Sent Successfully.
Home / Blog / Data Science / Statistical Data Science: Essential Knowledge For Every Data Scientist
Statistical Data Science: Essential Knowledge For Every Data Scientist
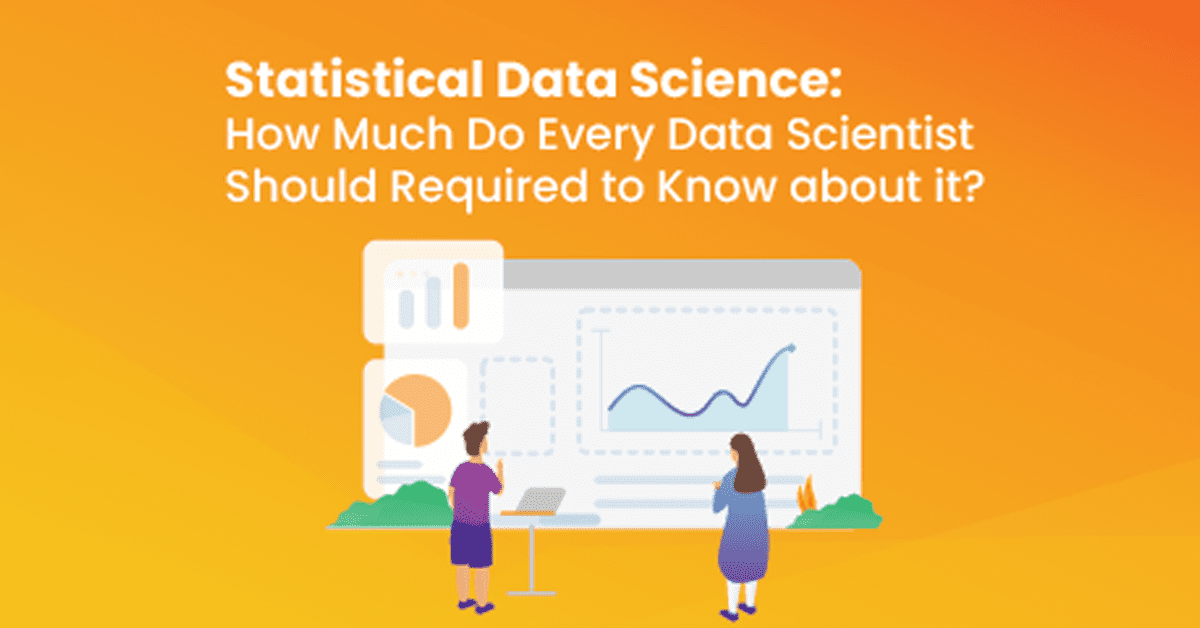
Table of Content
- What is Statistics?
- Statistics' Importance in Data Science:
- Why is Statistics a skill you should have?
- The First Four Basic Steps in Statistical Data Analysis:
- Statistical Data Analysis Techniques Every data scientist Should know about:
- Key Takeaway about Statistics:
- Strengthen your Statistics skills at 360digitMG:
Data science is a multidisciplinary discipline. To have a successful career, a data scientist needs to develop a broad range of abilities encompassing every aspect of the profession. Statistics is one of the building blocks. Even some people refer to machine learning as glorified statistics.
In its broadest definition, statistics refers to a group of instruments and techniques for analyzing, interpreting, visualizing, and coming to conclusions based on data. Statistics is also referred to as the mathematical study of technical data.
360DigiTMG offers the Advanced Data Science & AI in Bangalore to start a career in Data Science. Enroll now!
It is impossible to overstate statistics' role in data science and analytics. Statistics offers tools and techniques to uncover the structure and deliver more in-depth data insights.
Mathematics and statistics both like facts and detest educated guesses. When using the data to solve business challenges and create data-driven decisions, being able to think critically and creatively depends on having a firm grasp of these two crucial concepts.
What is Statistics?
It is possible to respond to significant inquiries about data using a collection of mathematical techniques and instruments called statistics. It is separated into two groups:
Descriptive statistics provide strategies for summarising data by translating raw observations into helpful information that is simple to analyze and communicate.
With the use of inferential statistics, it is possible to analyse experiments with tiny data samples and derive generalisations about the population (entire domain).
Statistics and machine learning are two fields of study that are closely inter-related. Since statistics aid in selecting, evaluating, and interpreting prediction models, it is a crucial precondition for practical machine learning.
Are you looking to become a Data Scientist? Go through 360DigiTMG's PG Diploma in Data Science and Artificial Intelligence!.
Learn the core concepts of Data Science Course video on Youtube:
Statistics' Importance in Data Science:
Most data scientists always spend more time pre-processing data. It necessitates a solid grasp of statistics. A few standard procedures must be followed when processing any data.
- Use multiple statistical tests to determine the significance of attributes.
- To rule out the potential of duplicate features, one must determine the link between the characteristics.
- The transformation of the features into the necessary format.
- Scaling and normalizing the data. Identification of the distribution and type of data is also part of this process.
- Taking the data and doing the necessary data alterations for further processing.
- Determine the appropriate mathematical technique or model after processing the data.
- When the results are attained, they are checked against the various accuracy measuring scales.
The use of statistics is necessary at every single stage of the data processing process, from the beginning to the end. Because of this, a competent statistician can also be a good data scientist.
Want to learn more about data science? Enroll in the Best Data Science courses in Chennai to do so.
Why is Statistics a skill you should have?
Every organization aspires to be data-driven. It explains why the demand for data scientists and analysts is rising so quickly.
Making sense of the data is necessary to address issues, provide answers to inquiries, and lay out a plan. Fortunately, statistics offers a variety of techniques to generate those insights.
From Data to Knowledge:
Raw observations are nothing more than data in isolation. We turn these findings into understandable insights using descriptive statistics. Then, we can investigate tiny data samples using inferential statistics and extrapolate our conclusions to the entire population.
The Definition of Statistical Data Analysis:
Since statistics is a branch of science, it includes data collection, interpretation, and validation. Statistical data analysis is the method of carrying out various statistical operations or in-depth quantitative research that makes an effort to quantify data and uses multiple types of statistical analysis. Here, descriptive data, such as surveys and observational data, are frequently included in quantitative data.
It is a primary method for business intelligence organizations that must work with enormous data volumes in the context of business applications. In the retail industry, for instance, you can use this method to find patterns in semi-structured and unstructured consumer data that you can use to make more robust decisions for improving customer experience and advancing sales. Trend identification is the fundamental goal of statistical data analysis.
Additionally, statistical data analysis has numerous applications in business intelligence (BI), big data analytics, machine learning, deep learning, financial analysis, and economic analysis.
Looking forward to becoming a Data Scientist? Check out the Data Science Course and get certified today.
The First Four Basic Steps in Statistical Data Analysis:
Four fundamental phases are included in statistical data analysis to analyze any problem;
-
Outlining the problem:
A specific and actuarial definition is essential for accurate statistics to be obtained regarding the problem. Data collection becomes incredibly challenging without knowing the precise description or solution to the problem.
-
Gathering the data:
Designing various strategies to gather data is crucial in statistical data analysis after tackling a particular issue. For example, you can collect data from genuine sources or by observation and experimental research investigations that are carried out to gain new data.
An experimental study determines the significant variable by applying the established problem to the study and then controls one or more study components to examine the effects of these components on other variables. An observational study does not conduct trials to influence or control the critical variable. An example of a typical form of observational research is a completed survey.
-
Data evaluation:
The approaches for statistical data analysis are separated into two categories:
Exploratory methods, which use straightforward math and simple graphs or descriptions to describe data, are used to ascertain what the data is showing.
Confirmatory techniques use concepts and ideas from probability theory to attempt to address specific issues.
Because probability is incredibly important in decision-making because it provides a method for predicting, describing, and explaining the possibilities connected with upcoming events.
-
Presenting the outcomes:
By concluding a sample, an estimate or test that purports to represent the traits of a population can be produced; the results may be presented as a table, a graph, or a list of percentages. Only a tiny subset of the data was examined; hence the given result can incorporate probability assertions and intervals of values to reflect certain uncertainties.
Experts might forecast and foresee future aspects of data with the aid of statistical data analysis. A good decision can be made by comprehending and using the information properly.
Statistical Data Analysis Techniques Every data scientist Should know about:
The process of carrying out numerous statistical processes is known as statistical data analysis. It is a quantitative study that uses statistical analysis to quantify the data. Descriptive data, including survey and observational data, are a component of quantitative data. A layperson can only undertake statistical data analysis with statistical knowledge because it often requires some statistical tool. The best statistical data analysis methods are listed below.
-
Linear Regression:
Linear Regression is a process for predicting a target variable by finding the best linear relationship between the dependent and independent variables. Best fit denotes that the gap between each data point's shape and actual observations is as close to zero as is practical. There are primarily two types of linear regression, namely, Straightforward Linear Regression: By offering the best linear correlation, it uses a single independent variable to predict a dependent variable. Click the link to learn more about Simple Linear Regression in depth. Multiple linear regression: This method uses various independent variables to provide the best possible linear relationship for predicting the dependent variable.
-
Classification:
Classification is a data mining approach that grants distinct categories to a data set to make more accurate predictions and analyses. Classification methods include; When the dependent variable is binary or dichotomous, you should use the regression analysis technique known as logistic regression. It is a type of predictive analysis used to explain data and the relationship between a single binary dependent variable and other nominal independent variables. Discriminant Analysis According to computed features, the fresh data set is categorized into one of the known clusters in this analysis, which uses two or more clusters (populations) as the a priori. It clearly shows the distribution of the predictors "X" in each response class. Finally, it applies the Bayes theorem to pitch these classes in terms of estimates for the response class's likelihood given the value of "X."
-
Resampling Methods:
Resampling is a non-parametric method of statistical inference that entails taking repeated samples from actual data samples. Additionally, it generates a novel sample distribution based on the original data and uses experimental methods rather than analytical methods to develop a particular sampling distribution. The following strategies are also necessary to comprehend the resampling procedure; Bootstrapping: You can use the Bootstrapping methodology in these situations for everything from the validation of a predictive model and its performance, ensemble methods, and assessment of bias to the variance of the model. It uses sampling with replacement from the actual data and treats the data points that were "not selected" as test samples. Cross-Validation: By partitioning the training data into K pieces, you can use this strategy to validate the model's performance. The K-1 portion of the cross-validation execution is the training set, and the remainder of the made-out portion serves as the test set. The procedure is repeated up to K times, after which the average of K scores is considered performance estimation.
-
Tree-based Methods:
The most popular approaches for tackling classification and regression issues are tree-based algorithms. As these methods require breaking up predictor space into smaller, more manageable portions, they also require layering or separating it into smaller, more manageable sections. The bagging method reduces the variance of prediction by providing extra data out of the original dataset for training by using "combinations with repetitions" to create multi-step datasets. In reality, increasing the training set size won't increase the model's predictive power; however, doing so will decrease variance, closely adjusting the forecast to an expected outcome. Boosting: This method is used to calculate the result using various models, and the average of the result is then derived using a weighted average method. An adequate prediction efficiency for a long chain of input data by merging the advantages and disadvantages of this approach and using different weighting formulas.
-
Unsupervised Learning:
When the groups or categories present in the data are unknown, unsupervised learning approaches might be used. Common methods (examples) of unsupervised learning include clustering and association rules, which put together distinct sets of data into closely connected categories. Analysis by Principal Components: By identifying a linear collection of the mutually uncorrelated mixture of characteristics with the highest variance, PCA aids in creating low-dimensional illustrations of the dataset. Additionally, it assists in discovering latent interactions between the variables in an unsupervised framework. K-Means Clustering divides data into k clusters based on the distance between each cluster and the centroid. Through the creation of a cluster tree, hierarchical clustering helps create a multilevel hierarchy of clusters.
Also, check this Advance Data Science course in MAlaysia to start a career in Data Science.
Data collection, analysis, and interpretation are all part of the job of statistics as a professional and academic study. In addition, statistics experts must be able to communicate their findings effectively. As a result, statistics is a key tool for data scientists, who are required to collect, evaluate, and present their findings from vast amounts of structured and unstructured data.
Raw information is data. It can be mined, says Data Science Central, and data scientists are taught how to do it. When analyzing data, data scientists look for patterns and trends using a combination of statistical formulas and computer algorithms. Then, they analyze the significance of such patterns and how they apply to actual events using their understanding of social sciences and a certain industry or sector. Value creation for a company or organization is the goal.
To become a data scientist, one need to be very knowledgeable in math, statistics, computer science, and information science. In addition, you must be familiar with statistical principles, fundamental statistical calculations, and how to convey and evaluate statistical findings.
Earn yourself a promising career in data science by enrolling in the Data Science Classes in Pune offered by 360DigiTMG.
Key Takeaway about Statistics:
Both in the workplace and daily life, statistics are crucial. It enables you to evaluate the information provided and make judgments about it.
- Understanding statistics makes reading pie charts, bar graphs, and other types of graphs easier. It also makes it easier to understand data, which, in turn, improves your ability to present data so that others may conclude it as well as you.
- You can quickly identify trends in any data, evaluate the data effectively, and draw better, more accurate conclusions thanks to it.
- Knowing statistics in machine learning (ML) enables you to properly comprehend the efficacy of your models based on the evaluation.
Strengthen your Statistics skills at 360digitMG:
360digiTMG's data science course trains graduates for immediate work prospects and long-term career planning. Although statistical methods and machine learning models will continue to develop, a graduate degree provides a strong foundation that enables students to keep up with technological advancements swiftly. Data science also aims to facilitate collaborative problem-solving. Online courses from 360digiTMG allow students to form enduring bonds with the faculty and their classmates. These networking opportunities can open up internship and career opportunities for students throughout their careers and long-lasting, meaningful relationships.
Looking forward to becoming a Data Scientist? Check out the Professional Course on Data Science and Business Intelligence Course and get certified today.
Data Science Placement Success Story
Data Science Placement Success Story
Data Science Training Institutes in Other Locations
Agra, Ahmedabad, Amritsar, Anand, Anantapur, Bangalore, Bhopal, Bhubaneswar, Chengalpattu, Chennai, Cochin, Dehradun, Malaysia, Dombivli, Durgapur, Ernakulam, Erode, Gandhinagar, Ghaziabad, Gorakhpur, Gwalior, Hebbal, Hyderabad, Jabalpur, Jalandhar, Jammu, Jamshedpur, Jodhpur, Khammam, Kolhapur, Kothrud, Ludhiana, Madurai, Meerut, Mohali, Moradabad, Noida, Pimpri, Pondicherry, Pune, Rajkot, Ranchi, Rohtak, Roorkee, Rourkela, Shimla, Shimoga, Siliguri, Srinagar, Thane, Thiruvananthapuram, Tiruchchirappalli, Trichur, Udaipur, Yelahanka, Andhra Pradesh, Anna Nagar, Bhilai, Borivali, Calicut, Chandigarh, Chromepet, Coimbatore, Dilsukhnagar, ECIL, Faridabad, Greater Warangal, Guduvanchery, Guntur, Gurgaon, Guwahati, Hoodi, Indore, Jaipur, Kalaburagi, Kanpur, Kharadi, Kochi, Kolkata, Kompally, Lucknow, Mangalore, Mumbai, Mysore, Nagpur, Nashik, Navi Mumbai, Patna, Porur, Raipur, Salem, Surat, Thoraipakkam, Trichy, Uppal, Vadodara, Varanasi, Vijayawada, Visakhapatnam, Tirunelveli, Aurangabad
Data Analyst Courses in Other Locations
ECIL, Jaipur, Pune, Gurgaon, Salem, Surat, Agra, Ahmedabad, Amritsar, Anand, Anantapur, Andhra Pradesh, Anna Nagar, Aurangabad, Bhilai, Bhopal, Bhubaneswar, Borivali, Calicut, Cochin, Chengalpattu , Dehradun, Dombivli, Durgapur, Ernakulam, Erode, Gandhinagar, Ghaziabad, Gorakhpur, Guduvanchery, Gwalior, Hebbal, Hoodi , Indore, Jabalpur, Jaipur, Jalandhar, Jammu, Jamshedpur, Jodhpur, Kanpur, Khammam, Kochi, Kolhapur, Kolkata, Kothrud, Ludhiana, Madurai, Mangalore, Meerut, Mohali, Moradabad, Pimpri, Pondicherry, Porur, Rajkot, Ranchi, Rohtak, Roorkee, Rourkela, Shimla, Shimoga, Siliguri, Srinagar, Thoraipakkam , Tiruchirappalli, Tirunelveli, Trichur, Trichy, Udaipur, Vijayawada, Vizag, Warangal, Chennai, Coimbatore, Delhi, Dilsukhnagar, Hyderabad, Kalyan, Nagpur, Noida, Thane, Thiruvananthapuram, Uppal, Kompally, Bangalore, Chandigarh, Chromepet, Faridabad, Guntur, Guwahati, Kharadi, Lucknow, Mumbai, Mysore, Nashik, Navi Mumbai, Patna, Pune, Raipur, Vadodara, Varanasi, Yelahanka
Navigate to Address
360DigiTMG - Data Analytics, Data Science Course Training in Chennai
D.No: C1, No.3, 3rd Floor, State Highway 49A, 330, Rajiv Gandhi Salai, NJK Avenue, Thoraipakkam, Tamil Nadu 600097
1800-212-654-321