhapur, Kolkata, Kothrud, Ludhiana, Madurai, Mangalore, Meerut, Mohali, Moradabad, Pimpri, Pondicherry, Porur, Rajkot, Ranchi, Rohtak, Roorkee, Rourkela, Shimla, Shimoga, Siliguri, Srinagar, Thoraipakkam , Tiruchirappalli, Tirunelveli, Trichur, Trichy, Udaipur, Vijayawada, Vizag, Warangal, Chennai, Coimbatore, Delhi, Dilsukhnagar, Hyderabad, Kalyan, Nagpur, Noida, Thane, Thiruvananthapuram, Uppal, Kompally, Bangalore, Chandigarh, Chromepet, Faridabad, Guntur, Guwahati, Kharadi, Lucknow, Mumbai, Mysore, Nashik, Navi Mumbai, Patna, Pune, Raipur, Vadodara, Varanasi, Yelahanka